Divergent thinking and true AI innovation – DataScienceCentral.com
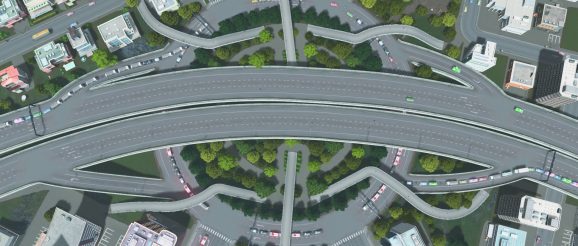
Research and Markets estimated that annual global sales of information technology reached nearly $8.4 trillion in 2021. At that level, IT sales made up just less than 9% total estimated global annual gross domestic product (GDP).
Global IT sales tend to grow about 6.6 percent annually. For the sake of argument, let’s assume that the annual IT sales growth averages 6.6 percent from 2022 through 2030. This assumption includes global GDP growth for the period averaging just over 3.0 percent annually.
Back in 2018, PwC’s economists in the UK predicted the following:
AI could contribute up to $15.7 trillion to the global economy in 2030, more than the current output of China and India combined. Of this, $6.6 trillion is likely to come from increased productivity and $9.1 trillion is likely to come from consumption-side effects.
In short, the economists’ best-case scenario is that the global economy will take effective and efficient advantage of the “AI” that’s becoming available, year after year, country by country.
Here are five reasons why I think AI is highly unlikely to reach this level of potential global economic impact by 2030:
Imagining the future with divergent thinking
One of the things that seem to be missing in conversations about how to best take advantage of “AI” is divergent thinking. A former boss of mine had a background in Clinical Psychology and had worked as a researcher at Stanford Research Institute (SRI).
One of the first things the boss (who had a lot of autonomy) did as the head of our newly formed group was to divide the research part of each project into two phases–divergent and convergent research. The divergent phase, the first phase of research, was purely exploratory. We intended to turn over all sorts of rocks and discover first, without preconceptions. Then the convergent phase would focus on what seemed to be the most fruitful path identified.
Let me give you an example of how effective this approach could be. A few years after our group was formed in the mid-2000s, we planned a project to uncover what was happening that was truly new and different in the area of business intelligence (BI). We were looking for fresh insights.
A divergent thinking example: BI
Consider first how IT market research has historically been done. If they were researching BI, many mainstream market research firms would look at the existing installed base of business intelligence software, find out what the incumbent providers had on their product roadmaps, size up the installed base, review new competition, and compare what the competitors were doing with what the incumbents planned.
Then do a forecast. In other words, the mainstream research firms assumed that mainstream BI software was already on the most fruitful path, to begin with. Implicit in that assumption is a clear bias toward incremental change–the path is more or less linear, and so the past is a good predictor of the future.
What did we do differently? First, we tried to ask better questions and do root cause analysis. What’s the biggest problem in business intelligence, we wondered? One of our early exploratory interviews was with Doug Lenat of Cycorp. Doug described a big problem with BI as the “drunk person looking for his keys under the lamppost” problem.
As the story goes, the drunk is out in the snow at night, on his knees near a lamppost, looking for his keys. Some people come by and ask him what he’s doing, and he tells them. “Where did you lose your keys?” someone asks him. “Over there,” the drunk says, pointing to an area well removed from where he’s looking. “So why are you looking here?” someone else asks. “Because this is where the light is,” the drunk responds.
For decades, BI software providers have only focused on tabular kinds of structured data. Data in that form is difficult to integrate and contextualize–note the problems with data warehousing and how most data warehousing has failed.
But Lenat has been a major advocate of semantic data integration–bringing many data sources heterogeneous sources together as an integrated, queryable knowledge base–for decades. From a business intelligence perspective, the Cycorp approach would, in theory, be more likely useful for root cause analysis and more relevant question answering generally.
For that reason, we decided to focus our convergent research on semantic web techniques from a BI context.
Takeaway: Think twice about following the herd
13 years later, I still think the research we did back then was directionally correct. In fact, the idea of knowledge graphs is a popular one. But what’s frustrating now is that so many organizations are, even so, still mentally back in the 2000s, because awareness is so low about alternatives to data integration, management, and building a better foundation for business intelligence. Those who have the budget and those who advise them often misdirect where the AI investment goes.
What should organizations have done differently, all those years ago? Learn from the divergent thinkers like Lenat and how foundational technologies such as knowledge representation (KR) could provide the foundation for machine learning and advanced analytics.