AI in healthcare: Real-world applications for cost-savings and innovation –
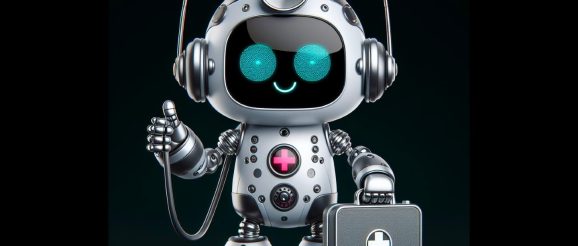
By Ramakrishnan Neelakandan The healthcare industry is under immense strain. Medical costs eat away at profits and strain household budgets, patients crave tailored treatment plans, and overworked professionals struggle with suffocating bureaucracy. To break this stalemate, businesses and providers alike are turning to transformative technology – artificial intelligence (AI), and, within the AI sphere, Large Language Models (LLMs). These innovative tools promise to revamp healthcare economics, delivering cost-effective solutions, patient-centric experiences, and much-needed relief throughout the system. Why AI, why LLMs, why now, and why you should care Costs out of control: Unsustainable medical spending burdens both businesses offering health insurance and individual consumers. AI tackles this head-on with accurate diagnoses, streamlined operations, fewer errors, and an emphasis on preventative care. LLMs add to this power, helping analyze complex medical texts for even deeper insights. The patient revolution: People want personalized healthcare, not cookie-cutter solutions. LLMs support this shift by combing through data for tailored care plans, understanding patient conversations, and accelerating the translation of research findings into real-world treatments. Boosting efficiency: Medical staff are overloaded with paperwork and repetitive tasks, impacting care quality and morale. AI, particularly LLMs, automate these burdens and empower proactive resource management. Consider chatbots to answer routine questions and virtual assistants for handling appointments, reminders, and personalized health advice. The how: AI and LLMs in action Let’s move from theory to practice. Here are a few real-world examples of how LLMs are already transforming healthcare: Spotting the invisible: The Mayo Clinic is now leveraging LLMs to analyze diverse patient data, including medical records, literature, and even genomic information. These models aim to uncover subtle patterns for incredibly early disease detection and potentially even predict patient responses to treatment. The right med, the right time: Analyzing drug interactions, flagging adherence issues, and optimizing complex drug combinations is a nightmare even for the most experienced doctors. Tools like MedAware, a leading AI healthcare solution, powered by LLMs, are changing that. They rapidly analyze vast databases of the latest medical journals at lightning speed and integrate their insights with patient data. This ensures patients get the safest, most effective medications tailored to their needs. Unclogging the workflow Healthcare is notorious for its paperwork. LLMs assist by pre-populating forms based on conversations, drafting follow-up emails to ease clinician load, and even helping navigate complex insurance documents. Suki, an AI-powered virtual assistant, is already making a difference in clinics nationwide. Suki uses LLMs to listen in on appointments, automatically generating accurate medical notes while the doctor focuses on the patient. Mental health: A sensitive area: Mental health care often relies on subjective assessments that can be inconsistent. LLMs have the potential to revolutionize this field. Imagine a tool analyzing patient journaling, social media posts, or even voice recordings to identify early signs of depression or anxiety. This level of continuous monitoring could lead to faster and more effective interventions. Projects like those at Stanford University are exploring how LLMs can help clinicians assess mental health needs more objectively and efficiently. The battle against superbugs: Antimicrobial resistance is a terrifying threat. LLMs can help by analyzing massive datasets to predict the evolution of drug-resistant bacteria. These models can also accelerate antibiotic development and even support doctors in making personalized antibiotic choices, leading to more effective treatment while minimizing the development of resistance. Beyond the clinic: LLMs for Healthy Habits: What if we extend the scope of AI healthcare beyond the clinical setting, shifting focus to prevention? LLMs could analyze diet and lifestyle data, tailoring personalized ‘nudges’ based on psychological insights. Think of an app that helps individuals identify negative thought patterns related to unhealthy eating or guides them towards activities based on their preferences and constraints. Revolutionizing medical education: Medical education is ripe for disruption. LLMs could create highly personalized lesson plans, adapting to each student’s learning style and knowledge gaps. They could curate relevant research, present complex concepts through engaging simulations, or even generate practice exam questions, enabling students to gain experience and confidence, all while reducing the burden on teaching faculty. LLMs are powerful, but quality and safety first As with any powerful technology, responsible implementation is vital: The bias trap: If LLM data is skewed, the results will be too. Rigorous testing and evaluation against diverse datasets is vital to ensure every patient gets the highest possible care. Having Humans in the loop for testing is necessary to ensure that the LLM is fair, equitable and safe for use. Privacy paramount: Patient data is sacred. AI security and privacy setup must be airtight to maintain trust and compliance. Ensuring compliance and robust data management policies is essential to gaining customer trust AI as a co-pilot, not autopilot: LLMs are incredible tools, but they must support, not replace, doctors or healthcare workers. Systems that enable transparency and offer explanations win over those that operate as black boxes. Utilizing transparent practices for LLM development can bring in efficiency and also confidence to the users. Conclusion The economic promise of AI and LLMs within healthcare is vast. By intelligently applying these tools, we’ll see a shift towards cost-effective, proactive care models centered on patient needs. This isn’t just about technology; it’s about transforming how we approach health entirely. Those embracing this change now will be rewarded with efficient organizations, happier patients, and a more sustainable healthcare system. About the author Ramakrishnan Neelakandan works at Google, leading software quality and safety efforts for their cutting-edge AI healthcare initiatives. A biomedical engineer by training, he brings over a decade of experience in the healthcare technology industry, having previously worked with leading pharmaceutical and medical device companies.