5 Q’s for Dr Ilya Feige, Director of AI at Faculty – Center for Data Innovation
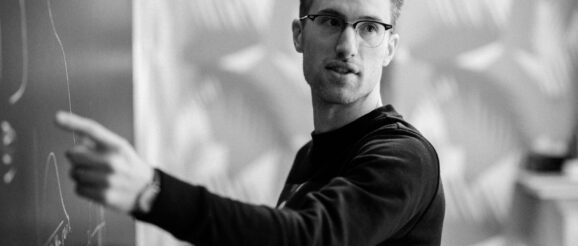
The Center for Data Innovation spoke with Dr. Ilya Feige, director of AI at Faculty, applied AI/machine learning company that provides a combination of strategy, software and skills for organizations. Dr. Feige talked about the differences between developing AI compared to traditional software engineering, Faculty’s approaches toward building safe, reliable, and explainable AI products, and the rise of causal inference in AI.
Ben Mueller: How does Faculty use AI to add value to its clients? Do you supply software products, bespoke consulting services, or a mixture of both?
Ilya Feige: To get the most value from AI, organizations need a hybrid approach that combines both deep expertise and best-in-class technology. Faculty offers a combination of the two. We build, deploy, and maintain AI that solves the most important problems for our customers. Our AI software draws on breakthroughs from years of research as well as expertise and approaches from our team which includes over 50 PhDs and consolidated experience deploying AI in over 350 real-world projects.
To give some color through examples, our AI software is helping the National Health Service (NHS) to forecast the demand for vital services during the COVID-19 pandemic and helping a U.S. retailer to optimize marketing spend and save millions by sending catalogues to the customers whose purchasing behavior is most likely to be changed.
Mueller: How does product development in AI differ from traditional software engineering?
Feige: AI often fails because it’s treated like traditional software. Unlike software, AI uses real-time data and that means it will inevitably degrade over time. In traditional software, you write your code and input your instructions and you get the outcomes. However, in AI software, you input your data and your objective, and you essentially get the code. This is because when you are training an AI system, you’re actually picking up on correlations that are inputted directly from the outside world through the data. AI does not necessarily tell you about the real (causal) structure of the world, instead, it looks at what is correlated, and it uses that to make predictions.
Furthermore, organizations that buy off-the-shelf AI software often struggle to integrate it into their business to create value, because they haven’t built it deeply into the processes and workflows that make up the operations of a business. That’s why you need a level of customization of an AI software product in order to get the best value from it.
AI systems need to work for organizations; they need to be understood and trusted by, and convenient for, their users. When it comes to AI products, it’s therefore important that the tool is built for the user and that you understand the business problem it’s trying to solve. The results need to be presented to end-users in an understandable and explainable way, allowing them to take the right action and be able to trust the recommendations it gives. An organization isn’t going to see widespread AI adoption if everyday operational users can’t see how or why the model is making decisions.
An example where we’ve overcome this challenge is in our recent work with the NHS to build the Early Warning System. This is a first-of-its-kind AI toolkit that forecasts vital metrics such as COVID-19 hospital admissions and required bed capacity using a range of different data sources. We have built-in AI safety and model validation analysis to support operational decision-makers. This is helping users to understand and interpret how each input, such as past hospital admissions or local testing data, influences the outcome of the forecast. For example, users can see how much recent historical admissions data for a particular trust is driving that trust’s forecast, versus how much local testing data is influencing it. This has been instrumental in driving more informed decisions, while also increasing trust in and adoption of the tool, which now has over 1,000 users.
Mueller: What is Faculty’s approach to AI safety and reliability?
Feige: AI safety considerations are at the heart of Faculty and the way we work and develop AI. If you want to make AI useful in the world, it has to be both safe and high-performing. We’re one of the few commercial companies with a dedicated research program into AI safety, and we regularly publish scientific papers that advance the field of machine learning and safety. This research is informed by our real-world experience using AI and our team of PhDs, and is built into our safety tools on our delivery platform allowing us to deploy and scale safe AI for our customers.
At Faculty, we focus on four main pillars of AI safety for ethical AI deployment: fairness (does the model have implicit biases that could skew its outputs?), privacy (does the model protect sensitive information?), robustness (does the model cope well with new data and errors?), and explainability (can you understand what the AI is doing and how it makes its decisions?).
Mueller: What is Faculty’s view on how to build trustworthy AI applications? Are demands for explainable AI technically sound?
Feige: The general public is increasingly aware of how businesses, public sector organizations, and government departments are using their data. This combined with falling trust in tech giants has rightly increased scrutiny of how our data is being used and who is using it.
Organizations on the other hand have been presented with a false choice: that you have to sacrifice the performance of AI models if you want to make them safe. In our experience, that’s just not the case. With the right techniques and tooling, you can have both, just like cars are built to be both fast and safe.
At Faculty, we have put a huge emphasis on the practical challenges around what “explainability” is, especially on what form of explanation is appropriate in different situations. For example, how the causality behind an action should affect its explanation. Many popular off-the-shelf tools use open-source packages like SHAP (which assumes feature independence) and LIME (which assumes both local linearity and feature independence). These have been proven to give inaccurate explanations because they tend to miss interactions between features and can lead to wrong or misleading conclusions about a model. In our view, a wrong explanation is worse than no explanation at all, especially if organizations are going to act on it to make critical business decisions.
AI safety tooling is needed to help organizations work within the law. However, while many AI principles have been published attempting to address safety issues, very few governments or companies have issued technical and practical solutions to this problem and their principles don’t tend to recognize the broad range of contexts in which AI can be applied. It’s our view that the next wave of regulations need to be informed by a practical and technical understanding of how organizations use AI day-to-day.
For example, we recently completed a piece of work with the Centre for Data Ethics and Innovation that looked at the implementation of fairness tooling and developing technical standards aimed at practitioners. This included a clear structure for considering different mathematical definitions of algorithmic bias and a clear range of approaches that practitioners can use to detect and mitigate that bias throughout development and deployment. We hope that collaboration between governments and data scientists on the ground, will lead to more practical guidance on how to build and deploy AI safely in a real-world setting.
Mueller: What developments in AI do you view as particularly promising for the coming decade?
Feige: We are seeing the beginning of the merging of the discipline of causal inference with that of AI. Causal inference is a discipline of statistics that seeks to learn the actual underlying causal relationships from data, rather than just learning the correlations. The potential of causal inference is highly compelling: If you knew the actual causal relationships between all the variables in your data, you’d be able to make predictions in scenarios that you’ve never seen before, making models robust and more powerful for scenario planning.
Developments over the coming decade are going to move the needle on causal AI. At Faculty, we have already been developing causal AI systems for our customers and are seeing more appetite for this as the use of AI becomes more widespread. A good example of the application is in marketing, where it is very easy to be (literally) confounded by the fact that customers who buy a lot look like really great targets for marketing, even when they would have bought anyway without the marketing touch or the promotion. Organizations who ignore the causation tend to give away their margins, and even worse, degrade their brands, for an upside that is largely not there. By adopting casual marketing methods with AI, it’s possible to target your marketing spend at what provides the most incremental impact on your sales.