Artificial intelligence expert Kate Crawford on why people should be concerned about the innovation’s risk
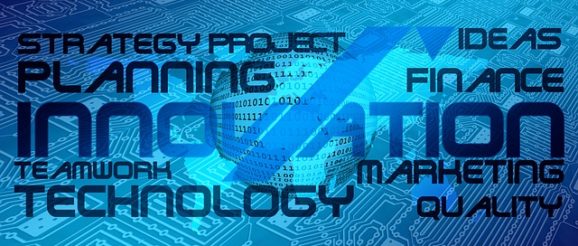
We’re heading to a city 7-Eleven, but outside an internet cafe on the fringes of Chinatown, Crawford stops and looks up. “That’s it,” she exclaims, pointing up at a series of arched windows on a vintage building. It’s the New York-loft-style space where she lived in the early 2000s while lecturing in media and communications at the University of Sydney and writing her PhD on adulthood and technology.
At the same time, Crawford was writing music as one half (with Nicole Skeltys) of the avant-garde electronic dance music duo B(if)tek. Now, looking back at old B(if)tek videos and their sci-fi visual tropes, it’s as though there’s a direct line between the irony-rich compositions and Crawford’s contemporary work on AI. “We’re interested in ‘the human/machine divide’,” Crawford told The Sydney Morning Herald in 2003. B(if)tek’s track Machines Work uses a sample from a 1967 IBM word processor ad: “Machines can do the work, so that people have time to think.” A single, We Think You’re Dishy, includes the lines, “We are the B(if)tek corporation/we have been watching you.”
Someone will be watching you if you grab a Slurpee at a 7-Eleven. Outside the outlet, we read a notice on the window: “By entering the store you consent to facial recognition cameras capturing and storing your image.” Inside, we study an array of CCTV cameras on the ceiling before engaging with a tablet device on the counter. “How did we do today?” is the question on the screen above four faces emoting levels of satisfaction, from the green-smiley “awesome” to the red-angry “awful”. Subsequent questions probe the main reason for my visit and whether staff have asked if I have the “My 7-Eleven” app. It’s only as I tap in my final responses to the survey that I notice the screen’s small black camera “eye”.
“How would you know how this data is being processed; it could be connected to facial recognition or emotion detection technology,” says Crawford as we leave the shop and its prying eyes. For some years she’s been arguing for a moratorium on the use of facial recognition technology. Later, I email questions to 7-Eleven. The reply is swift: a spokesperson says the chain uses the tablet-based system to solicit customer feedback and when someone chooses to do the survey, the tablet captures their image to filter out “potential duplicates”. He says CCTV cameras are for security purposes only and do not operate with facial recognition or other biometric technology. Both the CCTV footage and images captured when customers complete the tablet survey are deleted within weeks. Nevertheless, the Office of the Australian Information Commissioner confirmed to me that it is investigating 7-Eleven’s use of facial recognition technology.
Biometric technology, which uses one or more of a person’s physical or biological characteristics for the purposes of identification, has been around since before Arthur Conan Doyle gave Sherlock Holmes a magnifying glass and pointed him in the direction of some fingerprints. But as the Australian Human Rights Commission’s report notes, it has been “supercharged by AI” and its new capabilities to analyse large sources of data.
Facial recognition technology, a subset of biometrics, maps the geometry of a face, the length of a nose, the curve of a mouth, the distance between brow and eyelid. It then uses AI to compare the face to either another image of the same person (one-to-one facial verification such as that used at Australian airport passport control, SmartGates) or a larger “one-to-many” database of images, such as that which might be held by a police department. Or that used by Chinese authorities to identify people for investigation and/or detention in the Uighur autonomous region of Xinjiang. Or a database such as the one containing more than three billion faces owned by a shady New York-based facial recognition software company with connections to the far right, Clearview AI.
“I would imagine all of us, you, me, anyone who’s ever had a picture on the internet, is probably in the Clearview AI system,” Crawford says as we walk towards the towers of Barangaroo, where Facebook has its Australian headquarters. The story of Clearview AI and its three billion faces offers an insight into the multi-faceted issues connected to AI and personal data: how data is extracted, how it is classified, how it is used and its potentials for harm.
Last year, The New York Times published an article about Clearview, headlined, “The secretive company that might end privacy as we know it.” It revealed that Clearview’s database of faces had been “scraped” from public sites including employment and news sites, and social networks such as Facebook, and includes links to the images’ online sources. Clearview told the NYT that more than 600 law-enforcement agencies were using its technology to assist in investigating crimes including shoplifting, identity theft and child sex-abuse. A month later, Buzzfeed reported that Clearview was working with more than 2000 agencies around the world and that four Australian police forces (the Australian Federal Police and the Queensland, Victorian and South Australian forces) had tested its technology.
Concerns about Clearview AI are legion, including its secrecy, the almost inconceivable invasion of privacy its scraping practices constitute, the chance for errors in matches, and in the genie-out-of-a-bottle dystopia its technology has the potential to launch. The NYT article noted that computer code underlying the Clearview app includes programming language to pair it with augmented-reality glasses. “Users would potentially be able to identify every person they saw. The tool could identify activists at a protest or an attractive stranger on the subway, revealing not just their names but where they lived, what they did and whom they knew,” the article said. “Searching someone by face could become as easy as googling a name. Strangers would be able to listen in on sensitive conversations, take photos of the participants and know personal secrets … It would herald the end of public anonymity.” (Clearview told the NYT that it had designed a prototype for use with augmented-reality glasses but had no plans to release it.)
The backlash against Clearview, and to facial recognition technology more broadly, has been considerable. The Office of the Australian Information Commissioner and the UK Information Commissioner’s Office are jointly investigating Clearview. In February, the Canadian privacy commissioner announced Clearview was no longer welcome in Canada and should delete Canadian faces from its database. “It is completely unacceptable for millions of people who will never be implicated in any crime to find themselves continually in a police line-up,” the privacy commissioner Daniel Therrien said. In May, a number of European privacy organisations announced they had filed legal complaints against Clearview.
But corporations are not the only organisations to fear: if George Orwell taught us anything, it’s that we also need to keep a close watch on our governments. The federal government’s attempts to create a national facial recognition database that would enable “one-to-many” identification were temporarily stymied in 2019 when the Parliamentary Joint Committee on Intelligence and Security rejected the proposed legislation, arguing it had insufficient safeguards to protect people’s privacy and rights. Redrafted laws will inevitably resurface at some point. They are likely to ignore one of the key recommendations in the Australian Human Rights Commission’s report: that all governments should introduce a moratorium on the use of facial recognition and other biometric technology “in decision-making that has a legal, or similarly significant, effect for individuals, or where there is a high risk to human rights, such as in policing and law enforcement”.
Facial recognition algorithms get things wrong. Video surveillance cameras clocked the faces of thousands of people during London Metropolitan Police Service trials of facial recognition technology which started in 2016. Of the “suspects” identified in the trials, more than 80 per cent were incorrect matches; they were in fact innocent passers-by. And here’s another one: the story of Robert Williams, a Detroit black man accused of stealing watches from a luxury store and arrested in his driveway last year while his wife and kids watched. “This is not me,” Williams said when, during questioning, police showed him a grainy photograph of the suspect. The photo had been run through the Detroit Police Department’s facial recognition system and incorrectly matched with an old drivers’ licence photo of Williams. According to the MIT Technology Review, the department’s AI system was responsible for two other false arrests. They were both black men, too.
“People of colour, women, people with physical disability are all far less likely to be accurately identified using facial recognition than basically people who look like me: white, middle-aged men.”
“What we know is that errors are not evenly distributed across the community,” says Australian Human Rights Commissioner Edward Santow. “People of colour, women, people with physical disability are all far less likely to be accurately identified using facial recognition than basically people who look like me: white, middle-aged men.”
But issues of bias in AI go far beyond the potential for facial recognition errors. Bias and discrimination can be embedded in the very “brains” of AI systems. To understand how this happens, it helps to understand the process through which artificial intelligence becomes “intelligent”. Our publicly available digital personal data is the fuel that powers AI, and when our faces are “harvested”, they might not just be used for the purposes of facial recognition/identification. They can be classified and included in vast “training datasets” which are used to build algorithms for AI functions including object detection and language prediction.
Consider, for example, the task of building an AI system to detect the difference between an apple and an orange: as Crawford explains in Atlas of AI, a developer must first collect, label and train a neural network using thousands of labelled images of apples and oranges. Algorithms then conduct a statistical survey of the images and develop a model to recognise the difference between the two fruits.
To mount the Training Humans exhibition in Milan, Crawford and Trevor Paglen spent two years poring over training sets, including the ground-breaking ImageNet, which was created in the mid-2000s to map the entire world of objects and is led by researchers from a number of American universities. The pair were shocked by what they found in the images’ labels. Sure, some made sense: cat, dog, apple, tree. But then they looked at how people were categorised. “You look at ‘CEO’ and ‘Oh, it’s mostly white men, that’s interesting,’ then you look at ‘basketballer’ and it’s mostly black men. Then the categories start to shift and become moral judgments. So you have ‘bad person’, you have ‘kleptomaniac’ … the most appalling racist and misogynistic epithets.” Crawford recalls seeing a young woman’s graduation photo. “She had a low-cut dress on and now she’s in the ‘slattern’ training category.”
The labels had been imported from a database of words, WordNet and, through the crowdsourcing labour platform Amazon Mechanical Turk, given to a global army of “digital pieceworkers”, each of whom was paid a few cents an hour to label 60 or more images a minute. “It’s this idea that you can create ground truth from scraped flotsam and jetsam off the internet,” Crawford says.
Atlas of AI notes multiple other examples of embedded discrimination, including gender bias in Apple’s creditworthiness algorithms, chatbots that adopt racist and misogynistic language, voice-recognition software failing to recognise female-sounding voices, and social media platforms showing more highly-paid job advertisements to men than to women.
In June, The New York Times published a story about the increasing number of start-ups offering systems to identify and remove bias from AI systems. In an echo of the Robodebt debacle in Australia, the article noted the US Federal Trade Commission’s recent warning to companies about the sale of racially biased AI systems or those which could prevent individuals from receiving employment, housing, insurance or other benefits. The article also shared an example: in 2018, an American AI start-up aiming to build a system to remove nude or explicit images from the internet sent millions of photos to workers in India who were to tag explicit material. When the work was finished, the start-up discovered a problem: the workers had tagged every image of a same-sex couple as “indecent”.
Highlighting the processes in how some AI is created, the Training Humans exhibition displayed imagery – as Crawford puts it, “scraped flotsam and jetsam off the internet” – used to “train” AI systems.Credit:Marco Cappelletti/Courtesy Fondazione Prada
Crawford and I have roamed Barangaroo’s shadowy lanes, passed through multiple revolving doors into gleaming office tower lobbies, studied tenants’ directories, followed security officers’ directions into other lobbies, and still have not seen even a hint of Facebook’s headquarters. “It’s like an Escher experience of public space, like, ‘You’re not welcome, we do not want people coming to engage with us’,” Crawford says of our fruitless in-and-out, up-and-down search.
“It’s not public infrastructure, it’s for profit; they have no obligation to make sure information is accessible, even in a health crisis.”
How clever Facebook has been: it has persuaded us to think it’s for us, for everybody, for soccer mums and community groups and reconnecting with old friends. Instead, as Crawford explains, the trillions of photos, voice clips and bites of text that make up our Facebook content (and our content on Instagram, which was bought by Facebook in 2012) is training one of the largest AI systems in the world which, in turn, is creating an “unbelievably powerful” facial recognition engine. “It’s not public infrastructure, it’s for profit; they have no obligation to make sure information is accessible, even in a health crisis.”
Crawford was appalled when, in February, Facebook blacked out Australian mainstream media organisations’ newsfeed posts over a new law requiring tech giants to pay for content. “It was shocking. That was one of the most stark experiences of platform power, the power to say, ‘We don’t like the way this negotiation is going, we’ll switch off the pipes thank you very much.’ And that, to me, is the most telling experience of how hard it’s going to be to regulate Big Tech.”
The episode supports one of Crawford’s greatest fears about AI: that it gives more power to a highly concentrated sector of already powerful tech companies. “Historically, I don’t think there’s a comparison in the widening asymmetries of power.” But in countless ways, the AI power imbalance will also increasingly be felt at a micro level in our everyday lives, especially in the relationship between bosses and workers.
For generations we’ve worried that robots are coming to take our jobs but, as Crawford notes, the greater threat might be that humans will increasingly be treated like robots. As we’ve walked through the city, we’ve noticed innumerable “For lease” signs on office buildings, a reminder that, around the world, the pandemic has created armies of remote workers.
Get ready for an era of invasive algorithmic management in which your boss watches you remotely (in “stealth mode”, if they choose): how long you are online, what tabs you have open on your screen, how efficient you are.
If your kid works in a fast-food outlet or a shop, AI management systems will monitor performance indicators such as how fast they make a cheeseburger, or whether they push-sell to customers (no, the 7-Eleven staffer didn’t ask me if I have the “My 7-Eleven” app – will he be in trouble?). If you work in a warehouse or a factory, such as an Amazon “fulfilment centre”, surveillance systems will record your “picking rate”: the rate at which you gather products to meet orders. For Atlas of AI, Crawford visited a mammoth Amazon fulfilment centre in New Jersey. She observed multiple workers with knee braces, elbow bandages or wrist guards. At intervals through the factory, she says, vending machines are “stocked with over-the-counter painkillers for anyone who needs them”.
In a letter to Amazon shareholders in April, Jeff Bezos noted that, to decrease work-related musculoskeletal disorders, the company was automating staff schedules using “sophisticated algorithms” to shuffle staff between jobs using different muscle-tendon groups. “They’re resisting unionisation every step of the way and using rampant surveillance technology to try to produce the most efficient mechanism to extract value from human bodies down to the level of muscles and ligaments,” says Crawford. By the end of this year, Australia will have six Amazon fulfilment centres, including a new “robotics fulfilment centre” the size of 24 rugby league fields in western Sydney.
In her book’s final, chilling chapter, Crawford stops beside a road in west Texas and looks across a valley towards Jeff Bezos’s Blue Origin rocket launch facility. She describes it as “a techno-scientific imaginary of power, extraction, and escape … a hedge against Earth”. As she pulls her car back out onto the road, she realises she has been watched. Two black pick-up trucks start to tailgate her. “They maintain their sinister escort all the way to the edge of the darkening valley.”
To read more from Good Weekend magazine, visit our page at The Sydney Morning Herald, The Age and Brisbane Times.
The best of Good Weekend delivered to your inbox every Saturday morning. Sign up here.