The Impact of AI in Transportation Sector | CPO INNOVATION
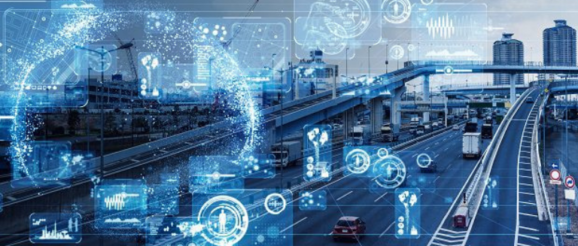
The transportation sector is quickly progressing in the active application of Artificial Intelligence (AI) on crucial tasks such as self-driving vehicles. Here, the common public will question the reliability and protection of an AI system. The transportation industry has several significant challenges, such as capacity issues, increased emissions, and power wastage, provide plenty of opportunities for AI technology along with the ROI generated for the companies behind it.
There is currently a notable investment in the broad-based automotive industry, focusing on Artificial Intelligence to optimize self-driving technology. Many firms are targeting higher levels of vehicles working with autonomous technologies for mass production. At the same time, new business players are asserting innovation that plays a major role in transforming the automotive market. Uber works on robot taxis; Tesla is developing its Autopilot system and Google, through its subsidiary Waymo, is focused on the production of autonomous cars. (McKinsey, 2017)
What makes artificial intelligence a dynamic tool to solve transportation problems?
Transportation problems arise when the behavior of the system is too difficult to model on a pattern that is predictable in nature, impacted by things like human errors, traffic or accidents. AI can assist with the unpredictability in such cases.
Observed data is appropriately used by AI to make and predict decisions. Neural networks and genetic algorithms are perfect AI methods for dealing with unpredictability of these types. AI is developing and can be utilized in various ways. Few examples are herewith-
Transportation Planning
Artificial intelligence has the scope in designing a transit network optimally for a provided community, in the development of an optimal work plan for the maintenance and rehabilitation of a pavement network, and in the development of a group of traffic signals along with a suitable timing plan.
Driver Behaviour
In case of law enforcement capacities, AI can be useful and could be challenge for the human officers because of the speed at which passengers, as well as vehicles, can get in and out of sight, with AI, thus providing a remedy for the problem. With data processing capabilities including advanced analytics, AI can help detect and identify when a driver is texting or drinking while driving.
Data Management
Artificial intelligence can handle the transmission and storage of obtained information as well as optimize communication to ensure the best link, Artificial intelligence can be used in non-linear analysis of device actions in which inputs & outputs, modeling of traffic demand, or modeling transport infrastructure health as a feature of traffic, building and weathering.
Traffic Signalling
AI can be used for signal traffic control at ramp metering on freeways, road intersections, positive train control on railways, dynamic route guidance. AI can be used for automatic incident detection, image processing can be used to collect traffic data and to identify cracks in bridge structures or pedestrian pavements and diagnosis of transport equipment.
Sustainable Transportation
Electric vehicles are becoming more prevalent, most of the countries are sharing the initiative to incorporate advanced technologies into their highways, China has already constructed a smart road that can charge electric vehicles while driving, and many smart road designs have included solar panels for charging of the vehicles, sensors and AI feedback to control the traffic patterns and to avoid congestion efficiently.
Autonomous Transportation
Self-driving trucks are effective to take over several dirty works like snow plowing and garbage collection, they could be used in freight, they could carry 2,000,000 pallets per year, the safer way to travel is the driverless bus, such buses have already popped up throughout Europe, utilizing cameras, sensors and GPS to provide a safe journey to passengers towards their destinations. Trucks which are self-driving will increase the productivity and reduce the frequency of road accidents, AI is important to these driverless vehicles because of their computing, control and optimization capabilities, and AI can help make autonomous vehicles safer and more common.
Autonomous Rail Rapid Transit is a rail-free train system that operates on a virtual painted track that is detected and followed by the train’s computer system, autonomous trains along with the facilities of machine learning and Artificial Intelligence in freight and passenger transportation have been tested in several countries.
But the edge-cutting improvements arise through Neural Networks. In most problem-solving fields, artificial neural networks (ANNs) have proven to be an important development. Equally rapid growth in the commercial mainstream use of ANNs has followed increased research interest in ANN applications. Some of the transportation examples of ANN’s are:
Driver Behaviour
Work in this area divides neatly into two schools: modeling tactical or instinctive decisions both explain using neural networks to analyze data from interactive route selection simulators. Volunteer drivers participating in these experiments were asked to make route decisions based on a wide variety of subjective values. Volunteer drivers who participated in these tests were asked to make route choices based on a wide variety of subjective values. A neural network was then trained to make similar decisions using these results. The network was then asked to make route selection predictions for data items that it hadn’t seen before; strong replication levels were recorded for the actual decisions. A neural network has been shown to be a faster and more accurate analysis method than alternative techniques such as logit models.
The second school is characterized by work aimed at building real-time models that can drive a car around a road environment. Lyons and Hunt (1992) described an initial study using data gathered from an interactive model of computer simulation that attempted to model maneuvers overtaking. This research was expanded later, and the problem was reparametrized to allow the use of real data. In this later work, learning vector quantization networks were used; the “winner takes all” approach seems to be well suited to modeling this form of decision-making.
Pavement Maintenance
A framework that offers advice on the best ways to maintain a road surface can be divided into two sub-systems: a diagnostic element and a prognostic element. Both received attention from the community of the neural network. As an image processing problem, the diagnostic element has been successfully attacked. Neural networks are used in this work to process images of road surfaces and to categorize features within them into various types of defects. Before the neural network stage was reached, considerable amounts of classical image processing were still needed, emphasizing the point that neural networks usually do not provide a complete solution to a problem. Another feature of pavement diagnosis is that road marking that has been destroyed or blurred are immediately remembered. Here, as associative memory, a Hopfield net was used to map incomplete images to templates. Here, we see the concept of using neural networks as sub-systems; what makes this research unique is that two separate neural network architectures are used for the various object association and recognition sub-tasks.
Vehicle Detection/Classification
Following the idea of a highway with sensor arrays, a lot of work has been done to extract the maximum amount of information from the generated signals. The class of passing vehicles is a typical derived quantity: a factor of their wheelbase, number of axles, weight, etc. Basic function networks were applied with some success to this problem; although the numerical results were not outstanding, an algorithmic approach-based commercial system performed even worse. Obviously, more thorough testing is needed to get a more complete picture, perhaps using tools supplied by other manufacturers.
Traffic Pattern Analysis
Traffic networks equipped with inductive loop arrays or other equivalent sensors are rich sources of data about parameters such as passing vehicle speed and volume. Such data sets, especially when collected from several distinct geographic sites, are extremely difficult to analyze due to the causal relationships in both space and time that drive traffic system behavior. Consequently, many groups have been interested in research with the general objective of using neural networks to discover patterns within this data, and there is a wide range of applications in this field.
Traffic Forecasting
Traffic prediction falls into two separate categories. Strategic forecasting is where an attempt is made to predict the flow of traffic into the future for months or years and typically affects major road planning decisions. Short- term forecasts, on the other hand, often have a horizon of just a few minutes and are likely to feed directly into traffic control systems. In both strategic and short-term, neural networks were used. In both cases, promising results will be reported.
The latter case has a particularly interesting theme because it makes considerable effort to compare the neural networks with the modeling of the ARIMA time series. There are several different metrics of fitness and it becomes obvious that the “right” method depends on how you choose to calculate the outcome. The findings of this study differ from other analyses using hedonic regression.
Nowadays, transport is much more important than getting people and goods from point A to B. In order to move the flow of goods within the economy, multinational corporations and international trade rely heavily on transport services. Technological advances have made it possible for the logistics and transport industries to improve the quality of their services. Leading automotive producers and technology firms are designing fully Autonomous Vehicles (AVs) for use as taxis, commercial transport, private transport and more in a heated contest. AI-led expertise has prepared the transportation industry for immense disruption. Artificial Intelligence has become nearly synonymous with engineering in the era of digitization. Ultimately, AI’s future looks very promising in the transportation industry. We are expected to have 10 million self-driving vehicles and over 250 million smart cars by 2020.
The transport industry is going to be more data-driven and therefore more structured. Innovative enormous steps have contributed to some interesting and exciting developments for the transport industry. Whether it’s a train, plane or automobile, all of our connected devices yield tremendous amounts of data that we can use to enhance safety and efficiency across the transport system. Clearly, times are changing when it comes to the transportation environment. From tracking traffic and optimizing delivery routes, to payment processing and real-time data- based insurance premiums, data is the fuel that will provide tremendous insights to improve the transport system. The transportation system, powered by big data, artificial intelligence, and the internet of things, can become safer, more effective, and smarter.
Autonomous vehicles, effective traffic management systems, and the elimination of outdated, inefficient static hardware systems are the future of AI in transportation. Autonomous vehicles will help reduce traffic, increase safety, and increase accessibility for all vehicle transportation. Metropolitan cities can easily implement traffic management techniques that sustain the flow of traffic and industry. Municipalities will move away from traditional hardware and use AI to predict traffic, toll, identify vehicles, and more. The transportation possibilities AI brings are truly endless.
This article is co-authored by Prof Raul Villamarin Rodriguez, Ahani Ghoshal, Jaydeep Das and Prerna Singh, Universal Business School.