Not so fast, InsurTech- long-tailed and unique claims are the Kryptonite to your innovation super power
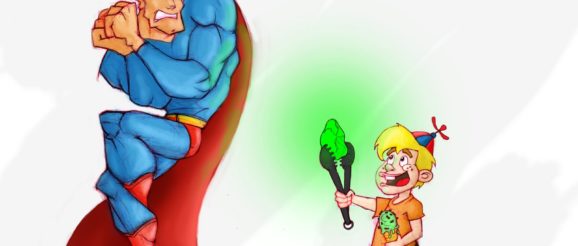

Artificial intelligence, machine learning, data analysis,
ecosystem insurance purchases, online claim handling, application-based insurance
policies, claim handling in seconds, and so on.
There’s even instant parametric travel cover that reimburses costs-
immediately- when one’s planned air flight is delayed. There are clever new risk assessment tools
that are derived from black box algorithms, but you know what? Those risk data are better than the industry
has ever had! Super insurance, InsurTech
heroes! But ask many insureds or claim
handlers, and they’ll tell you all about InsurTech’s weakness, the kryptonite
for insurance innovation’s superheroes (I don’t mean Insurance Nerd Tony Cañas)- those being- long-tailed or unique claims.
If insurance was easy you wouldn’t be reading this. That is simple; much of insurance is
not. Determining risk profiles for
thefts of bicycles in a metro area- easy.
Same for auto/motor collision frequency/severity, water leaks, loss of
use amounts, cost of chest x-rays, roof replacement costs, and burial costs in most
jurisdictions. Really great fodder for
clever adherents of InsurTech- high frequency, low cost cover and claims. Even more complex risks are becoming easier
to assess, underwrite and price due to the huge volume of available data
points, and the burgeoning volume of analysis tools. I just read today that a clever group of UK-based
InsurTech folks have found success providing comprehensive risk analysis
profiles to some large insurance companies- Cytora –
that continues to build its presence. A
firm that didn’t exist until 2014 now is seen as a market leader in risk data
analysis and whose products are helping firms who have been around for a lot
longer than 5 years (XL Catlin, QBE, and Starr Companies) Seemingly a perfect fit of innovation and
incumbency, leveraging data for efficient operations. InsurTech.
But ask those who work behind the scenes at the firms, ask
those who manage the claims, serve the customers, and address the many
claim-servicing challenges at the carriers- is it possible that a risk that is
analyzed and underwritten within a few minutes can be a five or more year
undertaking when a claim occurs? Yes, of
course it is. The lion’s share of
auto/motor claim severity is not found within the settlement of auto damage, it’s
the bodily injury/casualty part of the claim.
Direct auto damage assessment is the province of AI; personal injury
protection and liability decisions belong in most part to human interaction. Sure, the systems within which those actions
are taken can be made efficient, but the decisions and negotiations remain outside
of game theory and machine learning (at least for now). There have been (and continue to be)
systems utilized by auto carriers in an attempt to make uniform more complex
casualty portions of claims ( see for example Mitchell) but lingering ‘burnt fingers’
from class action suits in the 1980’s and 1990’s make these arms’ length tools trusted
but again, in need of verification.
Property insurance is not immune from the effects of
innovation expectations; there are plenty of tools that have come to the market
in the past few years- drones, risk data aggregators/scorers, and predictive
algorithms that help assess and price risk and recovery. That’s all good until the huge network of
repair participants become involved, and John and Mary Doe GC prices a rebuild
using their experienced and lump sum pricing tool that does not match the
carrier’s measure to the inch and 19% supporting events adapted component-based
pricing tool. At that intersection of ideas,
the customer is left as the primary and often frustrated arbiter of the claim
resolution. Prudent carriers then revert
to analog, human interaction resolution. Is it possible that a $100K water loss can
explode into a $500K plus mishandled asbestos abatement nightmare? Yes, it’s very possible. Will a homeowner’s policy customer in Kent be
disappointed because an emergency services provider that should be available
per a system list is not, and the homeowner is left to fend for himself? The
industry must consider these not as outlier cases, but as reminders that not
all can be predicted, not all data are being considered, and as intellectual
capital exits the insurance world not all claim staff will have the requisite
experience to ensure that which was predicted is what happens.
The best data point analysis cannot fully anticipate how
businesses operate, nor how unpredictable human actions can lead to claims that
have long tails and large expense. Consider
the recent tragedy in Paris with the fire at the Cathedral of Notre Dame. Certainly any carriers that may be involved
with contractor coverage have the same concerns as all with the terrible loss,
but they also must have concerns that not only are there potential liability coverage
limits at risk, but unlike cover limits, there will be legal expenses
associated with the claim investigation and defense that will most probably
make the cover limits small in comparison.
How can data analysis predict that exposure disparity, when every claim
case can be wildly unique?
It seems as underwriting and pricing are under continued
adaptation to AI and improved data analysis it is even more incumbent on companies
(and analysis ‘subcontractors’) to be cognizant of the effects of unique claims’
cycle times and ongoing costs. In
addition, carriers must continue to work with service providers to recognize
the need for uniform innovation, or at least an agreed common denominator tech
level.
The industry surely will continue to innovate and encourage those InsurTech superheroes who are flying high, analyzing, calculating and selling faster than a speeding bullet. New methods are critical to the long-term growth needed in the industry and the expectation that previously underserved markets will benefit from the efforts of InsurTech firms. The innovators cannot forget that there is situational kryptonite in the market that must be anticipated and planned for, including the continuing need for analog methods and analog skills.
Patrick Kelahan is a CX, engineering & insurance professional, working with Insurers, Attorneys & Owners. He also serves the insurance and Fintech world as the ‘Insurance Elephant’.
I have no positions or commercial relationships with the companies or people mentioned. I am not receiving compensation for this post.
Subscribe by email to join the 25,000 other Fintech leaders who read our research daily to stay ahead of the curve. Check out our advisory services (how we pay for this free original research).