NY’s Bill on Automated Hiring Will Dampen Its Recovery Efforts – Center for Data Innovation
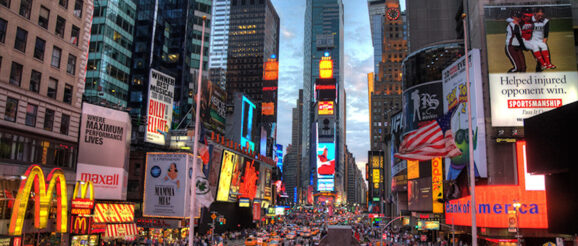
Reducing unemployment and bolstering the COVID-impacted economy should be the top economic priority for policymakers. Despite a 6.7 percent unemployment rate and increased teleworking widening the talent pool, four in ten American employers are still struggling to find workers with the right skills.
Fortunately, new technologies are poised to help millions of people get back to work faster by automating the hiring process, helping companies better identify and advance the best job applicants. But in New York City, where the unemployment rate is almost twice the national rate, brewing legislation threatens to slow recovery down.
The New York City Council has proposed a bill that would make it unlawful for companies to sell automated hiring tools that have not been audited for bias in the year prior to sale, and every sale would have to include a free annual bias audit service that provides the results to the purchaser. Unfortunately, this bill falls short by misguidedly framing discrimination in employment as a technical problem rather than a complex social one, and offers solutions that would do little to address discriminatory bias and do even less to help people get back to work.
Automated hiring tools can be used at several stages of the hiring process. Consider, for example, the task of reviewing resumes. Employers can use resume screening tools like those Ideal offers, to sift through large volumes of candidates for those that meet their basic qualification requirements. Checking references can be streamlined by companies such as Checkster that push standardized questions to those acting as references, aggregate the feedback, and analyze the responses. Bringing candidates in for interviews can also be eliminated with tools such as HireVue, which Goldman Sachs and JPMorgan, among others, are using to conduct standardized, video interviews that are scored against an assessment model tailored to an employer’s needs.
One problem with the bill is that it unfairly applies rules to a narrow set of these systems rather than all automated hiring systems. Automated hiring systems are defined in the bill as only those that are “governed by statistical theory” or those whose parameters include “inferential methodologies, linear regression, neural networks, decision trees, random forests, and other learning algorithms.” But applicant tracking systems—which employers have been using since the 1990s—that use less complex algorithms to aggregate and sort job applicants into databases that can be filtered based on pre-set criteria, would be excluded. Yet these systems can cause as much harm, if not more, than the more modern automated systems that use AI to better match the best candidate to a job opening. As it is written now, the bill only serves to stigmatize and discourage AI use, hindering companies from using AI to identify the best person for the job.
Another problem is the bill minimizes how technically challenging it is to audit for bias. Vendors can certainly attest that they have not designed their systems to intentionally discriminate against candidates based on protected characteristics, but it is much harder for them to validate that there is no bias with a particular employer or job listing. For example, an employer might indicate a preference for graduates from a particular set of universities, which may skew the demographics of hired candidates. Or a job listing may include unnecessary qualifications that tend to exclude other groups of candidates. Moreover, for vendors to test that their systems do not exclude candidates based on what the algorithms identify as their nationality, gender, race, age, or sexuality, they would need data from employers about the legally protected classes to which applicants and employees belong, information which many employers do not collect. Even if they do collect this information, responses to these types of questions are always voluntary, and so there will always be gaps in the data which might lead to mistaken conclusions about bias, especially on small datasets.
More importantly, just focusing on potential biased outcomes from an algorithmic tool ignores the bigger picture. Yes, in some settings algorithmic systems could run the risk of amplifying these biases. But these systems could also reduce biases and help employers address their own hiring biases. To be more impactful, policymakers should be addressing the root of the problem: that some employers exhibit biases in their hiring decisions. Focusing legislation on the vendors who sell one type of tool rather than employers who are involved in the hiring decisions themselves will do little to address the bigger problem.
Indeed, unless employers with biased hiring practices make changes, even seemingly debiased ranking algorithms will not eliminate this problem. For example, a 2020 study found that employers hiring for event staffing were always more likely to hire women, even when they used a ranking algorithm that swapped the genders of candidates from male to female and vice versa.
Focusing anti-discrimination rules on employers who use automated systems would be a more efficient way of encouraging vendors to develop responsible hiring systems because doing so would send a market signal to developers about what customers will expect of an algorithmic system. This will encourage developers to provide algorithms with the necessary capabilities, through mechanisms such as transparency, explainability, confidence measures, and procedural regularity, or risk losing market share to competitors that do.
Ultimately, policymakers in New York City should be doing everything they can to address the fact that they have one of the highest unemployment rates in the country. That means helping companies get more people back to work quickly and fairly. This bill is not going to help do either.