5 Q’s for Wim Kees Janssen, CEO & Co-Founder of Syntho – Center for Data Innovation
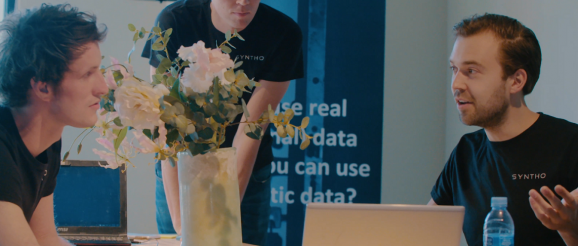
The Center for Data Innovation spoke with Wim Kees Janssen, CEO & co-founder of Syntho, an Amsterdam-based company expert in synthetic data generation and implementation. Janssen discussed how organizations can unlock the power of their real data by using artificial intelligence (AI)-generated synthetic data.
Christophe Carugati: What challenges does synthetic data aim to address?
Wim Kees Janssen: As most readers know, technologies like AI, machine learning, and business intelligence need data. However, in most organizations, getting access to data is challenging due to time-consuming and demoralizing processes that will cause those organizations to innovate in a suboptimal manner. Think about it, do you remember the risk assessments, legal contracts, and endless discussions with your colleagues or third parties to get access to relevant data?
Key question: why is this the case? Because original—real data—typically contains privacy-sensitive elements and is therefore restricted by regulations, such as the General Data Protection Regulation (GDPR). When realizing the potential value of the aforementioned buzzwords (AI, ML, BI) that stands high on the agenda of many organizations, a strong data foundation to get easy and fast access to high-quality data to realize them is crucial.
Our solution is to use AI-generated synthetic data as an alternative to using the original sensitive data. Thereby, we preserve the data quality and eliminate the risks and hence, all aforementioned data access challenges.
Carugati: What is synthetic data, and how does it differ from real data?
Janssen: Synthetic data is generated by an AI algorithm that creates completely new and artificial data points. This new dataset has no elements from the original data and therefore does not count as personal data.
A key difference is that we model those new data points with AI to preserve the statistics, patterns, and relationships on a dataset or even database level. This is what we call a synthetic data twin. With our advanced models, we are able to preserve this data quality to such an extent that conclusions drawn on the synthetic data will be the same as conclusions you could draw from the original data. As an expert and specialist in high-quality synthetic data generation and implementation, we focus on complex datasets, large databases with multiple tables, and complex data structures. With our maximized data quality, this even holds for the most complex analytics models, such as AI, machine learning, and business intelligence models. In other words, you will have all the pros and none of the cons when using synthetic data as an alternative to using original data.
Next to generating a synthetic data twin, synthetic data has even more to offer. By applying generative-AI, you can influence several parameters to optimize your data. For example, if your original dataset or subset contains 5,000 data points, we can easily create a synthetic dataset with 100,000 or even more data points. Another example is that we can remove biases. So if your original dataset consists of 70 percent females and 30 percent males, we can generate extra male or female data points to balance the dataset to 50/50, simulating a bias-free situation. We support many more of these value-adding synthetic data features that allow our clients to take their data to the next level.
Carugati: What are some of the most interesting ways organizations are using synthetic data today?
Janssen: There are many possible use cases.
One key use case is data for modeling. Many organizations develop models and dashboards—for example, with artificial intelligence, machine learning, and business intelligence—for internal use or their clients. One would like, for instance, a forecast of which persons one should target the new advertisement campaign based on various demographics, such as age, gender, status, purchase behavior, etc. Typically this data is sensitive and cannot simply be accessed and shared internally, or maybe even externally. Getting data access will take you time and energy, and classic anonymization techniques will destroy your data to do proper analysis, resulting in the garbage-in equals garbage-out principle. Here, when developing these models, having easy and fast access to high-quality data is of the essence. Syntho generates synthetic data on which you can develop those models as an alternative to using the original sensitive data.
Another use case that we focus on is test data for software development. When developing software, companies require test infrastructure to develop and test their applications. In such an infrastructure, high-quality test data is of the essence because bad quality test data results in bad opportunities to test and develop properly. As an alternative to using production data or classic anonymization techniques such as fake, randomly created dummy data or scrambled data, we arrange your test and development infrastructure with AI-generated synthetic data to eliminate risks while having high-quality data easily and fast accessible. As mentioned before, here we offer additional value-adding synthetic data features to take your test and development infrastructure to the next level with optimized data for testing purposes. Think about data that does not exist yet or edge cases that you want to have more frequently present in your test dataset.
Here, it is an option to train the model later on the original data. The benefit is that instead of bringing the original data to your model and facing all aforementioned challenges, you can develop the model on synthetic data and take your model to the original data.
Carugati: How do you ensure the quality of synthetic data? And what are the limitations of synthetic data?
Janssen: To ensure the quality of synthetic data, we provide a quality report for every generated dataset. This report shows various statistics where the original data is compared with the generated synthetic data. Naturally, the client is also encouraged to analyze statistical differences or similarities and create their own quality report. Furthermore, we work with an external expert who regularly assesses our data quality and provides our clients with these results as a reference. The data experts from SAS, the market leader in data analytics, recently assessed our data quality and will share the results with the broader public via a webinar and our data quality page.
The essence of synthetic data is that it does not contain any personal data. There is no one-on-one relationship with the original data anymore, meaning it is impossible to go back from the synthetic data to the original data. Consequently, where synthetic data is very useful for analytical tasks and purposes, it is not that useful for operational ones. For example, if you synthesize a dataset of clients and then send invoices to those synthetic clients and addresses, I can promise you that you will not receive one penny.
Carugati: How do you expect the use of synthetic data will change in the future?
Janssen: The possibilities of synthetic data are endless. For instance, Gartner recently predicted that by 2024, 60 percent of all training data for AI would be synthetically generated. Now, in 2021, we see that many organizations have not heard about synthetic data at all, that organizations struggle to define value-adding use cases or to form a concrete starting point. In conclusion, the potential is huge, but their journey toward realizing that 60 percent forecast is also huge.
We are here to boost the adoption of synthetic data and to solve synthetic data challenges. Syntho helps organizations to form concrete starting points, to define possible use cases, or to take the adoption of synthetic data generation to the next level. Our team consists of real persons (not synthetic persons), and we would love to get in touch with anyone who would like to explore the value of synthetic data with us.