Keys to Ensure that Data isn’t Slowing Down your Innovation Efforts – Cloudera Blog
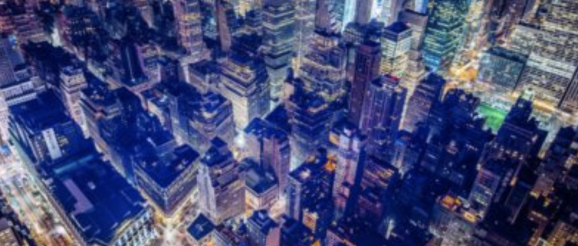
Data Lifecycle Management: The Key to AI-Driven Innovation
In digital transformation projects, it’s easy to imagine the benefits of cloud, hybrid, artificial intelligence (AI), and machine learning (ML) models. The hard part is to turn aspiration into reality by creating an organization that is truly data-driven.
ML models powering AI use cases are becoming more and more ubiquitous in a variety of environments, especially at industrial organizations adopting Industry 4.0 technologies. For those models to produce meaningful outcomes, organizations need a well-defined data lifecycle management process that addresses the complexities of capturing, analyzing, and acting on data. Otherwise, they risk quickly becoming overwhelmed by massive volumes of data captured in different formats from a diversity of sources, including Internet of Things (IoT) sensors, websites, mobile devices, cloud infrastructures, and partner networks.
To prevent that, companies must implement a strategy to make sense of data by first training AI algorithms and then continually refining them as new, relevant information becomes available. That way, the data can continue generating actionable insights.
“Before training can even begin, the hard problem is collecting the labeled data that is crucial for training an accurate AI model,” said Joshua Robinson, a founding engineer of Pure Storage’s FlashBlade. “Then, a full scale AI deployment must continuously collect, clean, transform, label, and store larger amounts of data.”
Rethinking the Data Lifecycle
In modern hybrid environments, data traverses clouds, on-premise infrastructure and IoT networks, so the process can get very complex. It requires rethinking the data lifecycle itself.
Data processed at the edge or in the cloud, for instance, is not effective if it follows the traditional lifecycle of “ingest, process, land, and analyze.” If the data goes into a data lake before analysis, extracting it can get pretty complex and time-consuming. It makes more sense to analyze and derive insights from it, and then place it in the data lake — properly tagged for easy access later.
Data source diversity also must be addressed because it, too, adds complexity. It’s important to implement an integrated platform that provides an accurate picture of an organization’s end-to-end operations, said Dr. Florian Baumann, CTO for automotive and AI at Dell EMC, during a recent Cloudera digital event, Industry 4.0 – Made Real.
Such a platform enables an organization to curate different types of data from diverse sources and identify which data to feed to ML algorithms to generate meaningful insights, he said. Data from sensors on factory equipment, for instance, delivers measurements on machine vibrations and ambient temperatures, which can be used for predictive maintenance to improve efficiencies and control costs.
Improving Patient Care
Improving the speed of the data lifecycle can have a measurable impact — and not just on the bottom line. In healthcare, organizations are using data to improve Patient Care.
At Rush University Medical Center in Chicago, the process of turning data from various sources into actionable insights is no longer just an aspiration. In its efforts to truly become “data-driven,” the hospital is using ML, predictive analytics, and other forms of AI for real-time analysis of various types of data, including information about patients’ genomic makeup and doctors’ notes, to improve medical care.
Rush needed a data platform to provide deep insights fast for patient care. “We don’t believe that we can do all the projects in our pipeline without a big data platform that can support new types of data, along with the growing volume and high velocity of data we want to analyze, ” said Jawad Khan, director of data science and knowledge management at Rush.
Using Cloudera’s enterprise data platform and technologies such as Apache Spark, the medical center streams data in real time and analyzes it in near real time to derive insights that have a real impact on patient care.
“Traditionally, we were waiting 24 to 48 hours for patient data to be able to collect the data and to do analysis on it,” Khan said. “The data helps us understand the specific gene makeup of a given patient, and understand what care path is best suitable for that patient.”
“The addition of doctor’s notes to the ML models,” says Dr. Bala Hota, Rush chief analytics officer, “doubled the accuracy of the models to provide best care and seek best outcomes.”
Rush’ transformation into a healthcare innovator through data and AI applications has received a seal of approval from the industry, when in June 2021, Newsweek ranked the medical center among the World’s Best Smart Hospitals.
Avoiding Complexity
Rush’s use of AI illustrates the importance of well-defined, properly executed lifecycle management. A keen focus on reducing complexity and delivering meaningful outcomes can truly make a difference in creating a data-driven organization. Companies that get it right don’t just aspire to a successful digital future; they make it happen.
Download The Definitive Guide to the Machine Learning Lifecycle now to learn how to take control of the ML lifecycle—so you can build and scale practical AI use cases to solve your actual business problems.