How to Hire a Productive, Diverse Team of Data Scientists | Articles | Chief Data Officer | Innovation Enterprise
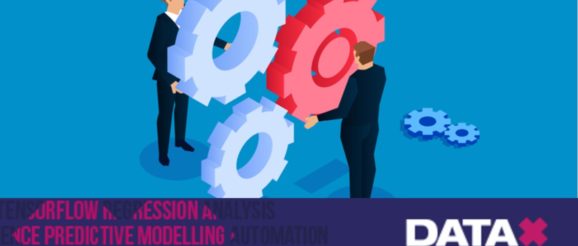
Haftan Eckholdt, chief data officer and chief science officer of Understood.org, has built data science teams at Plated, Audible, and AIG. He is also a former assistant professor of neuroscience and neurology at Albert Einstein College of Medicine.
Understood.org, Eckholdt’s current company, helps families of differently-abled children. Its Workplace Initiative has helped thousands of people with all types of disabilities find meaningful employment at inclusive companies.
Below is an excerpt from a presentation Eckholdt gave at DATAx New York last November. He explains in detail his process of hiring a data science team at Understood.org in December 2018, when he first posted the job descriptions, and early 2019.
The job description
“I build job descriptions to be welcoming and inviting to lots of different people. Most job descriptions I find have barriers that are completely unnecessary. I don’t care what language you code in. I just want to know that you like to code. You have a language. If you can express a preference, fantastic. I don’t care what language it is. And I don’t need to put languages in there as this barrier to ‘Oh, you must do x, y and z.’ Because there are a million people who would thrive on this team but never apply because they say ‘Oh, well, I don’t do HTML.’ Why would somebody put HTML in there? What’s the deal? You don’t need that stuff, so take it out. It’s to be inviting and welcoming. Let people come and try.”
“The goal was to hire six for the data science team. We got 450 applications. From those 450 applications I read every one of them and I found 90 that I wanted to then do phone screens with. [I use] a very simple scoring process that tells me about interests, experience, goals, disciplines, degrees. I’m not necessarily going to screen people in or out of the funnel based on degrees. I know that I want to see a mix of bachelor’s, doctorate’s, and master’s, that’s all. But I may actually rank and score people in the funnel based on their perceived interests, the relevance and the experience of their discipline. It’s a very simple way of scoring résumés.”
Phone screening
“I held 90 phone screens. Ninety phone screens, half an hour, not video. I always do the first encounter by phone. I find videos to be very [disruptive] to my [scoring] process and I think they create a lot of unnecessary anxiety in candidates. I need to get people to relax during an interview. If I can’t get somebody to relax I cannot assess their skills and abilities. If they’re terrified I can’t figure out if they can do the job. So, I want to get them to relax. All I’m interested in are skills. So I ask them questions: How did you find the ad? Are you actively applying? Is there a pattern to where you’re applying? What’s the last piece of code you wrote? Tell me all about it. That can go on and on and on forever. And then I always say the last thing — what questions do you have for me?”
“I score that phone screen exclusively on the number of questions they ask me, simplest metadata point in the world. No questions? Thank you very much, we’re done. Sorry. A handful of questions? that’s OK. Not great. You’re probably still nervous and it might be your first job. Ten, 20 questions, won’t stop, crazy. Curiosity is what we really look for in data scientists.”
Case studies
“For the case study [that I assign those who make it to the next phase], I basically tell them to go to Kaggle. I give them a brief description of the classes of problems that are suitable. And I invite them to pick a data set and then do something with it and then explain it to me. This resulted in 30 technical screens. I score the technical screens that are turned in based on their problem search, the selection of the data set, the problem description, their approach to the problem, and their code. Very easy [for me] to score.”
Second interview
“I then do hold a video interview to talk to them about their work. I need to understand their work. That’s it. They can get it wrong. I don’t care about that. And I even tell them that. I want to know what they were thinking and what decisions they made and why. This led to 15 interviews.”
“At this point in time what I needed to do was involve stakeholders from around the organization. I was the only data scientist in the building. And I’m now contaminated … I know these people too well. I’m useless.”
“So, I take non-data-scientists from different parts of the organization and I train them briefly. I say ask candidates what they do, and see how long it takes you to understand it. Explain what you do and see how long it takes them to understand you. And invite them to symbolically solve one of your problems with or without a whiteboard and see if comes to a rational conclusion. And you can score [the candidates] based on those things. It’s very easy for a non-technical person to now have a rich conversation with a data science candidate and figure out if they want to bring them on board.“
The results
“Those 15 interviews [were cut down] to 9 candidates for the reference stage. At this point, I call it chess. Because I’m trying to find the 6 optimal people, personalities, backgrounds to [join] the team. We offered 6 people those roles. … And 5 accepted. The one who did not accept had lags everywhere. They were the longest to respond to me in every moment of the chain. So, I knew there was hesitation throughout this process. By the way, we now have 7 on the team. Great dispersion of educational backgrounds. They use all different pronouns and [there is a] good distribution of people of color on the team. And it is the most balanced team in the organization. And I think everybody’s happy to be there and they’re productive.”