Decisions Part 2: Creating a Culture of Analytics-driven Innovation – DataScienceCentral.com
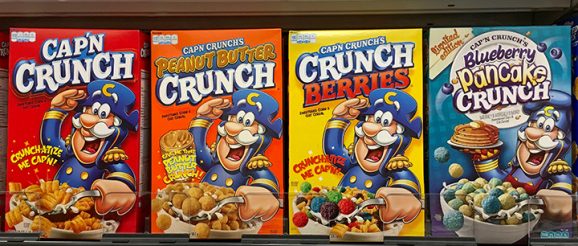
In part 1 of this multi-part series on the value-realizing, collaborative power of decisions, we introduced the concept of an AI-driven decision factory. An AI-power decision factory leverages an AI-enabled and human-empowered culture to continuously explore, learn, adapt, unlearn, and re-learn at the customer and operational frontlines of the organization. An AI-powered decision factory facilitates scaling value creation by continuously optimizing and refining the decisions that drive business and operational outcomes (quantifiable, measurable) in a world of constant disruption and transformation.
And leveraging the Value Engineering Framework (Figure 1) for understanding, defining, and operationalizing how organizations create value, and how they measure the effectiveness of the value creation processes, is the starting point for your AI-powered decision factory.
Figure 1: Value Engineering Framework
Defining how the organization creates and measures value should be the easy part. I mean, why does senior management exist if not to clearly define, communicate, and align the organization around how the organization creates and measures value. Unfortunately, many organizations struggle to clearly articulate and drive consensus about how they create value and measure value creation effectiveness. I can’t tell you how many times I’ve asked a client “what are the KPIs and metrics against which your organization measures the effectiveness of their value creation processes?” and gotten the blind stare (or to quote the movie “The Christmas Story”, they looked at me like I have lobsters crawling out of my ears).
Defining Value is a Team Sport
To facilitate an organization’s value definition process, I’ve developed the design template in Figure 2 as part of my “Thinking Like a Data Scientist” methodology. This design template guides organizations in identifying the KPIs and metrics against which they will measure the effectiveness of their business initiatives (as well as identify other important business initiative considerations such as ideal outcomes, benefits, potential impediments, and failure ramifications).
Figure 2: Step 1 in “Thinking Like a Data Scientist” methodology
While this is a good start, it is not sufficient to clearly define, communicate, and align the organization around how the organization creates and measures value. To achieve that, we need to get down to the individual stakeholder level – those stakeholders who either impact or are impacted by the business initiative.
The “Thinking like a Data Scientist” process collaborates across a diverse set of stakeholders to identify, validate, value, and prioritize the decisions that these stakeholders need to make in support of the business initiative, and gathering the KPIs and metrics against which the effectiveness of those decisions are measured (Figure 3).
Figure 3: “Thinking Like a Data Scientist” Stakeholder Persona
It is against these stakeholder decisions (and the supporting KPIs) outlined in Figure 3 that we will seek to deliver measurable, quantifiable business and operational outcomes that create new sources of customer, product, service, and operational value.
The AI-driven Decision Factory will excel at optimizing business process through the optimization of the decisions that drive business and operational outcomes at the frontlines of the organization. Where every customer engagement is an opportunity to learn more about the preferences and behaviors of that customer. Where every product interaction or usage is an opportunity to learn more about the performance behaviors of that product. Where every employee, supplier, and partner engagement are an opportunity to learn more about the effectiveness and efficiencies of your business operations.
Unfortunately, to achieve true digital and cultural transformation, organizations must move beyond just optimization and empower that natural human curiosity and intuition to innovate; to re-invent business processes and transforming business models. To achieve digital transformation, organizations much to move beyond business process “optimization” to “re-invention”.
To fuel reinvention, organizations much empower a culture of envisioning, ideating, exploring, trying, failing, learning, unlearning, sharing, and trying again in applying data and analytics to derive and drive new sources of value.
Empowering a Culture of Analytics-driven Innovation
Don’t just or optimize the cow path; re-invent it!
To re-invent business processes (and the decisions that the stakeholders need to make as part of that business process) requires organizations to empower a culture of innovation that integrates the decision optimization power of AI / ML with the process innovation power of Design Thinking.
Let’s say that you are a grocery retailer, and you want to re-invent the customer shopping experience. For example, trudging through the traditional customer journey to replenish my pantry when I discover that I am out of my favorite Cap’n Crunch cereal, I encounter multiple inhibitors of value creation, represented by red down arrows in the top value chain in Figure 4.
Figure 4: Re-inventing Customer Value Creation
However, integrating AI/ML decision optimization capabilities with Designing Thinking innovative mindset (to understand the customer journey decisions, pains, and gains), I can transform the customer purchase experience with a continuously-learning and adapting AI agent that can proactively alert me to a product shortage, or better yet, monitors my cereal consumption predicted propensities stored in my Analytic Profile to proactively predict and prescriptively deliver 2 boxes of the Cap’n before I realize that I’m even out of stock!
It isn’t about just optimizing the existing ordering process; it isn’t about just “paving the cow path.” Figure 4 requires the complete re-wiring—or re-invention—of the organization’s value creation process from the perspective of the key stakeholders. And each of those stakeholders represents another opportunity to integrate AI/ML capabilities with Design Thinking to create new sources of customer, product, service, and operational value.
In the blog “Is Analytics-driven Innovation the Ultimate Oxymoron?”, I introduced the “Pathway to Analytics-driven Innovation” map (Figure 5).
The “Pathway to Analytics-driven Innovation” map is a process for integrating customer journey-centricity (Design Thinking) with advanced analytics (Data Science) to translate an idea into a product or service that creates distinct, differentiated value (Economics).
The “Pathway to Analytics-driven Innovation” map (yep, yet again another framework from Schmarzo) navigates through the following stages:
The framework in Figure 5 seek to blend the best of the AI/ML decision optimization capabilities with the Design Thinking exploratory and creative mindset to empower an organization’s analytics-driven innovation capabilities.
Summary: Creating a Culture of Analytics-driven Innovation
The streets of downtown Boston are characterized by byzantine windings that seemingly defy logic — until one realizes that they trace the original cowpaths that cattle trod to skirt fields when ambling home from pasture in early colonial times. In downtown Boston, the streets are, indeed, paved cowpaths[1].
Integrating AI into your decision-making process to create an AI-driven Decision Factory is great… if your end goal is just to optimize your existing business processes. However, to thrive in a world of constant disruption and transformation requires more. It’s not enough to just optimize the cow path, organizations must seek to re-invent that cow path.
The key to moving beyond just optimization to reinvention is to empower a culture of ideation, exploration, trying, testing, failing, learning, and trying again. A culture of analytics-driven innovation includes:
The human-AI model collaboration isn’t just about creating a more compelling User Interface, it’s about empowering humans with customer, product, service, and operational insights uncovered in the data by AI / ML to drive curiosity, ideation, and eventually analytics-powered innovation.