Survival of the most informed: The journey to innovation begins with data – Scoophot
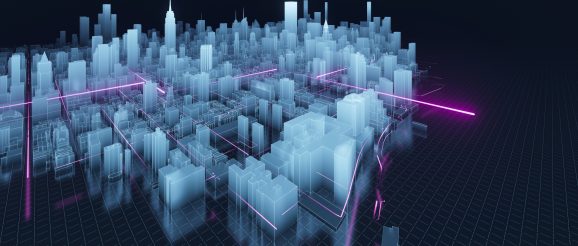
Were you unable to attend Transform 2022? Check out all of the summit sessions in our on-demand library now! Watch here.
While business transformation has always been critical to staying relevant and competitive, global disruptions brought on by the COVID-19 pandemic created an urgency to accelerate innovation to keep pace with market conditions and changes in customer demand. In fact, many digitally transformed companies have not only survived — they’ve thrived.
According to a 2021 McKinsey Survey, top-performing companies now obtain a larger share of their sales from products or services that didn’t exist just one year ago. These companies are making more aggressive plans to differentiate themselves with technology, and some are preparing to reinvent their value proposition altogether.
Business insights gleaned from innovations in data, analytics, and machine learning (ML) technologies are driving this shift. As these technologies have become mainstream and the volume of data has grown exponentially, business leaders are embracing a fundamental truth: The journey to innovation begins with data, and successfully becoming a data-driven organization begins by defining a modern data strategy and proliferating it throughout the company culture.
Defining the modern data strategy roadmap
In a 2021 executive survey on data leadership by New Vantage Partners, 92% of C-suite leaders stated that organizational culture remains the main barrier to becoming a data-driven organization.
MetaBeat will bring together thought leaders to give guidance on how metaverse technology will transform the way all industries communicate and do business on October 4 in San Francisco, CA.
Register Here
A modern data strategy works to create a culture that treats data as a strategic resource and invests in the right data infrastructure, solutions, people, processes and tools. It engages everyone in a data-driven vision by educating teams to boost data proficiency and enabling data-driven decision making from the top down. The strategy eschews monolithic, one-size-fits-all data structures, instead opting for data lakes and purpose-built databases and analytics engines to increase agility, easily scale and move data and expand the use of analytics and ML throughout the organization.
Modern data strategies also eliminate structural and departmental data silos, ensuring that all the right people can access data at the right time and with the right controls, even if they aren’t database administration or infrastructure management experts. An effective data strategy meets people where they are in their journey and provides tools to run analytics and ML that match their different skill levels.
Three precepts guide the implementation of the strategy: unify data to create a single source of truth; modernize data infrastructure, analytics and ML; and innovate with the modernized environment to create new processes, customer solutions, and experiences.
Unifying data
Unifying data and putting it to work across multiple data stores can give companies a full picture and single source of truth of their customers and business. Many companies are doing this by making a central data repository — or data lake — the foundational element of their unification strategy.
Data lakes allow various roles within the organization — data scientists, data engineers, and business analysts — to collect, store, organize, and process valuable data with their choice of analytics and ML tools in a governed way. Nasdaq knows the value of data lakes firsthand. The company was able to scale from 30 billion records to 70 billion records a day by building a cloud-based data lake, and can now load financial market data five hours faster and run relational database queries 32% faster using a cloud data warehouse.
Additionally, when all data is unified, it becomes exponentially more powerful because you can put it to work anywhere. Businesses can also modernize analytics and ML by adopting a tailored, yet unified approach. Modern analytics tools can look across multiple data stores and allow the right people to access the right data holistically to meet specific use cases.
Purpose-built analytics services can discover, access, interpret and visualize data in a manner that serves a specific business need. For example, Netflix uses a cloud based large-scale streaming data analytics platform to ingest, augment and analyze the multiple terabytes of flow log data its network generates daily, with sub-second response times for analytics queries. These tools and services also manage data access with the proper security and data governance controls.
Modernizing data, analytics and ML
One of the best ways to modernize large data infrastructure is to move away from legacy on-premises data stores to a fully managed end-to-end cloud platform that removes the undifferentiated heavy lifting.
IDC research found that businesses that moved their databases from on-premises to managed cloud-based services achieved 86% faster deployments of new databases, experienced 97% less unplanned downtime, and had a five-month average investment payback period. In practice, Samsung recently migrated 1.1 billion users to a cloud-based relational database service (RDS) across three continents and was able to cut monthly database cost by 44% while achieving 60 millisecond-or-less latency 90% of the time.
Multi-database strategies
Data is now so diverse that companies must embrace a multi-database strategy that includes structured relational, non-relational and large-scale data stores, as well as purpose-built databases that are optimized for specific workloads, like key-value databases for high-traffic web applications, time series databases for IoT applications, or graph databases for recommendation engines.
Case in point: Global information company Experian moved to a cloud-first microservices-driven architecture built on a fully managed, serverless, key-value NoSQL database. The company also replaced its legacy relational database with a fully-managed Relational Database Service (RDS). By automating time-consuming administration tasks like hardware provisioning, database setup, patching, and backups, the time spent to configure and deploy servers went from 60 to 90 days to a matter of hours.
Security, reliability, performance
It’s critical to note that moving from legacy databases to cloud databases is not just about using the latest technologies and getting better latency, it also enables developers to have better security, reliability, and performance — all without the hassle of dealing with undifferentiated heavy-lifting associated with day-to-day operations of these databases. Ultimately, it frees up time for developers, allowing them to focus on innovation and solving complex problems instead of managing database infrastructure.
Cloud environments allow businesses to harness ML at scale by standardizing the development process. Modern cloud ML platforms provide scalable infrastructure, integrated tooling, appropriate practices for responsible use of ML, and tools for users of all ML skill levels.
Intuit created an artificial intelligence (AI) driven expert platform that combines human expertise with ML to accelerate development and incorporate ML into its products. Development lifecycles that used to take six months now take less than a week. Intuit has also used ML to save customers over 25,000 hours via self-help for receipt processing and over 1.3 million hours in receipt processing.
Data strategy: Innovating with modernized analytics, BI and ML
While innovation can take place at each of the three pillars of the modern data strategy, it occurs most often at their intersection, when databases and analytics solutions are infused with ML.
Modern, unified data architectures are connecting different data stores and analytics tools into a coherent, integrated ML development environment that uses automated data collection, prep, and labelling services to ensure that the right data is fueling the model and that it is relevant for the model training and deployment stages. Managed ML services and integrated ML innovations are making modeling and implementation simpler, more democratized and more tailored to specific business challenges and outcomes.
ML is being integrated into these services and large-sale data stores like data lakes and data warehouses to dramatically reduce the time and complexity involved in running ML models at scale. Data stores and analytics services with built-in ML eliminate the need for cumbersome data preparation, feature engineering, algorithm selection, training and tuning, inference, and model monitoring.
For example, developers can use ML embedded into an Amazon RDS database to run models on transactional data using a simple SQL query.
Advantages of co-located ML
ML innovation is already having a measurably positive impact. Health technology company Philips developed a regulatory-compliant, platform-as-a-service (PaaS) solution, Philips HealthSuite, to provide tools and cloud capabilities to advance digital healthcare through imaging AI and ML solutions.
Philips’ ML solution aims to help optimize the quality of healthcare by delivering care quickly and significantly reducing human error. By working toward facilitating diagnostic recommendations using ML, medical professionals will have the tools they need to deliver accurate diagnoses and create treatment plans.
A great example of the advantages of co-located ML is the online job search firm Jobcase, which streamlined and accelerated ML models within its cloud data warehouse by using the in-database local inference capabilities afforded by integrated ML services.
Not having to move large amounts of data across networks or set up complex custom data pipelines to move from their data warehouse to ML platforms to perform quick ML experimentation allows the company’s data scientists to model inference on billions of records in a matter of minutes, directly in its data warehouse.
Maturing data strategy
Data is the gateway to new opportunities. With the right data strategy and culture, organizations can control their growing data, find insights from diverse data types, and make it available to the right people and systems.
The net result of embracing a modern data strategy is becoming the “most informed” organization with ready-made intelligence for applications and workflows that address business problems end-to-end. As an organization’s data strategy matures, it will transform how they solve problems and build customer experiences — which will lead to more breakthroughs in all fields including healthcare, smart buildings, homes and cities, personalized consumer experiences, and efficient manufacturing operations.
Swami Sivasubramanian is vice president of analytics, database and machine learning at AWS.