A Systems Approach To Innovation – From Guessing Correctly, To Learning Quickly
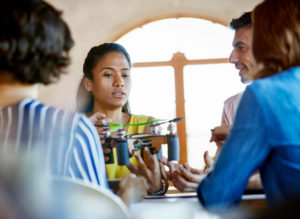
When we think of innovation, many of us envision a team of experts conducting projects in a systematic manner – following defined steps to track progress, determine outcomes, and plan next steps to design a new technology.
This lends itself very well to very well-defined problems. Typically, the team follows the engineering method, moving from idea, to concept, planning, design, development, and implementation to solve these problems. However, issues often arise when “things don’t go according to plan!” – when the outcome isn’t correct or foreseen – and lead to a project stalling or failing altogether.
In the case of undefined or very complex problems, as is almost always the case in business or social issues, this linear approach presents several critical issues. In these domains, most opportunities and challenges emerge from the behavior of a system over time, be it economic, social, physical, or technological.
A system, at its most basic, is the interaction of various discrete components or parts. It’s easy enough to visualize and consider each of these parts in isolation; however, we almost always underestimate the difficulty in predicting the outcomes and behavior of the system during the design stage.
For example, in the case of plastics pollution, key material technology developments in plastics allowed society to address economic issues, such as food availability (by increasing shelf life) and reducing the need for sterilization of certain medical equipment (due to plastic health consumables). However, plastic became too much of a good thing, with, for example, UV-stabilized plastic being largely impervious to breaking down in the natural environment.
Another sustainability initiative aimed to address worsening pollution issues by limiting the use of cars on certain days. Cars with license plates ending in even digits could be driven on certain days of the week, and those ending in odd numbers could be driven on the other days. Since people still needed to commute, they started buying old, second-hand vehicles (typically with worse carbon and pollution emissions) with different license plate numbers to use on the alternate days of the week – increasing, rather than decreasing, pollution.
While the engineering and business fields have developed several methods (including root-cause analysis, scenario planning, etc.) to try to understand and address these emerging issues, they still rely heavily on teams calculating or forecasting outcomes of systems. However, even systems with as few as three elements create outcomes that cannot be calculated or predicted. In some cases, existing technology solutions can reduce execution risk in many innovation initiatives.
Typical problem-solving techniques, however, require that we correctly guess the outcome of a system’s design or change. From an innovation standpoint, it is more effective to borrow from science and conduct experiments on or within the system to validate ideas cheaply and quickly (learn fast!) to determine what the true outcomes will be.
The mantra of “fail fast” is well known in the innovation community; however, the focus is often on rationalizing failure, even though it conveys that gaining knowledge through quick (ideally cheap!) tests can lead to more informed stakeholders and eventually successful outcomes.
Many areas of engineering, particularly in the software field, look at addressing problems in an empirical manner using techniques such as agile and test-driven development. These development teams don’t need to correctly guess the outcome of a certain stage to plan an entire project from start to finish; rather they can quickly learn the outcome, which will inform their R&D work in an iterative manner.
When considering how to approach different problems, key systems-thinking concepts need to be applied – considering that the impact of feedback, human psychology, and system changes can lead to counterintuitive solutions that address a challenge more comprehensively.
A classic example is the now pervasive “Just-In-Time” manufacturing method. When first proposed in the 1980s, business experts questioned this new method as being incredibly counterintuitive. It created a major risk that component stockouts would bring operations to a stand-still since inventory on hand was kept to a minimum. This new methodology, however, reduced cash tied up in inventory, contributing to better business performance.
It also allowed businesses to become increasingly agile in meeting ever-changing customer demand by allowing product customization, and it increased product ranges, as manufacturers weren’t limited to only what they had stockpiled. Using information technology to further reduce the stock-out risk, the Just-In-Time method has revolutionized manufacturing.
SAP Innovation Services helps companies navigate, understand, and address their innovation opportunities and challenges with methodologies to ensure ideas are rapidly validated throughout development, and ultimately able to be delivered at scale. Find out more at SAP Innovation Services.