Human behaviour, NPI and mobility reduction effects on COVID-19 transmission in different countries of the world | BMC Public Health | Full Text
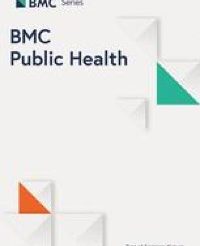
Quantifying the effective reproduction number using incidence data
To quantify the overall relative status of the pandemic at the various locations, we show in Fig. 3 the evolution of Reff−data in each region using a heatmap plot and depicting values of Reff−data(t)∈[0,4].
The effective reproduction numbers from incidence data plotted per range of values (Reff−data∈{[0,0.5],[0.5−1],…,[2.5−3]}) from February 15 to December 31, 2020. Lightest color patches signify biggest weekly reductions in values of Reff−data among other weeks in each region
The effective reproduction numbers from incidence data plotted per range of values (Reff−data∈{[0,0.5],[0.5−1],…,[2.5−3]}) from February 15 to December 31, 2020. Lightest color patches signify biggest weekly reductions in values of Reff−data among other weeks in each region
The color gradient signifies lowest values of the effective reproduction numbers in lightest color zones, and highest values in darkest zones. Figure 3 showcases a comparison of the Reff−data between locations. We can immediately see the effect of the reduction in Reff−data, across the board, in all countries between March and April, and the rest of the year. It is clear that the initial lockdowns and mobility reduction allowed all countries to drop their Reff−data to around 1, and interestingly most remained around this value for the rest of 2020. We include here (Table 5) the approximate weekly average value of Reff−data at each location in mid-to -end of April 2020..
Qualitatively, we can understand why Reff−data has generally fluctuated in the vicinity of 1: On the one hand, rapid growth of incidence causes alarm at both the policymaker and individual level, and typically leads to increased NPI directives as well as compliance. On the other hand, because these measures are hard to maintain and economically taxing, NPIs are generally relaxed not long after incidence starts to drop. In other words, it is the human behavioural element that we do not model here, but that we glimpse.
Quantifying the effective reproduction numbers accounting for mobility data
We observe that the initial sharp reduction in contact rate due to mobility happened in the middle of March in most regions under study, when initial lockdown was in effect, except in Sweden. The contact rate gradually increased from early May when partial reopening was implemented by governments. The results of these measures are noticeable in the Figs. 4 and 7 upper right panels.
Average weekly contact rates reflecting mobility changes from the Google Mobility index in the Home, work and other activities respect to the baseline (pre-lockdown), from Feb 15 to Dec 31, 2020. Baseline rates were computed from Google mobility reports over a 6 week interval of January-February 2020
Average weekly contact rates reflecting mobility changes from the Google Mobility index in the Home, work and other activities respect to the baseline (pre-lockdown), from Feb 15 to Dec 31, 2020. Baseline rates were computed from Google mobility reports over a 6 week interval of January-February 2020
The effect of mobility restrictions throughout 2020 for each geographical location under study is presented in Fig. 5 below. We plot the theoretically estimate Reff−mobile numbers together with the Reff−data effective numbers at each location. Overall, under Google mobility reductions using formula 7, the mobility reduction effect is not enough, on its own, to explain the values Reff−data. This is not surprising, as each location had a varying combination of NPI measures.
Effective reproduction numbers from the mobility data influenced by contact rate. In each panel, the red vertical dash line represents the lockdown measures date and the pink curve shows the weekly effective reproduction numbers from the mobility affected by average contact rate in that region
Effective reproduction numbers from the mobility data influenced by contact rate. In each panel, the red vertical dash line represents the lockdown measures date and the pink curve shows the weekly effective reproduction numbers from the mobility affected by average contact rate in that region
Figures 5 (and Fig. 6 in an ensemble view) reveal the reduction in mobility in each region. It seems that the mobility decreased 51% and 54% in Nepal and Italy with respect to the baseline in the first and second weeks of April 2020, respectively. The mobility fell about 20% percent in Indonesia and Sweden. Large-scale social restrictions (sometimes called partial lockdown) were introduced by the Indonesian government in place of nationwide lockdown at the end of March 2020 while the Swedish authorities imposed some restrictions on gatherings in late November.
Reduction in Average weekly contact rates reflecting mobility changes from the Google Mobility index throughout 2020. The week that reduction happened is highlighted by “month/day” in each bar
Reduction in Average weekly contact rates reflecting mobility changes from the Google Mobility index throughout 2020. The week that reduction happened is highlighted by “month/day” in each bar
A comparison of the effective reproduction number as obtained from the incidence data (yellow curves), to our theoretical estimate from Section Quantifying the effective reproduction number using incidence data (light pink curves) using mobility data. Beyond the date of mask mandate enactment in each region, we show the theoretically-estimated incidence both with (dark pink) and without (light pink) the added effect of mask-wearing
Human behaviour and its impact on disease transmission
The effects of mask mandates and mask compliance on further reduction of the effective reproduction numbers
We will be looking at two main tools that populations can use to control the pandemic: social distancingand mask wearing. In our framework here, each one of these directly influences the transmission rate β. Denoting by maskeff the efficacy of an average mask at preventing transmission and by compliancem the compliance with mask wearing let us imagine a meaningful contact between an infected and a susceptible individual: If an individual wears a mask and maskeff=0.3, then he/she has an increased protection against transmission. If the individual complies with mask wearing 50% of the time, then their protection due to mask wearing is, on average maskeff·compliancem=0.15 which implies that the per-contact transmission probability p will decrease with mask wearing:
Recalling that the level of mask-wearing and social distancing both change over time, we estimate that
Let us consider only mask-wearing for the time being. In this paper we use a value of maskeff=50%, as averaged based on estimates in [56] (see a more detailed discussion in Additional file 1: Appendix. and the values of mask compliance from IHME (details of data sources in Data sources section above and Additional file 1: Appendix.). Using (8) in the estimate (7) leads to:
with mask compliance data from IHME (see Additional file 1: Appendix) and contactav(t) data that is explained in section (Time-series of effective reproduction numbers accounting for mobility data and projected contact rates).
Figure 7 shows the effective reproduction numbers as obtained from the incidence data (yellow curves) from Fig. 2, together with our theoretical estimates from Fig. 5 (light pink curves) using mobility data, to which now we add a new theoretically estimated Reff−mobilemask number reflecting mask wearing data and formula 9 above. The red and black dashed lines in each panel show the lockdown and mask-wearing mandate dates, respectively, in each region as publicly available.
From 7 we observe that the theoretically-estimated curve using both mobility reductions and mask wearing data (which we will now denote by Reff−mobilemask) is accounting for more of the reduction in transmission than the estimated Reff−mobile curves of Fig. 5. Evidently, in some regions (Ontario and Argentina) we observe what it looks like an over-reduction in the values of Reff−mobilemask as compared to Reff−data, while in other countries, most notably Sweden, we notice essentially no contribution in further reduction from Reff−mobile to Reff−mobilemask. In Sweden’s case, this is not surprising, as the mask wearing levels in the IHME data we used hover between 1%−2% throughout 2020. Last but not least, this assumes a values of mask efficacy maskeff=50%. If this value is decreased, the Reff−mobilemask curves will shift upwards, i.e. the reduction of Reff−mobile due to mask wearing will be smaller.
Effects of other social distancing measures on further reduction of disease spread
As we highlighted in previous section, a host of other NPI’s have been employed, at different strengths, across the regions. While it is clear from our investigations thus far that the mobility reduction (as reflected in Google mobility data), along with mask wearing and mask compliance helped tremendously in the de-escalation of the Reff curves, we wish to further analyze the data to extract more information on the strength of other NPI’s. Let us denote by complianceoNPI the compliance level in the local population with other NPI measures (by other we mean other than mobility and mask wearing). With this in mind, an average individual in a local population is protected over the course of their contacts proportional to what fraction of the time they also comply with other NPI measures:
which then means that
This means that to quantify the effects of other NPI measures per region, we further look at the ratio: \({\frac {R_{eff-data(t)}}{R_{eff-mobilmask(t)}}}\). We present these plots in Fig. 8 below. Clearly when the ratio is less than 1, then the reduction in the Reff that we quantified from observed data is stronger than the reduction of Reff based on mobility and mask wearing and viceversa. Specifically we obtain the following:
In the other case, under our assumptions, we get
In cases where complianceoNPI<0 we interpret it to mean that perhaps the assumption of 50% efficacy in all regions may have been too optimistic..
Effect of other NPI measures (e.g. 6 feet (2m) social distancing, hand washing, etc.) [ Reff−data/Reff−mobile]. Mandatory masks were introduced at dates represented by the vertical dashed line in each panel
One remark is worth to be made at this point: if one decreases the mask efficacy maskeff to an average of 40%, respectively even lower to 30% (as in [56]), then the Reff−mobilemask curves of Fig. 5 would scale equivalently upward by a constant, thus implying that the mask wearing effect is less effective and therefore that the ratio \(\frac {R_{eff-data}}{R_{eff-mobile}}\) would be less than 1 (i.e., complianceoNPI(t)>0) for most (respectively all) regions.
Results in Sweden in particular stand out (see Fig. 9). They indicate that other NPI measures were extremely effective in reducing the transmission rate of disease, in the absence of mandated lockdown periods and nearly 0 mask wearing. In the case of Sweden for instance (upper panel of Fig. 9), complianceoNPI(t) is large starting in April 2020. Here complianceoNPI(t)>0 at and over 50% in Sweden. In case of Indonesia, we estimate that complianceoNPI(t) at and over 30-40% in Indonesia. Both these ranges assume an average of maskeff=50% over the time period May-December 2020.
Effect of other NPI measures in Sweden and Indonesia, [ Reff−data/Reff−mobile]. Mandatory masks were never introduced in Sweden until November 2020, but they are present earlier in Indonesia. Nationwide lockdown was never used in these countries in 2020
Effect of other NPI measures in Sweden and Indonesia, [ Reff−data/Reff−mobile]. Mandatory masks were never introduced in Sweden until November 2020, but they are present earlier in Indonesia. Nationwide lockdown was never used in these countries in 2020
At the other end of the spectrum, Ontario (upper left corner panel in Fig. 8) seems to display a ratio of \(\frac {R_{eff-data}}{R_{eff-mobile}}\approx 1\) after the mask mandate date (vertical black dash line). Moreover, we have periods of time where the ration is larger than 1 somewhat, so then complianceoNPI might have been negative (in the case where maskeff=50%). This seems to indicate that, under our assumptions, the mobility reductions captured via Google indexes and the mask compliance levels have essentially captured the full picture of the pandemic evolution in this region. Similar trends can be seen in Argentina and Nepal (see Figs. 8 and 10).
Effect of other NPI measures in Ontario and Argentina, [ Reff−data/Reff−mobile]. Mandatory masks were introduced at dates represented by the vertical dashed line in each panel
Effect of other NPI measures in Ontario and Argentina, [ Reff−data/Reff−mobile]. Mandatory masks were introduced at dates represented by the vertical dashed line in each panel
Further investigations into mask efficacy and its impact on transmission
In the sections above, we have considered a fixed value of maskeff=50% across all regions, and we have commented on how a decrease of this value may affect the reductions of Reff−mobile values. From formula 7 we have
for each region. Here, we perform a maximum-likelihood estimate of maskeff for each region, such that the fit of Reff−mobile to Reff−data is optimized. In other words, if we considered NPIs to consist only of mask-wearing, what mask efficacy would best reproduce the observed time series of effective reproduction number in a given region? We present our results in a new plot with a small Table 6:
The graphs of the new Reff−mobilemask time series are presented in Fig. 11 (dark pink curves). Here we see again that Sweden stands out simply because mask wearing was not a policy the population had adopted in 2020; even at a 100% efficacy, an adoption of 1-2% has negligible effect. Thus other NPI factors must have been in play.
Maximum-likelihood estimate of maskeff for each region so that the fit of Reff−mobile to Reff−data is optimized. Curves in dark pink color are the new Reff−mobilemask estimates, where for each country we use the deduced value of maskeff listed
Maximum-likelihood estimate of maskeff for each region so that the fit of Reff−mobile to Reff−data is optimized. Curves in dark pink color are the new Reff−mobilemask estimates, where for each country we use the deduced value of maskeff listed
Among all the other countries, we see that high levels of mask wearing (for instance in Ontario, and similarly Romania and Argentina) correlates to realistic mask efficacy values (in [56] a realistic expected protection from the mostly cloth-type masks available widely in 2020 is between 30% to 50%) In regions such as Florida, Italy, Indonesia, South Africa, Ghana and Brazil the lower mask wearing levels would have needed to be compensated by higher mask efficacy levels. Since such levels of mask efficacy were not possible in 2020 for an average individual in any of these countries, then other NPI factors had to have been in play.
This analysis furthers the importance of our analysis in the last subsection (Subsection Effects of other social distancing measures on further reduction of disease spread) where we manage to quantify the effect of other NPI factors (oNPI) in reductions of transmission in each of the 12 regions.