How to build a data architecture to drive innovation–today and tomorrow | McKinsey
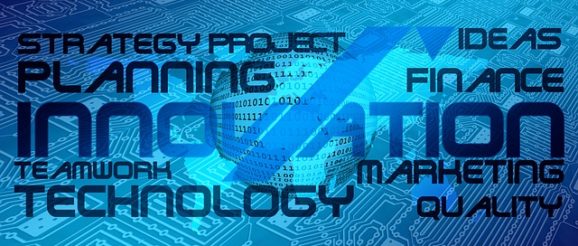
Downloadable Resources
Over the previous several years, organizations have actually had to move quickly to deploy new data technologies alongside tradition infrastructure to drive market-driven developments such as customized offers, real-time alerts, and predictive upkeep.
However, these technical additions– from data lakes to consumer analytics platforms to stream processing– have actually increased the complexity of data architectures tremendously, often considerably hindering an organization’s ongoing ability to provide new capabilities, keep existing facilities, and ensure the integrity of expert system ( AI) designs.
Existing market characteristics don’t permit such downturns. Leaders such as Amazon and Google have actually been utilizing technological innovations in AI to overthrow traditional organisation designs, needing laggards to reimagine elements of their own business to keep up. Cloud providers have actually released cutting-edge offerings, such as serverless information platforms that can be released quickly, allowing adopters to enjoy a faster time to market and greater agility. Analytics users are requiring more seamless tools, such as automated model-deployment platforms, so they can quicker utilize new designs. Many companies have embraced application programs user interfaces (APIs) to expose data from diverse systems to their data lakes and rapidly integrate insights directly into front-end applications. Now, as business navigate the unmatched humanitarian crisis brought on by the COVID-19 pandemic and get ready for the next normal, the requirement for flexibility and speed has actually only amplified.
For companies to build an one-upmanship– and even to keep parity, they will need a brand-new method to defining, executing, and integrating their data stacks, leveraging both cloud (beyond infrastructure as a service) and new principles and parts.
Six shifts to produce a game-changing information architecture
We have actually observed 6 fundamental shifts business are making to their data-architecture blueprints that allow more fast shipment of brand-new abilities and greatly streamline existing architectural techniques (exhibit). They touch nearly all data activities, consisting of acquisition, processing, storage, analysis, and exposure. Although organizations can execute some shifts while leaving their core technology stack intact, lots of require cautious re-architecting of the existing data platform and infrastructure, consisting of both tradition innovations and more recent innovations formerly bolted on.
when it comes to one United States bank, and 12 to 15 percent profit-margin growth when it comes to one oil and gas company ). We find these kinds of advantages can originate from any number of areas: IT cost savings, performance enhancements, minimized regulatory and operational threat, and the delivery of entirely brand-new abilities, services, and even entire companies. What crucial changes do companies require to consider? Would you like to get more information about McKinsey Digital? 1. From on-premise to cloud-based
data platforms Cloud is probably the most disruptive motorist of a radically brand-new data-architecture method, as it provides business a way to rapidly scale AI tools and abilities
for competitive benefit. Significant global cloud service providers such
as Amazon (with Amazon Web Services), Google( with the Google Cloud Platform), and Microsoft (with Microsoft Azure) have actually transformed the method organizations of all sizes source, release, and run information facilities, platforms, and applications at scale. One utility-services company, for instance, integrated a cloud-based information platform with container innovation, which holds microservices such as searching billing data or adding brand-new residential or commercial properties to the account, to modularize application abilities. This allowed the business to deploy new self-service capabilities to around 100,000 organisation consumers in days rather than months, deliver big amounts of real-time inventory and transaction data to end users for analytics, and reduce expenses by” buffering” deals in the cloud rather than on more costly on-premise tradition systems. Allowing principles and elements 2. From batch to real-time data processing The expenses of real-time data messaging and streaming abilities have reduced substantially, leading the way for mainstream usage. These technologies allow a host of new service applications: transport business, for
circumstances, can inform clients as their taxi approaches with accurate-to-the-second arrival forecasts; insurer can analyze real-time behavioral data from wise devices to individualize rates; and manufacturers can predict facilities concerns based on real-time sensor information. Real-time streaming functions, such as a membership system, allow information customers, consisting of information marts and data-driven employees, to register for “subjects “so they can obtain a constant feed of the deals they require. A typical data lake typically acts as the” brain” for such services, retaining all granular transactions. Enabliing concepts and parts 3.
From pre-integrated business services to modular, best-of-breed platforms To scale applications, companies often need to push well beyond the boundaries of tradition information environments from big option vendors. Many are now approaching a highly modular information architecture that uses best-of-breed and, often, open-source elements that can be replaced with new innovations as required without impacting other parts of the information architecture. The utility-services company discussed earlier is transitioning to this technique to
quickly deliver new, data-heavy digital services to millions of clients and to link cloud-based applications at scale. It uses accurate day-to-day views on customer energy consumption and real-time analytics insights comparing specific usage with peer groups. The company established an independent information layer that includes both industrial databases and open-source parts.
Data is synced with back-end systems by means of a proprietary business service bus, and microservices hosted in containers run organisation logic on the data. Allowing ideas and elements 4. From point-to-point to decoupled information gain access to Exposing information through APIs can ensure that direct access to see and modify information is limited and safe and secure, while concurrently offering faster, current access to typical information sets. This permits information to be quickly reused among teams, speeding up gain access to and enabling seamless collaboration among analytics teams so AI use cases can be developed more efficiently. One pharmaceutical company, for instance, is setting up an internal” information marketplace” for all staff members through
APIs to simplify and standardize access to
core data assets rather than counting on proprietary user interfaces. The company is gradually– over 18 months– moving its most valuable existing information feeds to an API-based structure and releasing an API management platform to expose the APIs to users. Allowing concepts and parts How primary data officers can browse the COVID-19 response and beyond Check out the post 5. From an enterprise warehouse to domain-based architecture Numerous data-architecture leaders have actually rotated from a central enterprise information lake towards “domain-driven” designs that can be customized and “suitable for purpose” to improve time to market of brand-new information products and services. With this method, while the data sets may still live on the exact same physical platform,” item owners” in each organisation domain( for instance, marketing, sales, manufacturing, and so on )are entrusted with arranging their information sets in an easily consumable way both for
users within their domain and for downstream information consumers in other business domains. This
a cautious balance to prevent ending up being fragmented and inefficient, but in return it can minimize the time invested up front on constructing brand-new information designs into the lake, frequently from months to simply days, and can be a simpler and more reliable choice when mirroring a federated business structure or sticking to regulatory restrictions on information mobility. One European telecoms supplier utilized a dispersed domain-based architecture so sales and operations staff might expose client, order, and billing information to data researchers for use in AI designs or directly to consumers by means of digital channels. Instead of developing one main information platform, the organization deployed logical platforms that are handled by item owners within the company’s sales and operations groups. Product owners are incentivized to promote using the information for analytics and are using digital channels as well as forums and hackathons to drive adoption. Allowing ideas and elements 6. From stiff data models towards versatile, extensible data schemas Predefined information designs from software vendors and exclusive data designs that serve particular business-intelligence needs are typically constructed in highly normalized schemas with stiff database tables and data components to reduce redundancy. While this approach stays the requirement for reporting and regulatory-focused usage cases, it likewise requires that organizations undergo lengthy advancement cycles and have strong system understanding when they want to incorporate brand-new information aspects or information sources, as any changes can affect information stability. To get higher flexibility and a powerful competitive edge when exploring data or supporting advanced analytics, business are progressing to” schema-light” approaches, utilizing denormalized data designs, which have less physical tables, to organize data for optimum performance. This
users within their domain and for downstream information consumers in other business domains. This
method uses a host of advantages: agile data exploration, higher flexibility in saving structured and disorganized data, and reduced complexity, as data leaders no longer need to present additional abstraction layers, such as several” signs up with” in between highly stabilized tables, to query relational data. Enabling ideas and parts How to get started Information innovations are developing quickly, making traditional efforts that specify and develop towards three-to-five-year target architectural states both dangerous and ineffective. Information and technology leaders will be finest served by instituting practices that allow them to rapidly examine and deploy new innovations so they can rapidly adjust. Four practices are important here: As information, analytics, and AI become more ingrained in the daily operations at the majority of companies, it’s clear that a radically various approach to data architecture is required to develop and grow the data-centric business. Those data and technology leaders who welcome this brand-new approach will much better position their companies to be agile, resilient, and competitive for whatever lies ahead. About the author( s). Josh Gottlieb is a practice manager in McKinsey’s Houston office, Jorge Machado is a partner
in the New York office, and Matthias Roggendorf
is a partner in
the Berlin workplace. The authors want to thank Antonio Castro, Sameer Kohli, Aziz Shaikh, and Nikhil Srinidhi for their contributions to this short article.