Maintaining consumer protections in midst of innovation
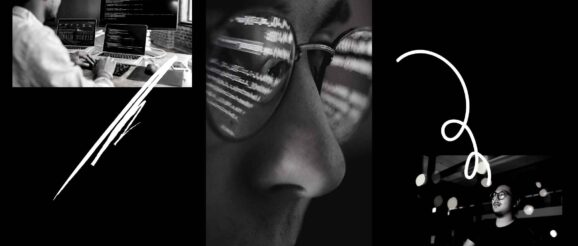
Recent years have witnessed an increased adoption of black-box AI methods, particularly those involving Deep Learning, within products & services.
However, despite their superior performance, such black-box methods increase the risk of legal liability for manufacturers & service providers, due to their non-explainable, & non-transparent decision making. At the same time, we do not want to dilute existing consumer protections for our citizens, while addressing the concerns of manufacturers.
In this article we explore the possibility of having our cake & eating it too, ie., we explore the possibility of maintaining strong consumer protection, while also maintaining the pace of AI innovation. We believe that this can be facilitated by the creation of a national data repository, that allows companies to test the fairness of their AI augmented products & services. We present a case for this below.
Rise of black-box AI increases liability
The incorporation of black-box AI into goods & services, to make them “smarter,” is beginning to upend traditional notions of product & service liability, particularly because their intelligence is governed by oracles – black boxes containing opaque decision rules, that return a “correct” answer, but which are nevertheless, not amenable to legal scrutiny. This opens up companies to allegations of discrimination from unhappy customers, that are difficult to defend against.
Allegations of discrimination cause liability
It’s almost a truism that no company can satisfy all its customers, all the time. Unhappy customers regularly demand explanations from companies, which results in significant legal & PR implications. In the near future, this is likely to be increasingly true for systems engaged in self-driving navigation, credit recommendation, & medical diagnosis, to name a few, as their decisions require a high-degree of traceability & justification, to survive legal challenges from customers allegedly harmed during their operation.
Consider a situation where a bank is using a deep learning system to evaluate potential loan applicants. The bank’s decision, contingent on the recommendation of its software, might be challenged in court by an disgruntled applicant, who was denied a loan, on the grounds that it was racist or discriminatory. Such a system is a serious liability risk for the bank, given that their programmers cannot fully explain how the deep learning model arrived at its answer.
In this regard, there’s good reason to suspect that this liability will not reduce, despite advances in the field of “Explainable AI”.
Intelligence cannot be encompassed by logical rules
There is a trade-off between the intelligence of a system, & it’s degree of decision transparency. As sub-symbolic approaches such as deep learning have become more effective, our ability to explain their reasoning has correspondingly fallen. Work in the area of explainable AI, though useful, is no silver bullet, as intelligence is not akin to an algorithm operating on predictable input.
Algorithms don’t cover all potential scenarios
Current approaches in explainable AI involve tracing its decisions via techniques such as first-order logic, measures of marginal contribution of predictive features, or simple what-if scenarios, to name a few. However, despite their utility, such techniques suffer from the problem of being mostly applicable to situations spanned by the training data. This is not ideal in domains such as in navigation or medical diagnosis etc., as the potential list of real-world scenarios encountered by the AI system, is likely to be far larger, & be far more complex than what could be tackled via standard data augmentation hacks, like adding noise to existing data points.
Consider a situation where you’re walking on the pavement, & you’re hit by a self-driving car that swerved on to you, to avoid hitting a group of children that darted onto the street. What is your legal recourse, here? What should have the car done? Readers may recognize this as a variation of the “Trolley Problem”, from Philosophy, which has no solution.
The typical data-driven approach to “solve” the above problem would be for a company to train its AI navigation with thousands of hours of data, which captured the responses of what human volunteers did in the same scenario, simulated or otherwise. However, such a data-driven approach is problematic for multiple reasons. Firstly, there’s no way to capture all of the potential scenarios that might occur while driving on chaotic streets. Secondly, it’s difficult to prevent the unconscious encoding of societal biases of human volunteers within the training data (Is an older adult’s life less valuable than a child’s life? Is it justifiable to kill/maim one person to save many?). Moreover, there may be an additional danger of the system arriving at a poor decision, if some volunteers don’t fit the legal standard of a “reasonable person”.
For example, some US police departments have faced flak for employing “smart policing” systems that use training data from actual crime reports, to guide the deployment of their personnel. Allegations of racist profiling began flaring up after the “impartial” statistical algorithms of these systems, began recommending greater policing in areas predominantly populated by the underprivileged, & minorities.

Intelligence is embodied cognition
One could speculate that in the near future, we will achieve an ideal situation of both intelligent & explainable AI. We believe, however, that it’s more prudent to focus our efforts on formulating legal frameworks to reconcile with our current, less than ideal situation, rather than wait for the future, as history suggests that such optimism is generally misplaced, given the failures of rule-based, symbolic AI, like CYC, & expert systems.
In his influential critique of such rule-following systems, the philosopher Hubert Dreyfus posited that intelligence is embodied cognition, & thus, cannot be merely based on a disembodied set of logical rules. This is because an overwhelming amount of our understanding of the world is derived from the fact that we possess bodies that are constantly engaged in coping with our environment. Such “bodily knowledge” cannot be subsumed via purely symbolic rules, as such rules can only describe a small subset of environmental interactions. His assertion is supported by Moravec’s paradox, which is an observation that it’s far easier to get machines to learn “higher-order” activities, like logic, rather than “lower-order” physical ones, which involve environmental interaction.
Multiple notions of fairness make legal defense difficult
Companies could try to preempt allegations of discrimination, by checking for the fairness of their AI decision engines. Some ways to accomplish this currently are through Google’s What-if tool, & Microsoft’s Fair-learn toolkit. The What-if tool allows investigations of fairness via slicing datasets by their features, comparing performance across those slices, & identifying subsets of data on which the model performs best. Similarly, the Fair-learn toolkit also helps navigate trade-offs between fairness and model performance. However, despite the availability of these tools, it’s still difficult for companies to defend against allegations of unfair discrimination, as there are multiple, incompatible notions of fairness.
For example, in the case of loan disbursal, in an effort to allocate loans purely on the merit of criteria other than demography or gender, the bank may decide to not include the associated fields within the training data (Group unaware criteria). However, the resultant system decisions may still end up biased against certain minorities or genders, due to already existing historical & social inequities. Including said demographic fields would also not help to pin down fairness either, as there is no universal notion of what’s fair (Should the system’s decisions satisfy group thresholds, or should they instead satisfy demographic parity? Should the system’s decisions satisfy equal opportunity, or should they satisfy equal accuracy?)
Liability puts the breaks on innovation
We’ve seen thus far that black-box AI innovation increases liability due to difficulty in tracing decisions, & justifying that they’re unbiased. We’ve also seen that massive data sets still cannot span all the potential problematic real-world scenarios, that an AI system might encounter. Could the problem be ameliorated by incorporating extra safety features into the final product or service? Unfortunately, this isn’t the case, as such additional features have the side-effect of raising costs, which acts as a damper on product innovation, in general.
A standardized data set lowers liability risk & boosts innovation
The solution is to reduce both the risk of liability, & the cost of innovation, for companies. This would cause no detriment to AI innovation, & also not dilute existing consumer protection. This can be achieved through creation of a standardized data set, on which companies test the fairness of their AI-augmented products & services. Such an initiative, which could be either industry-led or government-led, would have a number of positive effects.
Amortizes risks & costs across all firms
We can take a leaf out of the playbook of the Oil industry, which has used some form of an industry-funded or government-funded National Data Repository (NDR), since decades. The purpose of NDRs is two-fold:
1. Preserve & update data generated by exploration firms within a country; &
2. Lower business risk for all member firms via sharing data related to exploration, production, & transportation of oil.
Additionally, NDRs also specify best practices for the data schema, data access & data updating, for all the member firms.
Such NDRs provide an existing template that our industry can draw from. This would lead to similar benefits such as spreading of liability risk across all firms, due to data sharing & updating, as well as lowered cost of product development across all firms, due to standardization of practices across the industry.
Reduces legal risk
A standardized data set would lower the risk of liability for all firms in other ways as well. Our current legal frameworks are under-prepared to deal with AI liability, as our laws weren’t framed with AI-augmented goods & services in mind, leading to ambiguity, which raises the risk of damaging lawsuits. Such a data set would kickstart efforts to define AI-fairness, as well as define other concepts like that of a “reasonable machine” – the machine analogue of a “reasonable person”. Currently we use the “reasonable person” definition to determine negligence. However, analogous definitions involving machines, would inevitably be required as AI performance surpasses that of humans, something that has already occurred within certain health disciplines of medical diagnosis.
Improves incentive to innovate
Standardization would also institute a minimum, legally recognized, baseline data set, over which the fairness of all AI-augmented products, within a particular domain, could be evaluated. While bigger firms would no doubt invest in also creating their own independent, non-public data sets, to outperform others, the existence of a public data set, would boost incentives for innovation, as it would lower the barrier of entry, & allow other smaller firms to also compete.
Increases scrutiny
Some of the most secure & robust software ever created is open sourced. This is explained by Linus’ Law of software development which states that, “given enough eyeballs, all bugs are shallow”. As AI becomes more intelligent, we may not be able to probe the reasoning behind its decisions, but we can at least ensure that the performance of any product meets some minimum, legally accepted level of fairness, thereby reducing the impact of harmful biases. This is best accomplished with a national, publicly available dataset, that’s open to scrutiny from many experts, instead of each company working on its own private data silo, as it’s the case now.
The post Maintaining consumer protections in midst of innovation appeared first on Analytics India Magazine.