Exploring a leading and lagging regions dichotomy: does entrepreneurship and diversity explain it? | Journal of Innovation and Entrepreneurship | Full Text
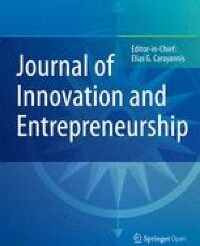
Regional growth theory offers different perspectives on specialization as either advantageous or potentially detrimental to regional growth. Broadly, these theories consider the presence and importance of different types of agglomeration economies (McCann, 2009). In this section, we do not intend to provide a comprehensive review but we briefly discuss some of these perspectives. Our empirical analysis draws mainly on arguments that are emphasized in the literature on endogenous growth determinants—the implications of industry specialization vs. diversity for knowledge externalities, and the role of entrepreneurship for regional growth (Capello, 2009). Accordingly, this section first considers agglomeration economies that are associated with industry composition, followed by a brief discussion of the role of entrepreneurship for regional growth.
Specialization, diversity, and regional growth
The notion that specialization is important for regional growth is not newFootnote 3. Arguments for such a relationship traces back to Adam Smith (1776) and to David Ricardo (1817) who contributed with the theory of comparative advantage. Krugman (2009) succinctly defines comparative advantage as meaning that “countries trade to take advantage of their differences.” The notion of comparative advantage has important implications for regional analyses. Drawing on comparative advantage, the work of Heckscher (1919) and Ohlin (1924) became the foundation of the Heckscher-Ohlin model (see for ex. Jones, 1956), which predicts that regions will specialize in producing and exporting products that are intensive in their relatively abundant factors of production (e.g., Kim, 1995). The local availability of inputs is however only one of several factors that may influence the location decision of firms. It is not our ambition to provide a comprehensive review of location theory, nor to review the literature on regional growth in this paper. Instead, we draw on for example Frenken et al. (2007) who note that the economics of agglomeration posits that economic activity tends to cluster because firms experience benefits from locating near other firms. Frenken et al. (2007) outline four sources of such benefits, or agglomeration economies, which we discuss briefly here.
First, the field of New Economic Geography (NEG), which in particular builds on the work of Krugman (1991), emphasizes increasing returns to scale as a benefit of agglomeration. According to NEG, the presence of large economies of scale, low transport costs, and enough mobile production can lead to a self-sustaining spatial concentration of production (i.e., specialization), and its location can be a result of initial conditions or mere historical “accident” (Krugman, 2009).
The second source of agglomeration economies discussed by Frenken et al. (2007) is localization economies. These reflect Marshallian externalities that emanate from sectoral specialization, which enables labor market pooling, creation of specialized suppliers, and fosters knowledge spilloversFootnote 4. Localization economies are thus available to all same-industry firms in the area. Stigler (1951) emphasized the relationship between size and degree of specialization: the creation of specialized suppliers requires that the industry or market is sufficiently large.
The third type of agglomeration benefit that Frenken et al. (2007) identify consists of external economies that arise from urban size and density. These urbanization economies are available to all local firms. This concept reflects advantages such as a dense presence of diverse economic, social, political, and cultural organizations and actors, typically associated with large urbanizations (cities). Florida, Adler, and Mellander (2017) argue that cities have distinct advantages as they provide “the enabling infrastructure where connections take place, networks are built and innovative combinations are consummated.”
This line of reasoning is connected to the fourth source of agglomeration economies outlined by Frenken et al. (2007)—Jacobs externalities. This concept draws on the work of Jane Jacobs (1969), who emphasized variety and diversity of industries as conducive to innovation and growth due to knowledge spillovers between firms in different industries. Frenken et al. (2007) argue that knowledge spillovers between industries should facilitate radical innovation and product innovation in particular, as knowledge and technologies are recombined in new ways.
Whether regions benefit more from specialization or diversification remains a contested issue which is summarized in the “MAR vs. Jacobs” controversy described by for example Content and Frenken (2016). The empirical evidence on these theories is mixed (e.g., De Groot et al., 2016). Frenken et al. (2007) made an important contribution to this literature with the notion of related variety, which implies that when a diversified economy is characterized by industries that are related in a technological or market sense, it is especially supportive of innovation and regional economic growth, as it fosters Jacobs-type knowledge externalities. This notion has gained increasing empirical support (e.g., Boschma & Iammarino, 2009; Da Silva, Goncalves, & De Araújo, 2019; Miguelez & Moreno, 2018; Östbring & Lindgren, 2013; Tavassoli & Carbonara, 2014; van Oort, de Geus, & Dogaru, 2015).
In summary, this limited account of some basic perspectives found in regional growth theory indicates that the field offers a diverse set of explanations for the sources of advantages which lead some regions to prosper while others lag behind. These advantages may include the presence of comparative advantages, economies of scale, and knowledge spillovers between or within industries, facilitated by urban size and density. In this paper, we are particularly interested in the role of industry composition and hence, our empirical analysis will focus mainly on two types of external economies: localization economies and Jacobs externalities. In that sense, we contribute to the literature on “MAR vs. Jacobs.”
Entrepreneurship and regional growth
Solow (1956) argued that economic growth is determined by the stocks of capital and labor. Later, researchers have emphasized that investments in knowledge is an important source of growth since it also spills over for use of third parties (Romer, 1986). Audretsch and Keilbach (2004a) in turn argued that such spillovers require economic agents which create and make use of diversity of knowledge. As such, entrepreneurship has been deduced as an important mechanism facilitating the spillover of knowledge through creating diversity of knowledge (Audretsch & Keilbach, 2004b).
There are several empirical studies examining the relationship between regional levels of entrepreneurship and economic growth. Holtz-Eakin and Kao (2003) performed an empirical test of variations in the birth rate and the death rate for firms across different states in the USA and they found this measure of entrepreneurship to have a positive impact on productivity growth. Audretsch and Fritsch (2002) found similar results where entrepreneurship had a positive impact on regional growth in Germany. Also, Foelster (2000) has found that regional levels of self-employment influence regional growth in Sweden. Callejon and Segarra (1999) performed a study in Spain where they found that new-firm startup rates and exit rates both contribute positively to the growth of total factor productivity in regions. As such, there is ample support suggesting regional entrepreneurship to be positively related to regional growth.
Since cluster membership is a categorical variable, we employ multiple discriminant analysis to examine how a set of independent continuous variables on regional characteristics such as entrepreneurship and specialization perform in predicting group membership. We aim to examine if the level of specialization and other characteristics in one period seems to influence future growth performance, and therefore we mainly use independent variables covering the year 2010, while the dependent variable (group membership) is constructed based on growth between 2010 and 2015. This approach differs from the extant literature (e.g., Frenken et al., 2007) which typically employs multiple regression analysis and treats economic outcomes in a direct fashion as continuous measures of dependent variables, for example, growth in regional employment or GDP. Our approach enables us to conceptualize economic outcomes as regional growth trajectories that combine economic and demographic development in categorical region types (i.e., lagging, leading, etc.) which may provide multidimensional and nuanced perspectives to the literature. We do not claim that this approach is necessarily better than typical multiple regression analyses, but rather it is complementary. The results shed light on the role of entrepreneurship, industry structure, and other aspects of regional economies.
Operationalizing specialization
In a study on regional industrial specialization and risk sharing in the EU15, Basile and Girardi (2010) argue that there exists no optimal measure of specialization. In addition, they find that the conventional indices are strongly correlated. They instead assert that a more critical issue is the choice of variable. In their view, specialization can be observed from the input side through employment or from the output perspective by using measures such as value added or exports. Basile and Girardi (2010) favor employment data, as it is less sensitive to valuation problems compared to production data. A third option is proposed by for example Nakamura (2009), who suggest that the number of firms or plants may provide a different perspective than the more conventional expression in terms of employment.
A common approach is to operationalize regional economic specialization through some relative measure of employment specialization. We follow this conventional approach and operationalize three different conceptualizations of specialization or diversity through the use of employment data. We choose the Herfindahl-Hirschman index (HHI) as a measure of the overall level of regional specialization, i.e., measuring the variety of industries in a region. The HHI is computed as follows. Let Si denote the number of industries in region i, esi is employment in region i in industry s, ei is total employment in region i. The HHI for each region is then computed as follow:
A value near zero indicates a highly diversified regional economy and a value of 1 means that the region is completely specialized in a single sector. Following Kemeny and Storper (2015), we use disaggregated data and compute regional Herfindahl indices using five-digit SNIFootnote 5 2007 employment data. These data are fully compatible at the four-digit level with the NACEFootnote 6 Rev.2 classification used by Eurostat. We use the same data to compute an indicator of related variety (RV), following the formula proposed by Frenken et al. (2007). It is computed as follows: employment in a five-digit industry i belongs to an aggregate sector Sg (g = 1, …, G), which is at the 2-digit level in this study. Pg is the 2-digit sector’s share of total employment in the region, and Pi is the 5-digit industry’s employment share. We then calculate related variety (RV) as follow:
where:
High values of RV relative to other regions suggest that the industry structure is relatively more characterized by industries that are related in a technological sense. We select a five-digit SNI level since high levels of aggregation may lump industries together as comparable specializations, when in reality they are “apples and oranges” (Kemeny & Storper, 2015).
Operationalizing entrepreneurship
There is compelling empirical evidence on the link between entrepreneurship and regional growth and development (Audretsch & Keilbach, 2004a; Fritsch & Wyrwhich, 2017). Entrepreneurship is a multidimensional concept that is challenging to measure empirically (Fischer & Nijkamp, 2009). We use the concept of entrepreneurship capital (Audretsch & Keilbach, 2004a), which is measured by the number of startups in a region relative to its population. High values of entrepreneurship capital relative to other regions indicate “a regional milieu of agents that is conducive to the creation of new firms” (ibid). We obtain data on new business formation from a database maintained by the Swedish government agency Growth Analysis (Tillväxtanalys). Fritsch (2015) emphasizes that the impacts of new businesses may take considerable time to manifest, even up to ten years. To account for this, we calculate an average rate of new business formation per 1000 inhabitants in ages 16-64 for the years 2008-2010. This treatment of entrepreneurship capital is similar to the approach used by Audretsch and Keilbach (2004a), who compute their measure based on the number of startups between 1989 and 1992, where 1992 is the base year of their analysis.
Additional independent variables
We include the initial level of economic output in 2010, which we measure as GRP (i.e., regional GDP) per capita. This variable is intended to account for the possibility that regions that were highly productive in 2010 may enjoy competitive advantages, while regions that are lagging behind may struggle to overcome disadvantages that are unobserved in this analysis.
Furthermore, there exists compelling support for the notion that human capital is associated with economic development (e.g., Barro, 2001; Lucas, 1988). We therefore include the share of each FA-region’s population in the ages 20 to 64 years with tertiary (post-secondary) education of at least 3 years (i.e., equivalent of bachelor’s degree), again for the year 2010. The use of educational attainment as a measure of human capital endowment is not without critique (Florida, Mellander, & Stolarick, 2008), yet it remains a common measure of this concept in the empirical literature (e.g., Lee, Florida, & Gates, 2010; Tavassoli & Carbonara, 2014).
There are certainly other regional characteristics that would be relevant to include in the analysis. One example is to include a variable which captures urbanization economies (i.e., region size and/or density). However, our relatively small sample size poses a limitation since we apply discriminant analysis. Hair Jr. et al. (2014) suggest a ratio of 20 observations for each independent variable, with a minimum recommended size of five observations per independent variable. For this reason, we restrict the number of variables in this exploratory analysis.
Summary statistics of independent variables
Table 3 lists our independent variables. Our data contained extreme outliers for GRP per capita (n = 3 regions dominated by capital-intensive extractive industries). Discriminant analysis is sensitive to outliers Hair Jr. et al., 2014, and for this reason, we eliminate the extreme outliers. After this, several variables still display non-normal distributions, which we address by transforming GRP10 and HHI10 to their natural logarithms.
We estimate the following discriminant function:
Where Z is the discriminant score of function j for region r, W denotes the discriminant weights for each independent variable, lnGRP is the natural logarithm of GRP per capita in 2010, Humcap is the share of population age 20-64 years with at least 3 years tertiary education in 2010, Entcap is entrepreneurship capital (average for 2008-2010), lnHHI is the natural logarithm of the Herfindah-Hirschman index of overall specialization (diversity), RV is the index of industry relatedness, and W0 is the constant.
We use the simultaneous estimation procedure, which means that the discriminant function is estimated based on the entire set of independent variables in a confirmatory approach to assess the overall performance. Technically, discriminant analysis estimates several functions if the number of groups is more than two, as in our case. The number of estimated functions is equal to the number of groups, less one. Each function will then represent a different dimension of discrimination (Hair Jr. et al., 2014). We employ the leave-one-out classification, which reduces optimistic bias due to small sample sizes (Cox & Wang, 2014).