Battling Fraud Through Innovation: Your Data Quality Depends on it
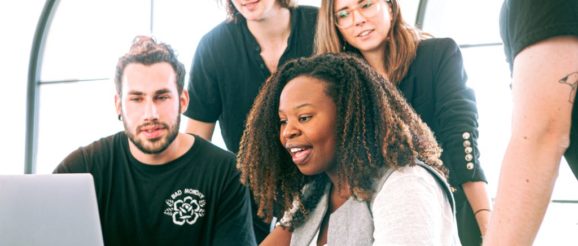
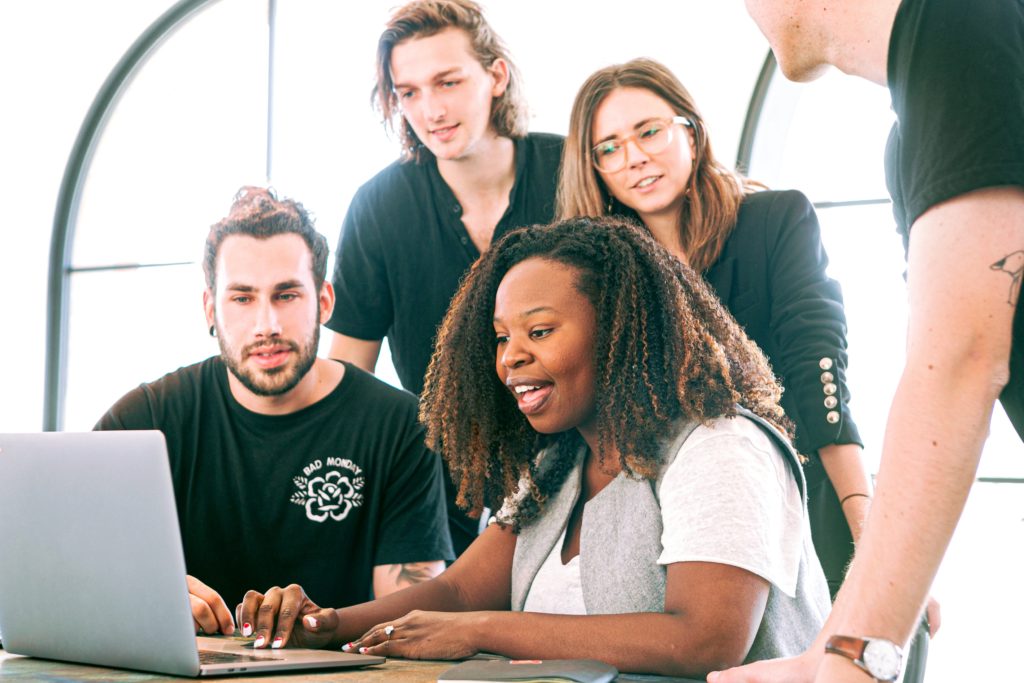
We talk a lot in the market research industry about what needs to change and the challenges we face, but there are some notable innovations happening as well. In fact, we’re seeing positives like rising respondent engagement with suppliers focusing on experiences and using technology like automation to even turn down “bad” studies that are long and complicated. Client-side researchers are showing commitment to better surveys that are shorter and easier for respondents to navigate. Actions such as these are producing better data quality.
The Battle Against Fraud
But as technology use rises to help quality, there is a flip side. Fraud is also evolving and it’s on the rise. Fraudsters are using technology to increase their activities in a number of industries. One space that’s been experiencing this for years is AdTech. They see organized crime – in the form of fraud – inflating traffic and clicks on campaigns as malevolent players attempt to monetize fake traffic. In fact, ad fraud is the fastest-growing segment of cybercrime with estimates showing that advertisers are losing an estimated $19 billion to fraudulent activities.
Market research is not immune. Bad behavior is apparent in our space as hackers find gaps in our traditional fraud mitigation approaches. At Cint, we track a multitude of data points, so this increase in fraud is not just conjecture. When tracking subsets of data relating to fraud, we have been seeing patterns that indicate fraud is growing.
In order to battle fraudulent behavior, we started by uncovering the kinds of fraud we were dealing with when bad data popped up in a survey. We noted issues from the full panelist lifecycle ranging from unengaged respondents to script fraud to no data at all, including the number of completes outnumbering the number of people who actually entered the survey. This “ghost completes” problem is becoming more prevalent, which can be simply explained as link manipulation where respondents are being paid incentives without actually completing a survey. These kinds of behaviors can have a wide-reaching impact on the market research ecosystem if left unchecked. As the sophistication and agility of fraudsters rise, they are beginning to conduct fraud at a larger and larger scale, potentially affecting data quality.
How to Combat Fraud
So what can we do to combat these trends? Our methods must rise in sophistication as well. Many companies are coming up with proprietary solutions to help in the market research space, and some expertise from outside the industry is being employed as well. There are a few best practices that we can all employ in order to combat fraud at scale:
- Knowledge sharing: Share information that is gathered along all parts of the value chain – from recruitment to data collection and more – and this will help to build a holistic, deep view of the panelists and their behaviors.
- Secure traffic: Review how you communicate with your suppliers and choose secure server-to-server approaches over standard redirects.
- Fraud mitigation tools: Employ a combination of fraud detection tools such as deduplication monitoring, IP checks, Captcha techniques and open-end validation.
- Dynamic prevention: Combine traditional mitigation techniques with new dynamic approaches using artificial intelligence and machine learning to stay a step ahead of the organized, agile fraud of today.
Many suppliers who take fraud mitigation seriously have made investments in proprietary tools and techniques, using them at registration, during the sampling and survey stages, and again at the reward stage. Just as fraudsters are figuring out new ways to get around the barriers we construct, we need to constantly battle them with a dynamic approach. AI holds great promise on this front as it can quickly catch bad behavior before it affects data quality through things like pattern recognition and introducing open-ended questions at different points throughout the survey.
In fact, as part of our acquisition of P2Sample earlier this year, Cint will be using billions of data points, demographic and behavioral, to detect and combat fraud. Sophisticated artificial intelligence algorithms can analyze anomalies and patterns in the data, which could encompass, for example, user “surges” involving specific demographics, IP ranges or completion time frames. This proprietary AI will allow proactive detection and mitigation of fraudulent activity.
Challenges surrounding fraud are not going to be solved with one stroke of the AI-powered magic wand, but it is an innovation that holds great promise for the future of data quality. The battle against fraud is a race to stay ahead of organized fraudsters that are savvy and nimble. If the industry continues to work together to address these issues, high data quality will prevail.