5 Q’s for Cornelius Fritz, Statistician at the University of Munich about Facebook’s Data for Good Program – Center for Data Innovation
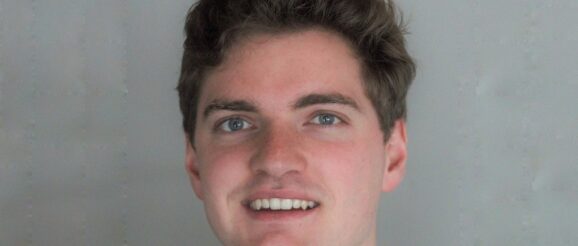
The Center for Data Innovation spoke with Cornelius Fritz, a statistician at the University of Munich in Germany. Fritz discussed how he is using mobility data from Facebook’s Data for Good program to investigate the impact of social connectedness on the spread of COVID-19.
Hodan Omaar: How have you been using Facebook’s data on human mobility?
Cornelius Fritz: In a study we published last month, we were using Facebook data to analyze how regional differences in mobility and social connectedness affect the spread of COVID-19 in Germany. Many studies on mobility during the pandemic have focused on the impact that restrictions have had on national infection rates or the economy. Our work has a different focus and is complementary to these efforts. We’re using mobility data to understand how mobility patterns and friendship ties affect the spread of COVID-19 at a local level.
To do this, we used data from approximately 10 million Facebook users, who enabled geolocation features on their phones, and aggregated this data to the 401 federal administrative districts in Germany. With this data, we were able to construct a district-level model for meeting patterns, where highly concentrated meeting patterns indicate that people are only meeting up with people from their own or nearby districts, and lowly concentrated meeting patterns translate to more dispersed meeting patterns.
We also included a social connectedness index to measure the strength of friendship ties between the districts of Germany based on an anonymized snapshot of active Facebook users and their friendship networks. We included this because friendship ties are much more influential in long-distance mobility than they are in short-range mobility, so it is crucial to understand the impact social network links have on movement to understand variations in infection rates for COVID-19.
Omaar: You included a really interesting graph that shows a distinction between the former East and West Germany. What’s going on there?
Fritz: Yes, we found a fascinating result when we visualized friendship ties. We constructed a graph that plots friendship coordinates, which capture characteristics from the social connectedness index and measure the connectivity within federal states and neighbouring districts. The graph shows that there are still divisions between East and West Germany when it comes to social ties even more than 30 years after reunification. On one side, there are social ties among western districts, and on the other side there are social ties among eastern districts. There is largely a blank space in the middle, except for Berlin, which makes sense as it is the capital and the most central city that connects the two sides.
In fact, there are distinctions in predicted infection rates as well. Our model suggests that the number of infections in a district would likely be lower than the national average if that district was located in the former East Germany.
Omaar: Who is the end user of the insights from your project? What decisions do you anticipate they will make with your work?
Fritz: There are many ways people can use these models, but perhaps one of the most useful would be for policymakers to evaluate district-level policies. In particular, our results corroborate that interventions limiting trans-district movements are useful and show that concentrating meeting patterns through local lockdowns could mitigate further national outbreaks. But policymakers can also use this type of model as a predictive tool to better manage healthcare resources, such as hospital beds, respirators, and vaccines.
Omaar: What types of data would you need for policymakers to be able to use this type of model for COVID-19 forecasting?
Fritz: For policymakers to be able to properly identify which containment strategy will be most successful, they need a robust and interpretable forecast of infection rates. Machine learning models such as graph neural networks are a great way to do this, but the most promising of these models are those that use nuanced data on behavioral patterns.
In a study we published in January, we improve upon existing forecasting efforts with graph neural networks by including three different types of data. The first type of data that we incorporate are colocation maps, which indicate the probability that two people from different districts will meet up during a given week. Second, we use the social connectedness index to quantify social connections between districts. Finally, we used geolocation data to identify the percentage of people who were staying put, defined as those who stay within a 0.6km radius throughout a day. Using our model, we get consistently smaller errors than benchmark models to forecast COVID-19 infection rates one week into the future.
Omaar: Could you have done your work without Facebook’s data?
Fritz: We would have struggled to do our work without Facebook’s data because it is at such a granular level, which is what we are looking at. It is becoming increasingly important to do work with mobility data and while many companies collect this data, it is still not readily available. Many times it just sleeps on servers somewhere. It is really commendable that Facebook has made this data available to researchers, and it would be good to see other companies do the same.