Generative AI Sparks Innovation Across Payment Workflows
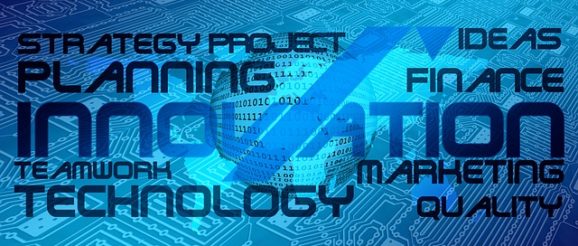
Anyone operating in the payments space already knows about artificial intelligence (AI). After all, the technology, along with its first cousin, machine learning (ML), together have been powering back-end systems and innovative products for years. “What is fundamentally changing, is that AI is redefining itself this year with generative AI,” Billtrust Senior Vice President, Data Analytics and AI Ahsan Shah tells PYMNTS. And this fundamental change is creating an equal transformation across the payments landscape. Shah emphasized the significance of large language models (LLMs) like OpenAI’s ChatGPT in revolutionizing the space by seamlessly integrating natural language processing (NLP) with conventional AI functionalities. This integration introduces a new player in the game, changing the dynamics of payments through language multimodalities. “Things like fraud detection, forecasting, anomaly detection and recommendations have existed for a very long time — but generative AI knows how to write, how to communicate, and how to generate content,” Shah said. “It’s a human-centric interface that is very much complimentary from a tech stack perspective.” As businesses strive for greater efficiency, accuracy, and security in their financial transactions, the role of generative AI is emerging as a catalyst for further innovation. Machine learning algorithms can analyze and extract relevant information from invoices, significantly reducing the time and resources spent on manual data entry, while automated invoicing systems can streamline workflows, minimize errors, and accelerate the entire invoicing lifecycle. From Predictive to Generative AI: Complementary Innovations The ease of use and versatility of AI make it a valuable addition to the tech stack, enhancing workflows and allowing businesses to concentrate on more strategic tasks. Still, for organizations thinking about rushing headfirst into this new ecosystem of opportunity, Shah cautions, “the models know about the world, but they don’t know about your business.” “Now we’re talking about security parameters, infrastructure. How do you feed data to a language model? How do you fine tune it, give it the context? There’s a lot of engineering,” he said. As for his recommendations? “Go after known problems and low-hanging fruit,” he said. Immediate wins for the applications of AI include customer support, sales, marketing and anomaly detection in payments. By streamlining processes and reducing manual overhead, businesses can leverage AI to enhance efficiency and decision-making. Still, technical gap, including engineering and data layers, is crucial, and organizations must align their workforce to become proficient in providing context to AI models, Shah said. “Finding the low-hanging fruit allows you to build the infrastructure and get confidence in proven wins. From there, you can expand into more complicated workflows,” he said. “There’s no reason to think that AI agents can’t become muti-agent ecosystems … you can have an AI in the payments universe talk to an AI in the collections world. We’re not there yet and there are a lot of guardrails to apply, but these cross-domain and cross-functional applications of AI could prove to have a compounding impact the higher up the stack you go.” Building the Technical Infrastructure for AI in Payments Shah emphasized the ubiquity of AI applications, describing the innovation as a “new electricity” that can be harnessed universally. While applications are broad, Shah anticipates the emergence of domain-specific AI applications, fine-tuned to specific use cases within the payments landscape. “What is new here is that now most companies will not be able to build their own foundational models. Only large companies like OpenAI, Anthropic, Google, etc. will do this. So, the data shared between those third parties is where you have to be very aware of what can be shared, what can’t be shared, and how those interact, because there’s a lot of power in those models,” he said. “What you want to do is find domain-specific applications that can be formed over time, like a payment-centric LLM,” Shah said, noting that “there are a lot of use cases where you don’t want to apply generative AI” or don’t need the technology for help. When it comes to these domain-specific AI systems, they will need to be trained on their own domain-specific data, which could represent a hurdle. “Way before AI existed, data fragmentation was always a problem,” Shah said. “Getting your data in order is always step one. And it’s important to be deliberate.” As for what the Billtrust leader sees the future holding, “Doing nothing is the worst option out there, but you also don’t want to be hasty in how you approach AI,” he said. “But because AI has such a human, conversational element to it, I think that it will catch on quickly. It will be hard to go to back manually submitting something or sifting through data when you have tried a 10 to a 100x process improvement based on natural language.” That’s part of why, as the payments landscape evolves, the integration of AI is not just a possibility, it’s an inevitability.