Deep learning helps group study contagion pattern of COVID-19 – Med-Tech Innovation | Latest news for the medical device industry
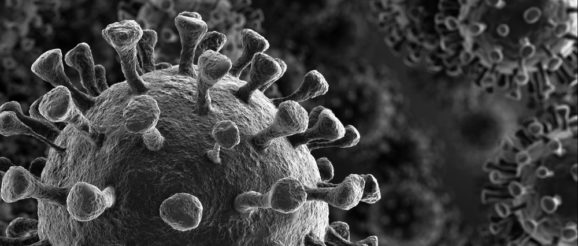
U.S. Consumer Healthcare Advocacy Group (USCHAG), a company that uses deep learning algorithms to advocate on behalf of consumers within the health and wellness space, has started to study the contagion pattern of COVID-19.
It has discovered, by collecting and releasing collated data related to the path COVID-19 has taken, the ability to track and predict the spread of the virus, in real time via an open source platform, which could be instrumental in containment and future outbreak control.
While the epidemiology of the disease has already identified the genome responsible for the virus, the patterns by which the virus spreads are still wholly unknown. This is where open source data collection and release can step in and contribute to research and development of solutions, faster than ever before.
USCHAG CEO, Scott George said: “Lack of information is often the catalyst for mass panic when it comes to fast spreading disease. We see some very applicable ways to help contain and educate through data that can be collated on the quantum level, that when released, has the ability to definitively slow or even stop the spread of these super bugs and viruses before they reach pandemic proportions.”
USCHAG uses technology, mostly based in Artificial Intelligence and accelerated by quantum computing to solve healthcare issues. By using open source data via sources like Nextstrain.org it can guide and expediate research techniques prior to the epidemic growth. Open source data is the key to quickly assessing epidemic solutions and with other health issues which allows doctors and scientists to create plans and procedures, before the crisis can get out of control.
George added: “Imagine the application of open source data to eradicate complex public health issues currently known to mankind, quickly, due to the massive amounts of data being shared in real time utilised by some of the smartest minds in science. This is in stark contrast to how data is collected and correlated now. Moving to an open source platform of data sharing – flies in the face of conventional scientific findings, which currently includes peer review and curation of data in a more analog system.”