META Unveils 24,576 GPU Data Center Scale Cluster To Advance AI Innovation
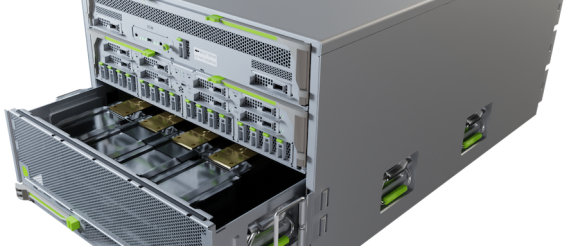
Meta continues its AI innovation through strategic investment in hardware infrastructure, crucial for advancing AI technologies. The company recently unveiled details on two iterations of its 24,576-GPU data center scale cluster, which is instrumental in driving next-generation AI models, including the development of Llama 3. Meta continues its AI innovation through strategic investment in hardware infrastructure, crucial for advancing AI technologies. The company recently unveiled details on two iterations of its 24,576-GPU data center scale cluster, which is instrumental in driving next-generation AI models, including the development of Llama 3. This initiative is a foundation of Meta’s vision to generate open and responsibly built artificial general intelligence (AGI) accessible to all. In its ongoing journey, Meta has refined its AI Research SuperCluster (RSC), initially disclosed in 2022, with 16,000 NVIDIA A100 GPUs. The RSC has been pivotal in advancing open AI research and fostering the creation of sophisticated AI models with applications spanning many domains, including computer vision, natural language processing (NLP), speech recognition, and more. Building upon the RSC’s successes, Meta’s new AI clusters enhance end-to-end AI system development with an emphasis on optimizing the researcher and developer experience. These clusters integrate 24,576 NVIDIA Tensor Core H100 GPUs and leverage high-performance network fabrics to support more complex models than previously possible, setting a new standard for GenAI product development and research. Meta’s infrastructure is highly advanced and adaptable, handling hundreds of trillions of AI model executions daily. The bespoke design of hardware and network fabrics ensures optimized performance for AI researchers while maintaining efficient data center operations. Innovative networking solutions have been implemented, including a cluster with remote direct memory access (RDMA) over converged Ethernet (RoCE) and another with NVIDIA Quantum2 InfiniBand fabric, both capable of 400 Gbps interconnects. These technologies enable scalability and performance insights crucial for the design of future large-scale AI clusters. Meta’s Grand Teton, an in-house-designed, open GPU hardware platform, contributes to the Open Compute Project (OCP) and embodies years of AI system development. It merges power, control, compute, and fabric interfaces into a cohesive unit, facilitating rapid deployment and scaling within data center environments. Addressing the often under-discussed yet critical role of storage in AI training, Meta has implemented a custom Linux Filesystem in Userspace (FUSE) API supported by an optimized version of the ‘Tectonic’ distributed storage solution. This setup, paired with the co-developed Hammerspace parallel network file system (NFS), provides a scalable, high-throughput storage solution essential for handling the vast data demands of multimodal AI training jobs. Meta’s YV3 Sierra Point server platform, backed by Tectonic and Hammerspace solutions, underscores the company’s dedication to performance, efficiency, and scalability. This foresight ensures the storage infrastructure can meet current demands and scale to accommodate the burgeoning needs of future AI initiatives. As AI systems grow in complexity, Meta continues its open-source innovation in hardware and software, contributing significantly to OCP and PyTorch, thereby promoting collaborative advancement within the AI research community. The designs of these AI training clusters are integral to Meta’s roadmap, aiming to expand its infrastructure with the ambition of integrating 350,000 NVIDIA H100 GPUs by the end of 2024. This trajectory highlights Meta’s proactive approach to infrastructure development, poised to meet the dynamic demands of future AI research and applications. Engage with StorageReview Newsletter | YouTube | Podcast iTunes/Spotify | Instagram | Twitter | TikTok | RSS Feed