Hospital Predictive Tool Takes Advantage of Nursing Expertise | Healthcare Innovation
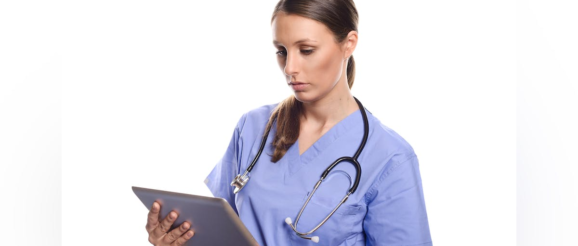
Several health systems, led by the Columbia University Irving Medical Center (CUIMC), are seeking to combine the expertise of nurses with artificial intelligence to create prediction models that can improve patient outcomes.
Nurse expertise includes the ability to pick up cues about patients’ health from subtle changes in behavior and appearance.
CONCERN (COmmunicating Narrative Concerns Entered by RNs) is a predictive tool that extracts nurses’ expert and knowledge-driven behaviors within patient health records and transforms them into observable data that support early prediction of organ failure or other critical conditions in hospitalized patients.
CUIMC is partnering with three hospital systems — Mass General Brigham (MA), Vanderbilt University Medical Center (TN), and Washington University School of Medicine/Barnes-Jewish Hospital (MO) — to test the effectiveness of the CONCERN implementation toolkit, developed to support large-scale adoption of the tool.
This initiative recently received funding from the American Nurses Foundation through the Reimagining Nursing Initiative.
“CONCERN shows what nurses already know: Our risk identification is not simply a subjective clinical hunch,” said Sarah Rossetti, assistant professor of biomedical informatics and nursing at Columbia, in a statement. “We’re demonstrating that nurses have objective, expert-based knowledge that drives their practice, and we’re positioning nurses as knowledge workers with tremendous value to the entire care team.”
Annually, more than 200,000 patients die in U.S. hospitals from cardiac arrest and over 130,000 patients’ deaths are attributed to sepsis. Many of these deaths could be preventable if patients who are at risk are detected earlier. Prior work from the CONCERN team found that nursing documentation within EHRs contains information that could contribute to early detection and treatment, but these data are not being analyzed and exposed by EHRs to clinicians to initiate interventions quickly enough to save patients.
The research team defined a new source of predictive data by analyzing the frequency and types of nursing documentation that indicated nurses’ increased surveillance and level of concern for a patient. These data documented in the 48 hours preceding a cardiac arrest and hospital mortality were predictive of the event. While clinicians strive to provide the best care, there is a systematic problem within hospital settings of non-optimal communication between nurses and doctors leading to delays in care for patients at risk. Well-designed and tested EHRs are able to trend data and support communication and decision making, but too often fall short of these goals and actually increase clinician cognitive load through fragmented information displays, “note bloat,” and information overload.
The aim of the CONCERN Initiative is to design and evaluate a SMART-on-FHIR app used across multiple large academic medical centers that exposes to physicians and nurses the predictive data source from nursing documentation to increase care team situational awareness of at-risk patients to decrease preventable adverse outcomes.