How to Use Predictive Analytics to Remove the Guesswork for the Future – Innovation & Tech Today
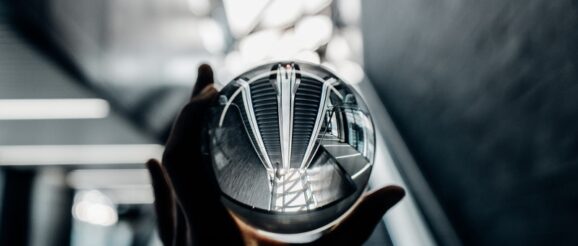
By Guest Author Srinivas Kunta
In a data-driven world, consumers have high expectations from companies like Amazon, Netflix, and Spotify to know their preferences to determine exactly what they want to buy or watch next. The ability to deliver on these expectations stems from what is known as predictive analytics, a powerful tool that uses data and machine learning algorithms to predict consumer behavior. A recent study released by Reports Insights reveals that the market generated a revenue of $13.5 billion in 2022 and is anticipated to achieve $44.3 billion by 2030, displaying a compound annual growth rate (CAGR) of 18.5 percent. That’s a huge leap in less than a decade and that growth is likely to continue. Predictive analytics uses historical data, artificial intelligence (AI), and machine learning (ML) to forecast future outcomes, behavior, and trends. Predictive analytics is a powerful way of analyzing data, giving decision-makers the right information to make informed decisions. This creates a path to eliminate guesswork and helps to build better strategies to reach customers and other stakeholders. That’s why data analysts need to identify the right tools to streamline and transform data from modeling to analytics.
Predictive analytics today: Why it is essential for businesses and individuals alike
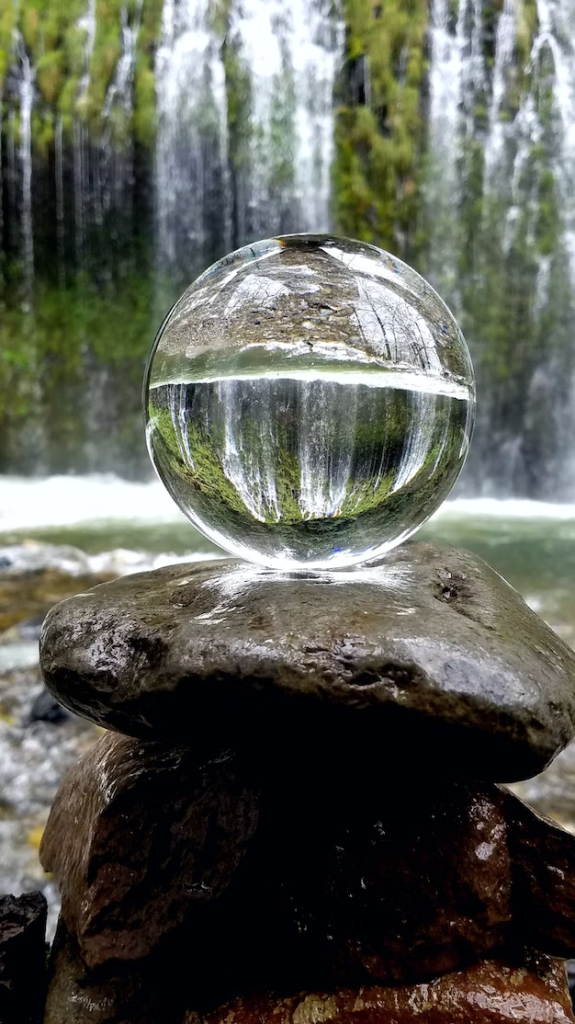
A decade ago, the use of data was growing, but consumers and companies were just starting to tap into its potential. Today, data is touted as the new oil, and claims of being in a data-driven world are appearing in headlines regularly. As a result, businesses now have access to vast amounts of data. Still, raw data means nothing without a strategy to collect, organize, analyze, and visualize what that data represents to draw actionable insights. With the proper foundation, tools, and action plan, it’s possible to leverage predictive analytics to visualize patterns and trends to help organizations and individuals predict future outcomes. As a result, companies can identify cost-saving opportunities, eliminate or reduce waste, and increase efficiency and productivity. It provides valuable information to make better business decisions and mitigate risks. The effect of predictive analytics doesn’t end with business-facing benefits. It impacts consumers, too, just like those streaming algorithms that recommend the next watch list. Predictive analytics can help consumers make better purchase decisions, receive better service, and improve their decision-making.
Technologies such as ML, AI, and cloud computing have made it easier and more affordable to perform predictive analytics across various industries and individual use. Predictive analytics can impact an organization’s sales and marketing, help business campaigns target the right customer segments, and navigate market research by identifying current trends and predicting future ones. It can improve supply chain optimization by forecasting demand and improving logistics such as transportation delivery times by optimizing routes or cargo. Manufacturing can predict demand and optimize production schedules, improving productivity and efficiency. At the same time, predictive analytics can anticipate machinery failure, reducing costs and improving employee safety.
Predictive analytics impact individuals through weather and natural disaster forecasting. These impacts can be individual-facing, but typically trickle down from larger organizations. For example, a utility provider can share data with their customers to recommend optimized, energy-saving options to assist customers in making better decisions about their energy consumption or budget.
Four critical pillars of predictive analytics (and the technologies that drive them)
For predictive analytics to be successful, it’s essential to look at what has happened, what is happening, what might happen, and what is likely to happen. Only then are relevant insights gleaned that result in action. A compelling discussion of one type of analytics includes information on all four pillars: descriptive, diagnostic, predictive, and prescriptive.
Descriptive analytics examines historical data for patterns and trends. This is where the central question, “What happened?” is answered. This historical data can be organized and visualized in charts, graphs, and other mapping technologies to identify and compare trends and patterns. In this stage, data is organized, described, or named so it can be grouped and identified. For predictive analytics to succeed, gathering data from many sources, including statics, ML, and data mining are vital.
Diagnostic analytics moves a step past descriptive analytics. Instead, it acknowledges those identified trends or patterns from the past and then evaluates and explains why they occurred the way they did. This stage looks for the cause and effect to identify probabilities, outliers, and the relationships between specific pieces of the data picture.
Predictive analytics takes what was learned from diagnostic analytics using historical data to predict likely outcomes and make educated forecasts about specific scenarios. Uncovering realistic future probabilities is impossible without a thorough commitment to descriptive and diagnostic analytics first. Predictive analytics typically rely on proven models that help predict behaviors, such as cluster models, which separate data into similar groups based on shared features, or decision trees, to identify the choices available based on specific variables.
Prescriptive analytics is the next rung in the analytics ladder. It uses the previous three areas of data collected to evaluate those possible future outcomes and recommend or narrow in on the best option. This stage might use ML tools and algorithms to identify those recommendations. Prescriptive analytics is helpful when a customer requires guidance while making a purchase decision or when offered a broad spectrum of options, such as when using streaming services. For organizations, prescriptive analytics can help mitigate risks by providing possible actions that leadership can visualize based on market trends.
Predictive analytics is here to stay and grow.
Predictive analytics is only going to become more relevant in people’s daily activities. That said, the prevalence might be hard to identify as technologies and uses advance and become more seamlessly intertwined with day-to-day life. Predictive analytics is a powerful tool that can benefit both businesses and consumers. Businesses that invest in predictive analytics now will gain a competitive edge while their impacts can benefit individuals in more ways than they may realize. By using data and ML algorithms to predict market fluctuations, consumer behavior, and future circumstances and outcomes, companies can make smarter decisions and improve customer experiences while promoting overall business growth. It’s important for consumers to be aware of how predictive analytics is used, and leveraged in their personal lives. The rapid growth of AI and related technologies will accelerate predictive analytics’ accuracy and potential uses. With a better understanding of predictive analytics, data can support consumers to make informed purchase decisions and enjoy a more personalized customer experience.
About the Author
Srinivas Kunta is an IT solutions architect with more than 15 years of experience in SAP. He is a subject matter expert in SAP Basis, integrations, and cloud solutions. Srinivas holds a bachelor’s degree in computer applications from Osmania University, India, and can be reached at ksrinu06 at gmail.com.