AWS names 6 key trends driving machine learning innovation and adoption | VentureBeat
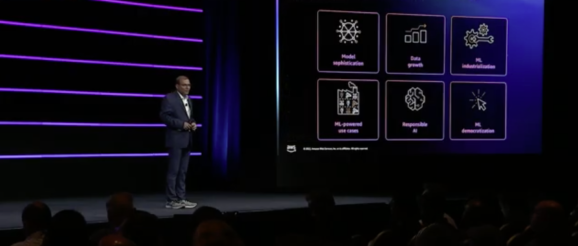
Check out the on-demand sessions from the Low-Code/No-Code Summit to learn how to successfully innovate and achieve efficiency by upskilling and scaling citizen developers. Watch now.
Machine learning (ML) has undergone rapid transformation and adoption in recent years, driven by a number of factors.
There is no shortage of opinions about why artificial intelligence (AI) and ML are growing. A recent report from McKinsey identified industrializing ML and applied AI as among its top trends for the year. In a session at the AWS re:Invent conference this week, Bratin Saha, VP and GM of AI and machine learning at Amazon, outlined the six key trends the cloud giant is seeing that are helping to drive innovation and adoption in 2022 and beyond.
AWS claims to have over 100,000 customers for its AI/ML services. These services are spread across three tiers: ML infrastructure services, enabling organizations to build their own models; SageMaker, which provides tools to build applications; and purpose-built services for specific use cases, such as transcription.
“Machine learning has transitioned from being a niche activity to becoming integral to how companies do their business,” Saha said during the session.
Learn the critical role of AI & ML in cybersecurity and industry specific case studies on December 8. Register for your free pass today.
Trend 1: Model sophistication is growing
Saha said that in recent years there has been an exponential increase in the sophistication of ML models. His use of the term “exponential” isn’t hyperbole either.
One way to measure machine learning models’ sophistication is by counting the number of parameters within them. Saha explained that parameters can be thought of as variables of values that are embedded inside ML models. In 2019, Saha said, then-state-of-the-art ML models had approximately 300 million parameters. Fast forward to 2022 and the best models now have more than 500 billion.
“In other words, in just three years, the sophistication of machine learning models has increased by 1,600 times,” Saha said.
These massive models are what are now commonly referred to as foundation models. With the foundation model approach, an ML model can be trained once, with a massive dataset, then reused and tuned for a variety of different tasks. Thus enterprises can benefit from the increased sophistication, with an easier-to-adopt approach.
“[Foundation models] reduce the cost and effort of doing machine learning by an order of magnitude,” Saha said.
Trend 2: Data growth
Increasing volumes of data, and different types of data, are being used to train ML models. This is the second key trend Saha identified.
Organizations are now building models that have been trained on structured data sources such as text, as well as unstructured data types including audio and video. Having the ability to get different data types into ML models has led to the development of multiple services at AWS to help in training models.
One such tool that Saha highlighted is SageMaker Data Wrangler, which helps users process unstructured data using an approach that makes it practical for ML training. AWS also added new support for geospatial data in SageMaker this week at the re:Invent conference.
Trend 3: Machine learning industrialization
AWS is also seeing a trend of increasing ML industrialization. That means more standardization of ML tools and infrastructure, enabling organizations to more easily build applications.
Saha said that ML industrialization is important because it helps organizations automate development and make it more reliable. An industrial, common approach is critical to scaling as organizations build and deploy more models.
“Even within Amazon we are using SageMaker for industrializing and machine learning development,” Saha said. “For example, the most complex Alexa speech models are now being trained on SageMaker.”
Trend 4: ML-powered apps for specific use cases
ML is also growing because of purpose-built applications for specific use cases.
Saha said that AWS customers have asked the vendor to automate common ML use cases. For example, AWS (and other vendors) now offer services such as voice transcription, translation, text-to-speech and anomaly detection. These give organizations an easier way to use ML-powered services.
Sentiment analysis in live audio calls, for example, is a new, complex use case that AWS now supports with the real-time call analytics capabilities of its Amazon Transcribe service. Saha said that the feature uses speech recognition models to understand customer sentiment.
Trend 5: Responsible AI
There is also a growing trend, and need, for responsible AI.
“With that growth in AI and ML comes the realization that we must use it responsibly,” Saha said.
From AWS’ perspective, responsible AI needs to have several key attributes. A system needs to be fair, operating equally for all users regardless of race, religion, gender and other user attributes. ML systems also need to be explainable, so organizations understand how a model operates. Also needed are governance mechanisms to make sure responsible AI is being practiced.
Trend 6: ML democratization
The final key trend that will drive ML forward is democratizing the technology, making tools and skills accessible to more people.
“Customers tell us that they … often have a hard time in hiring all the data science talent that they need,” Saha said.
The answers to the challenge of democratization, in Saha’s view, lie in continuing to develop low-code and use case-driven tools, and in education.
“AWS is also investing in training the next set of machine learning developers,” Saha said. “Amazon has committed that by 2025 we will help more than 29 million people improve their tech skills through free cloud computing skills training.”
VentureBeat’s mission is to be a digital town square for technical decision-makers to gain knowledge about transformative enterprise technology and transact. Discover our Briefings.