Unlocking Data-Driven Intelligence And Innovation Through Artificial Intelligence
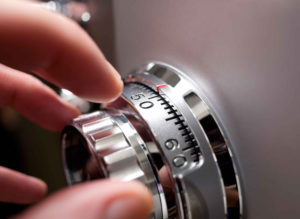
In the second of a two-part blog, you’ll learn how companies are successfully using artificial intelligence and how to take advantage of these new capabilities.
The addition of artificial intelligence (AI) and machine learning (ML) to systems opens new opportunities to take over business processes. In the past, machine learning (ML) had to be hand-coded and hardwired; if a change to an algorithm was necessary, the process had to stop and be rewritten.
Today, coding is easier and ML algorithms are available as a service or an API. Embedding intelligent technologies like AI and ML within business systems makes that processes become more intelligent and can take over repetitive work tasks that used to take hours and do them in minutes. And as systems become more intelligent, it is much easier to digitally integrate them through data, robotic process automation (rpa) and machine learning. As with each technological innovation, this opens the door to a different management approach through technology application and new service paradigms to the challenge of improving business performance; how to create the intelligent enterprise.

In order to create an intelligent enterprise, in reality, business ecosystems will need to change, and there must be a continuing evolution in business models. Consider the example of new business models for ride-sharing and usage-based auto insurance. As envisioned by a company like Lyft or Uber, automated processes are not only an acceleration of processes, they are also about adding flexibility to the overall service whilst they have changed the business model of asset ownership or employment relation.
Most work tasks are accomplished through a combination of automation and manual tasks. Manual tasks require human intervention or decisions, and seamless parts are automated. The connections between manual and automated tasks may have weaknesses and are often labor-intensive and time-consuming.
For example, in manufacturing, due to geopolitical changes and changing trade agreements, a supply chain manager recently told me how with every change, they need to rapidly find and source different suppliers to supply their components within a few weeks, so their production does not come to a standstill or impact their margin. Typically this search and assessment process used to be a one-off that would take months, but speed isn’t the only consideration; it’s also about increasing my company’s flexibility in a changed world.
These kinds of challenges justify a good look at existing business processes that may contain vulnerabilities or weak links. One needs especially to examine areas that touch their customer. Can I remove a step and eliminate a repetitive task with AI? This is the beginning of how AI and ML can support human decision-making.
The mythical “moonshot promise”
The “moonshot promise” is a strategic misconception that AI and ML are for things that will solve giant problems and contribute to full industry disruption.
Take the Uber app. It didn’t change taxi travel; rather, it simplified the process. Consumers weren’t waiting for a new whole taxi process but were really happy when Uber created an app to:
- Summon a cab
- Show us where it was
- Facilitate payment without delay
It simply took two steps out of the old process and infused some ML. In fact, contrary to the “moonshot promise” of new technology, most people want to do the same things they have always done, only faster, cheaper, and with much more data.
The impact of AI: AI will impact employers before employees
While it sounds counterintuitive, employers will likely be affected more by AI than employees because of the competitive differences it offers – but they will have to make changes to take advantage. In the United States, 22% of companies that have published an annual report in recent months have allocated parts of their profits to AI.
According to a recent study which SAP conducted in conjunction with the Economist Intelligent Unit on AI adoption, the organizations doing the most with machine learning (the “Fast Learners”) have experienced 43% more growth on average than those who either haven’t started using AI and ML or who aren’t using it well. Using new technology is not a recipe for success, just like owning a sailboat does not mean you will win the race.
While AI is becoming core to business strategy, data governance and data management remain a persistent obstacle. Here, leadership is key, and leading organizations find that the first step on a successful AI journey is to improve data management. No matter how great the algorithm, bad training data will limit the effectiveness of AI and ML. Transparency, data quality, ownership, and governance make all the difference for AI and ML success.
After all, new technology requires new rules. We need design principles that focus on providing transparency on how AI came to its answer and ones that focus on proactively dealing with regulatory and ethical dilemmas. We need to know: Who is going to pay attention to managing data? How does AI come to this decision? Who trained the system? What data is the basis? What confidence does the AI have in the data quality and relevance? Do I trust this decision?
Innovate for success
A smart approach is to take a business problem that is specific to one customer, find the elements of that problem that are common across the industry, then define the elements of a methodology and the technologies used to solve that problem. Packaging them and making them available as industry innovation kits or embedding them into other applications helps other customers more easily address common challenges.
Business solutions need to provide intelligent technologies for business processes to create better outcomes. A business solution comprised of a pre-orchestrated tech stack, enabled by best practices and process blueprints, can solve business problems and complement the core processes and the tasks people manage every day. For processes that are difficult to complete, new technologies like ML and AI can help automate them.
Some key requirements:
- Do you have the training data or the business examples to train your AI & ML?
- Can you afford for your best business process experts to make their time available to train the ML in your secret sauce or how you do things?
- Is the strategy clear and focused on scaling knowledge augmentation across the enterprise? The goal should be to implement ML initiatives enterprise-wide, rather than within individual business units or functions.
Leverage design thinking
Design thinking best practices take an outside-in, user-led approach to uncover insights, co-create ideas, and accelerate new technological innovations to market. It’s all about open innovation and rapid-design development. While other innovation processes take two to four years, design thinking can deliver results in weeks with fewer risks. The risk-reward balance leans heavily toward reward, as participants can walk out of a design thinking workshop with tenable concepts that prove their time and money was not wasted.
A case study
- Vale, a large metals and mining company in Brazil, applied design thinking to improve mine maintenance and operations. After going through the design thinking process, the team identified the issue of reducing the number of rejected purchase requisitions from services technicians.
- In just four weeks, Vale designed a user-centric solution that optimized the purchase, requisition, and maintenance process, reducing the rejection rate by 86% using a prototype based on machine learning.
During the past year, we’ve seen how the design-thinking approach and industry best practices are key to successes in discrete manufacturing, energy and natural resources, and consumer industries. Some recent examples of successes:
- Reducing the number of times customers need to call a support line to fix issues because the technologies can now self-analyze and fix problems
- Reducing downtime by 40% because equipment can predict and self-heal failures
- Decreasing costs by as much as 68% by integrating AI and cloud computing during the manufacturing process
- Increasing employee retention with the help of analytics and ML
Personally, I see ML within AI as the most powerful components organizations can use because they directly reduce repetitive work and take care of the heavy lifting. Some, like the Fast Learners in the machine learning report, are already profiting from their first initiatives that infuse ML into their processes. Others are creating new business models and improving operations with IoT, blockchain, or predictive analytics.
It’s time for all organizations to start thinking about how AI and machine learning fit into a strategy for digital transformation.
If you’d like to apply AI, ML, or any of the other intelligent technologies, begin preparing and sharpening your strategy with SAP Leonardo and design thinking.
Follow Marc on Twitter to track all the latest news about digital innovation, design thinking, and SAP Leonardo.