AI Helps to Earlier Detect Brain Injury in Survivors of Cardiac Arrest – Polsky Center for Entrepreneurship and Innovation
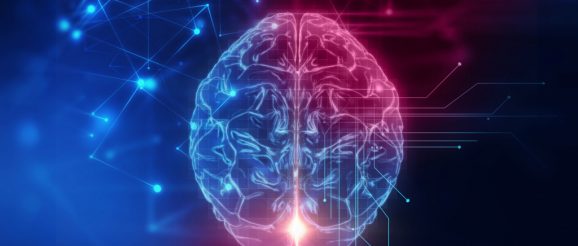
AI Helps to Earlier Detect Brain Injury in Survivors of Cardiac Arrest
The AI system improves the prognosis of surviving patients with Hypoxic Ischemic Brain Injury (HIBI) after cardiac arrest by allowing and facilitating earlier treatment. (Image: iStock/monsitj)
University of Chicago researchers have developed a patent-pending technique using deep learning, a form of artificial intelligence (AI), to better assess hypoxic-ischemic brain injury in survivors of cardiac arrest.
Over the past three decades, Maryellen Giger, A.N. Pritzker Distinguished Service Professor of Radiology, has been conducting research on computer-aided diagnosis, including computer vision, machine learning, and deep learning, in the areas of breast cancer, lung cancer, prostate cancer, lupus, and bone diseases.
She also is a cofounder of Quantitative Insights, which started through the 2010 New Venture Challenge at the Polsky Center. The company produced QuantX, which in 2017 became the first FDA-cleared machine-learning-driven system to aid in cancer diagnosis (CADx). In 2019, it was named one of TIME magazine’s inventions of the year and was bought by Qlarity Imaging.
Backed by this wealth of knowledge, she is today applying her research to neuro-imaging in collaboration with Fernando Goldenberg, a professor of neurology and neurosurgery, as well as the co-director of the comprehensive stroke center and director of neuroscience critical care at UChicago Medicine. The research team is enhanced with collaborators Jordan Fuhrman, a PhD student in Giger’s lab in the Committee on Medical Physics and the Department of Radiology, and Ali Mansour, an assistant professor of neurology and neurosurgery with expertise in advanced clinical neuroimaging and machine learning.
The goal of this multi-department research was to see if machine-learning could help clinicians at the hospital better assess hypoxic-ischemic brain injury (HIBI), which can occur when the brain does not receive enough oxygen during cardiac arrest. The extent of this damage depends on several variables, including the baseline characteristics of the brain and its vascular supply, duration of oxygen deprivation, and cessation of blood flow.
“While the neurological injury that follows cardiac arrest is largely a function of HIBI, the process of determining a patient’s projected long-term neurological function is a multifaceted endeavor that involves multiple clinical and diagnostic tools. In addition to bedside clinical exam, head CT (HCT) is often the earliest and most readily available imaging tool,” explained Goldenberg.
In their work, the researchers hypothesized that the progression of HIBI could be identified in scans completed on average within the first three hours after the heart resumes normal activity.
To test this, the team used machine learning, specifically, a deep transfer learning approach (which Fuhrman had been using to assess COVID-19 in thoracic CTs) to predict from the first normal-appearing HCT scan whether or not HIBI would progress. The deep learning technique, for which there is a patent-pending, automatically assessed the first HCT scan to identify the progression of HIBI.
This is important as currently there is no imaging-based method/analyses to identify early on whether or not a patient will exhibit HIBI, and while more data is needed to further confirm the efficacy of the AI-based method, the results to date are “very promising,” said Fuhrman.
“The findings in patients’ first HCT may be too subtle to be picked up by the human eye,” said Giger. “However, a computer looking at the complete image may be able to determine between those patients who will progress and eventually show evidence of HIBI and those who will not.”
According to the researchers, the AI system can help in the process of prognostication in survivors of cardiac arrest “by identifying patients who may differentially benefit from early interventions – a step along precision medicine in this patient population. If prospectively validated, it could also allow for the neuroprognostic process to start sooner than the current standard timeline,” said Mansour. Additionally, the AI algorithm is expected to be easily integrated into various commercially available image analysis software packages that are already deployed in clinical settings.
// Polsky Patented is a column highlighting research and inventions from University of Chicago faculty. For more information about available technologies, click here.