Should You Have a Department of Artificial Intelligence? | Human-Centered Change and Innovation
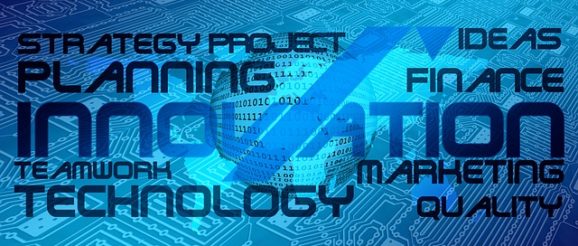
GUEST POST from Arlen Meyers
Several hospitals, academic medical centers and medical schools are creating artificial intelligence organizational centers, institutes and programs. Examples are Stanford, the University of Colorado , Children’s Hospital of Orange County and Duke.
If you are contemplating doing the same, think about what is the best organizational structure.? There’s a lot of debate about where AI and analytics capabilities should reside within organizations. Often leaders simply ask, “What organizational model works best?” and then, after hearing what succeeded at other companies, do one of three things: consolidate the majority of AI and analytics capabilities within a central “hub”; decentralize them and embed them mostly in the business units (“the spokes”); or distribute them across both, using a hybrid (“hub-and-spoke”) model. We’ve found that none of these models is always better than the others at getting AI up to scale; the right choice depends on a firm’s individual situation.
10. How will you measure results? Dr Anthony Chang, the co- founder of the American Board of Artificial Intelligence, suggests that the following are some helpful metrics to measure the artificial intelligence capabilities of the health system in the context of an individual AI project:
AI Project Score
The projects that involve machine learning and artificial intelligence, either clinical oradministrative, can be followed in stages (with each stage being scored 1 point each to a maximumof 5 points) and scored to keep track as well as maintain momentum:
Stage 1: Ideation. The project is first discussed and brought to a regular meeting for input from all stakeholders. This is perhaps the most important part of an AI project that is often not regularly done with enough discussion and consideration.
Stage 2: Preparation. After approval from the group, the data access and curation takes place in order to perform the ML/AI steps that ensue. The team should appreciate that this stage takes the most effort and will require sufficient resources.
Stage 3: Operation. After the data is curated and managed, this stage entails a collaborative effort during the feature engineering and selection process. Using the ML/AI tools, the team then creates the algorithms that will lead to the models that will be used later on in the project.
Stage 4: Presentation. Upon completion of the model with real world data, the project is presented in front of the group and depending on the nature of the project, it is either presented only or is also presented at a regional or national meeting or advanced to be published in a journal.
Stage 5: Implementation. Beyond the presentation and publication, it is essential for the AI project to be implemented in the real world setting using real world data. This project still requires continual surveillance and maintenance as model and data often fatigue.
11. Are you connected to the other parts of the healthcare AI ecosystem?
Feeding the organizational beasts that are resistant to change is hard. They have an insatiable appetite. Be sure your pantry is well stocked.
Image credit: Pixabay
Sign up here to get Human-Centered Change & Innovation Weekly delivered to your inbox every week.