Industrial Demand and Innovation: An Application of Binomial Regression Model to Project Statistics of NSFC of China
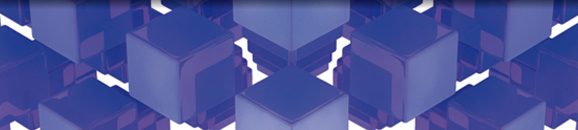
The carbon emission reduction potential of photothermal utilization of solar energy is outstanding, but the breakthrough of technology is closely related to the management of innovation and research in higher educational institutions. What factors affect such relationship is discussed in this paper. Based on the photothermal technology project data released by the National Natural Science Foundation of China (NSFC) and China education yearbook, this paper analyzes the variable relationship between the output of solar thermal technology innovation and industrial demand. Specifically, the negative binomial regression was constructed based on the technological project approval data. The results show that the input of scientific research resources has a key influence on the development of solar thermal technology, the establishment of technological projects or the subsequent patent application derived from technological projects. The results also show that the demand for photothermal technology and green innovation complement each other.
1. Introduction
With the increasing shortage of global fossil energy supply and increasingly prominent environmental problems, solar energy, as typically clean and renewable energy, has attracted more and more attention [1]. The development and application of solar thermal utilization technology are gradually becoming a hot spot in the energy industry [2]. The main thermal energy of the earth comes from the sun, and the total energy of solar radiation received by the earth’s surface every year is about 1018 kWh, which is about ten times the sum of the energy generated by all fossil fuels on the earth. Solar thermal power generation technology generates electricity by gathering solar energy and converting photoelectric energy by physical means, which does little harm to the environment. The CO2 emission of a solar thermal power plant in the whole life cycle are only 13–19 g/kWh [3]. At the same time, the use of inexhaustible solar energy resources for power generation can effectively save fossil energy and alleviate the energy crisis. Due to its technical characteristics, solar thermal power generation can be equipped with heat storage devices, and fuels like natural gas can be used as an ancillary power generation system [4]. The solar photothermal power generation system with heat storage devices and an ancillary power generation system can solve the problem of unstable unit output caused by the intermittency of light resources, ensure the operational continuity of the power generation system, significantly smooth the output curve, and better match with the load. Compared with photovoltaic power generation, the solar photothermal power generation system can reduce the cost of ancillary services such as the operating reserve and has better grid friendliness [5]. Given these advantages of solar photothermal power generation technology, it is of great significance to promote the rapid development of the solar photothermal power generation industry for the environment, resources, and economy of all countries in the world. At present, the utilization of solar thermal resources is still in the form of photothermal power generation mainly based on the Rankine cycle principle, which makes it difficult to further improve the cycle efficiency of the solar thermal power plant. At the same time, the power generation system based on this cycle mode will aggravate the pressure of water resources in areas with abundant solar energy resources but relatively short of water supply to a certain extent. Therefore, how to use this rich and pollution-free clean energy efficiently and safely is one of the main directions of current research.
Basic research is the source of the national innovation system and the most critical part to promote applications. The scientific research results of colleges and universities have a promoting effect on the development of the industry. Li et al. [6] pointed out that China’s fund in basic research increased from USD 880 million in 2002 to USD 14.57 billion in 2017, with an average annual growth rate of 17.6%, but its investment intensity and proportion in total scientific research expenditure are still lower than those of developed countries. The support for the development of downstream industries is weak, and the scientific research fund the management system still needs to be further improved. This paper intends to discuss the relationship between scientific research and China’s industrial demand and green sustainable innovation in the project approval from the perspective of university fund project information. To understand the basic research fund, by applying the binomial regression model, this paper evaluates the efficiency of the National Natural Science Foundation of China in the process of stimulating the basic knowledge innovation of universities (upstream innovation carriers) to promote the transformation of application achievements of enterprises (downstream innovation carriers) and makes a specific description of its transformation channels. As for data, the project data from the National Natural Science Foundation of China from 2018 to 2019, has been used as the research object, taking the number of projects in the photothermal industry as the explained variable, and education and scientific research in various provinces as explanatory variables to explore the impact of various variables.
Compared with the literature, this study provides empirical evidence for the dissemination and transformation of tacit knowledge among innovation entities. For tacit knowledge that is difficult to encode or record, industry-university-research cooperation has always been considered as the most effective channel for its dissemination from upstream to downstream. However, due to data limitations, relevant studies have stopped at qualitative analysis, and few works of literature have carried out quantitative mathematical analysis [7, 8]. The structure of this paper is as follows: the second section is the literature discussion; the third part is the data and empirical results; and finally comes the summary of this paper.
2. Literature Review
In recent years, with the continuous development and innovation of photothermal technology, policy research on promoting the development of photothermal technology has also been accumulating. For example, Zongxian et al. [9] believe that the rapid development of China’s solar photothermal industry is mainly attributed to the fact that the price of solar photothermal, a new energy source, is lower than that of traditional energy sources such as electricity and natural gas, which can fully meet the needs of residents. It is further found that the rising price of traditional energy sources, the improvement of residents’ living conditions and behavior, large-scale housing construction, and Chinese society’s emphasizing on environmental protection will further promote the growth of the solar thermal industry [10, 11]. As for investment costs, like all emerging industries, the investment cost of photothermal technology is relatively high due to the lack of large-scale production in the early stage of development. The photothermal technology of the United States, Spain, and other countries is relatively mature, and the investment cost is lower than that in the early stage of industrial development [12]. References [13, 14] used the system advisor model (SAM) simulation software to estimate the current and future costs of trough power plants and molten salt tower power plants in the U.S. power market. The prediction results show that the levelized cost of energy (LCOE) of the two power generation technologies will be reduced to 11 cents/kWh, which makes the cost of photothermal power generation competitive compared with the benchmark electricity price of California. Li et al. [15] established a comprehensive indicator system of solar thermal power generation technology from five aspects: development conditions, power generation technology, the economics of power generation, grid connection requirements, and policy environment; and comprehensively evaluated the current situation of solar thermal power generation in China. The evaluation results show that solar thermal power generation has certain advantages in terms of grid connection requirements, power generation technology, and development conditions, but not in terms of advantages in the policy environment and the economics of power generation [16]. Given the development environment of China’s solar thermal industry, Yu and Schwieter [17] pointed out that the development of China’s solar thermal industry is more business-opportunity oriented than government-environment oriented. Similarly, Xu et al. [18] believe that the development of the solar photothermal industry requires a reasonable allocation of corresponding R & D funds and a favorable policy environment. The discussion on fund research in Europe and America started much earlier than in China. The national innovation system theory proposed by Freeman provides a classic analytical framework for people to understand the process of transforming basic research funding into application innovations [19]. The authors point out that government-promoting research funding involves the main entities of the government, universities, scientific research institutions, enterprises, etc. They divide work and cooperate to capitalize knowledge, with knowledge creation, dissemination, and transformation as the mainline. In this process, universities and scientific research institutions are upstream of the knowledge dissemination chain and mainly carry out basic research. Enterprises are located downstream of the knowledge dissemination chain to develop and utilize the research results generated upstream, and finally, transform them into new products and technologies that meet the market demand [20]. In actual economic activities, due to the externality of knowledge, the scientific research achievements based on research investment will reduce the R & D costs and R & D investment returns of enterprises. Hence, the motivation of independent R & D of enterprises will decline [21]. At the same time, due to the reduction of market access cost and the increase in market competition, the profit margin of the enterprise fund in application R & D is reduced. Therefore, the final impact of basic research funding on enterprise innovation remains to be empirically studied and tested.
The frequent interaction between various main innovation entities in formal and informal ways can promote the dissemination and transformation of knowledge and improve the efficiency of the national innovation system [22]. In terms of the transmission channel of knowledge from upstream to downstream, the existing literature mostly conducts qualitative research, such as case analysis, to provide evidence. Explicit knowledge in the form of words or patents can be transferred to enterprises through learning or transfer [23]. In addition, a large amount of tacit knowledge depends heavily on forms such as the cooperation between schools and enterprises, face-to-face communication, and the flow of scientific researchers to disseminate and transform [24, 25].
Combing the above literature, it can be seen that the discussions on scientific research are more focused on path orientation, qualitative analysis, etc., and there are relatively few discussions on quantification. The relevant research literature on China is even more scarce. Based on this, the statistics of the National Natural Science Foundation of China from 2018 to 2019, are taken as the research object, the number of projects in the photothermal industry as the explained variable, education and scientific research, etc., in various provinces as explanatory variables to explore the impact of each variable.
3. Data and Empirical Results
As mentioned above, this paper considers the research on solar thermal in China’s scientific research development as the object. Because the situation of scientific research in colleges and universities may be affected by the number of local schools, the applicant’s achievement requirements at the time of project application, the number of scientific researchers in their region, the fund for scientific research, and intellectual investment, based on the project data of the National Natural Science Foundation of China from 2018 to 2019, this study analyzes the keywords or titles related to solar thermal in the project during the year. Based on the empirical data, the number of projects approved by the National Nature Fund and the data of China’s education statistics yearbook are sorted out. From the 2018–2019 China’s education statistics yearbook, we have sorted out the number of schools, the number of teaching staff with a Ph.D. degree or a Master’s degree, the number of professors or associate professors, the number of graduates and undergraduates, and the school’s teaching and scientific research assets; and listed their abbreviations into Table 1. In the statistics of the projects approved by the National Natural Science Foundation of China, the statistics of photothermal projects and projects approved in each province are compiled. Table 2 sorts out the descriptive statistics of basic variables. It can be seen from Table 2 that among the faculty members in China’s current colleges and universities, the number of teaching staff with a master’s degree is higher than that of a Ph.D. degree, and the overall faculty members are made up mostly of associate professors. In addition, the number of undergraduates is about 6-7 times that of graduates.
|
|
Description | Symbols |
|
|
The total number of approved projects in the province | Ni |
The number of teaching staff with a Ph.D. degree | Pa |
The number of teaching staff with a master’s degree | Pb |
The number of professors | Pc |
The number of associate professors | Pd |
The number of undergraduate students | Pe |
The number of graduate students | Pf |
The teaching and research asset of a school (10,000 RMB) | Ar |
Logarithm of the number of people logarithm of | Pa |
Logarithm of the numbers of professors and associate professors logarithm of | Ph + Pd |
Logarithm of the number of graduate students logarithm of | |
Logarithm of the number of undergraduates logarithm of | Pm |
Logarithm of the teaching, science and research asset of a school logarithm of | Ar |
|
|
||||
Variable name | Mean | Std. Deviation | Minimum | Maximum |
|
||||
Ni | 30.17 | 20.532 | 1 | 72 |
Pa | 17678 | 10757 | 312 | 38109 |
Pb | 23323 | 8963 | 1052 | 36928 |
Pc | 9420 | 4449 | 741 | 17534 |
Pd | 20696 | 7661 | 1355 | 34764 |
Pe | 773045 | 291920 | 42248 | 1236156 |
Pf | 110176 | 87839 | 3035 | 299562 |
Ar | 2018069 | 1265583 | 64211 | 4592204 |
|
Table 3 shows the correlation coefficient matrix of each variable. The statistical results show that the correlation among the variables is mainly between −0.400 and 0.800. First, the positive correlation between the number of teaching staff with a Ph. D. degree, the number of graduate students in colleges and universities, and the total number of projects the highest, which is 0.945 and 0.947, respectively. The number of professors in colleges and universities also has an important impact, and the correlation coefficient is 0.907. Since scientific research institutions need to list the structure of scientific research teams when applying for projects, the requirements for professors and associate professors in scientific research teams are relatively important. The statistical results reflect the relationship between professors and project establishment.
|
|||||||||
(1) | (2) | (3) | (4) | (5) | (6) | (7) | (8) | ||
|
|||||||||
(1) | Ni | 1.000 | |||||||
(2) | Pa | 0.945 | 1.000 | ||||||
(3) | Pb | 0.110 | 0.212 | 1.000 | |||||
(4) | Ph | 0.907 | 0.965 | 0.360 | 1.000 | ||||
(5) | Pd | 0.563 | 0.654 | 0.846 | 0.758 | 1.000 | |||
(6) | Pm | 0.177 | 0.290 | 0.982 | 0.429 | 0.893 | 1.000 | ||
(7) | 0.947 | 0.955 | −0.025 | 0.902 | 0.451 | 0.041 | 1.000 | ||
(8) | Ar | 0.951 | 0.990 | 0.148 | 0.953 | 0.596 | 0.219 | 0.968 | 1.000 |
|
Empirically, we adopt two methods: ordinary least square (OLS) and negative binominal regression. Since the explained variable is nonnegative count data (count data), we mainly take the ordinary least square method as the corresponding method and the negative binomial regression as the main research result. In previous literature, negative binomial regression has been considered as the main way to process count data.
The model is set as follows:In the model, represents the unobservable part or the individual heterogeneity in conditional expectation, and formula (2) can be obtained through calculation: is the deterministic function of the explanatory variables and is still a random variable, which belongs to the unobservable part. Therefore, the empirical model still obeys the Poisson distribution:Since is usually greater than 0, it is assumed to obey the Gamma distribution, and it is assumed that , a > 0. Therefore, the expected value of is 1 and the variance is a. The negative binomial distribution can be obtained by substituting its probability density into the equation, and MLB can be estimated to form a negative binomial regression.
To further verify whether the empirical results under the negative binomial regression estimation are consistent with the Poisson regression results, Table 3 and 4 sort out the negative binomial regression estimation results. The coefficient estimates β consistent with each explanatory variable can be deduced from equation (2). In the above formula, is the explanatory variable. Empirically, the impact on the explained variable is discussed based on the number of doctoral teaching staff, the number of associate professors, the number of graduates and undergraduates, and the teaching and scientific research assets of schools in the province.
Table 4 first estimates regression and negative binomial regression, and the explained variable is the total number of approved projects. The estimation results show that the number of teaching staff, students, and teaching and scientific research assets has a significantly positive impact on the project approval in colleges, universities, and regions. Based on the consistency of OLS estimation and NBR estimation results, taking the sixth column of Table 4 as an example, the empirical results of the estimation combination show that the impact of teaching staff with a Ph. D. degree on the explained variable is 0.227, indicating that the increase in the number of teaching staff can positively drive the growth rate of the region. Secondly, the coefficient estimates of graduates and undergraduates are 0.737 and 0.747, respectively, indicating that there is a positive effect on the total number of project approval when the number of students increases.
|
||||||
Explained variable: total number of approved projects | ||||||
(1) OLS | (2) OLS | (3) OLS | (4) NBR | (5) NBR | (6) NBR | |
|
||||||
ln (Pa) | 0.002 | 0.003 | 0.505 | 0.227 | ||
(0.001) | (0.002) | (0.046) | (0.120) | |||
ln (Ph + Pd) | 0.004 | 0.014 | 0.889 | 0.603 | ||
(0.003) | (0.003) | (0.114) | (0.849) | |||
0.002 | −0.006 | 0.621 | 0.737 | |||
(0.0003) | (0.007) | (0.126) | (0.368) | |||
ln (Pm) | 0.973 | 0.002 | 0.758 | 0.747 | ||
(0.093) | (0.001) | (0.441) | (0.107) | |||
ln (Ar) | 0.077 | 0.204 | ||||
(0.018) | (0.047) | |||||
Constant term | −1.593 | −0.790 | −0.054 | 1.951 | 2.256 | 2.046 |
(0.831) | (0.852) | (0.876) | (0.044) | (0.039) | (0.048) | |
N | 483 | 483 | 483 | 483 | 483 | 483 |
R2 | 0.916 | 0.894 | 0.929 | |||
Log likelihood | −1658.582 | 1675.872 | −1625.618 | |||
|
Panel estimation is done in Table 5. First, the random effect empirically shows that the number of teaching staff with a Ph. D degree, the number of undergraduate students, and the scale of school teaching and scientific research assets in the province have a significantly positive impact on the number of photothermal projects. It shows that with the development of China’s economy and industrial demands, human resources provided by colleges and universities and the use of teaching and scientific research resources have a significantly positive impact on whether the school is approved or not. In addition, the possible regional differences should be considered, for example, the industrial technology in north China is more intensive than that in south China, mainly due to the difference in the distribution of natural resources. Therefore, the fixed effect is also analyzed in Table 5. The empirical results show that the number of teaching staff with a Ph. D degree and the number of professors and associate professors in the province have a significantly positive impact on the explained variable. For example, in the fixed effect (2) in Table 5, all explanatory variables are significant, indicating that the increase in the number of teaching staff with a Ph. D degree in the province has a positive and significant impact on the number of projects. And the increase in the number of graduate and undergraduate students as planned also has a positive impact on the scientific research development of the university and the region. Both correlations reflect that in terms of the scientific research development, the approval of scientific research projects of solar thermal or the follow-up patent application derived from the scientific research projects, the investment in teaching and scientific research, and human resources in colleges and universities have a key impact. The results also show that the development of scientific research projects, industrial demand, and green sustainable innovation complement each other.
|
||||
Explained variable: | The total number of approved projects | |||
The total number of approved projects | Random effect (1) | Random effect (2) | Fixed effect (1) | Fixed effect (2) |
|
||||
ln (Pa) | 0.504 | 0.510 | 0.776 | 0.410 |
(0.326) | (0.127) | (0.398) | (0.189) | |
ln (Ph + Pd) | 0.212 | 0.641 | 0.897 | 0.844 |
(0.276) | (0.462) | (0.404) | (0.437) | |
0.008 | 0.554 | |||
(0.290) | (0.132) | |||
ln (Pm) | 0.550 | 0.510 | ||
(0.204) | (0.221) | |||
ln (Ar) | 0.153 | 0.159 | 0.218 | 0.322 |
(0.091) | (0.091) | (0.090) | (0.065) | |
Constant term | −8.922 | −4.261 | ||
(1.930) | (2.660) | |||
N | 483 | 483 | 483 | 483 |
|
4. Conclusion
This paper empirically analyzes the count data through regression and constructs a negative binomial regression empirical model through the project data of the National Natural Science Foundation of China on solar thermal and the China education statistics yearbook from 2018 to 2019. The results show that the human resources provided by colleges and universities and the use of teaching and scientific research resources have a significantly positive impact on whether the department of thermodynamics is approved or not. The empirical results show the importance of talent education and training. Talent training drives scientific research funding, which is extremely important for the development of environmental science and has a key impact on the correlation between China’s industrial demand and green sustainable innovation.
Based on the national science fund, this paper analyzes the factors affecting scientific research in the education system. However, this paper also has many limitations. Due to the lack of centralized release of official data in China, the project data that can be made public can only be obtained by crawling, and the research year is lagging behind and short. At the same time, although quantitative methods can find correlation more directly, they cannot completely explain the meaning behind the data. In the follow-up research, it is proposed to further explore the issues of industry-university cooperation. Industry-university-research cooperation can effectively coordinate the innovation needs of various innovating entities, thereby significantly improving the transformation efficiency of basic research funding into the innovation of middle and downstream enterprises. A series of national science fund for cultivating innovative talents and teams can further promote the patent output of enterprises. How to effectively combine scientific research with current and future environmental development is the focus of follow-up research.
Data Availability
Requests for access to the data used to support the findings of this study should be made to Jian Chu ([email protected]).
Conflicts of Interest
The authors declare that they have no conflicts of interest.
Authors’ Contributions
Tu Yan performed conceptualization and formal analysis, developed the methodology, and wrote the initial draft. Chu Jian collected data, wrote the original draft, and performed supervision. Mohd Asif Shah wrote the final draft and performed supervision.
This study was supported by the 2021 Talent Introduction Research Start-Up Fund Project of Suqian University.