UPMC’s Revenue Cycle Leader: A Gradual Evolution into AI-Facilitated RCM Work | Healthcare Innovation
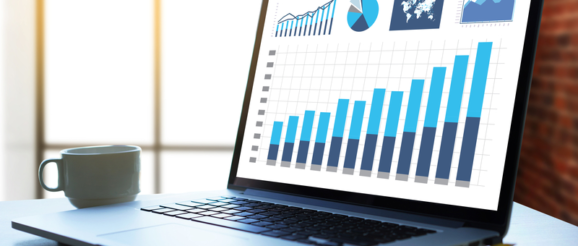
Revenue cycle management (RCM) operations continue to evolve forward in U.S. hospitals, medical groups, and health systems, through a combination of advancing technologies and enhanced strategies implemented by finance leaders in patient care organizations. Among the methods that have evolved forward have been business process automation (BPA)—also known as robotic process automation (RPA)—a set of processes that has been successfully incorporated into core RCM processes. Emerging recently have been the earliest attempts to leverage machine learning- and artificial intelligence (AI)-based technologies to achieve what is being called predictive denials management. Essentially, this involves the development of algorithms based on data analytics, that can trigger interventions based on anticipated insurance claims denials.
That evolution was the subject of one of the Ten Transformative Trends that the editors at Healthcare Innovation examined in the publication’s March/April issue. In an article entitled “The New Revenue Cycle Management,” Healthcare Innovation Editor-in-Chief Mark Hagland interviewed a variety of industry leaders regarding the rapid advances taking place now in revenue cycle management, which has become an absolutely critical function for hospitals, medical groups, and health systems, as their leaders attempt to weather the financial storm that has come in the past year with the emergence of the COVID-19 pandemic in the United States, and its impact on patient care organizations nationwide.
One of the industry experts whom Hagland interviewed for that Trend article was Jeffrey Porter, vice president of revenue cycle and chief revenue cycle officer at the 40-plus-facility, Pittsburgh-based UPMC health system. Below are excerpts from the interview that Hagland conducted with Porter in February.
Let’s begin by my asking you about the size of your team. How many individuals do you have on your staff altogether?
About 2,500. That total number covers a lot of different functions. We have a centralized scheduling team, and a contact center with about 500 people who schedule patient appointments. I have a care management team doing utilization review.
How many among that total are engaged in doing revenue cycle management?
It breaks down this way: out of the 2,500 total, 500 are employed in the contact center, doing scheduling and responding to billing inquires; 500 are involved in the patient access area, 500 or so are in the coding space—we do all the physician and hospital coding for all of our entities. And another 200 are working in the care management space. And then the rest would be managing claims, as well as our support service team, where the data scientists are. So in other words, about 800 individuals out of that total number of 2,500 on our team, are directly involved in revenue cycle management.
Others I’ve interviewed for our Trend article have noted that, while most hospital-based revenue cycle management teams have been involved in business process automation or robotic process automation for some time now, few have actually advanced into the area of predictive denials management. Would you agree?
Yes, I agree 100 percent with that assessment, that you really have to discern between RPA or web-bot-ing, where you’re automating a process, and the idea of moving into more of an AI- and machine learning-based model that can predict with high accuracy the likelihood of a claim being denied. And we’re not there yet, but that’s where we’re planning to go. That’s more cutting edge. In fact, RPA is pretty prevalent now, we’ve been doing automation-based work for about a decade now. Back when we started it, we called it screen scraping, then web-bot-ing, then RPA. It’s basically using technology to support what would be a manual process. It’s high-volume around transactions, where you want to optimize your staff’s work.
BPA—robotic process automation—is the same thing. It became prevalent a decade or more ago, when payers pushed everything onto portals, all the administrative activity, where you had to go and find out the claims status—paid, denied, pending. Once payers had done all of that, they made it ripe for providers to emulate that process through technology.
What steps will you be taking in advancing toward predictive denials management, and what might the timeframe be like, for your organization?
It will be incremental. First, you have to invest in the people—you need actual data scientists, who have a high technical capability. And those people are difficult to get, and are expensive. So, we’ll approach this in two ways: first, through predictive denials management. And second, we’re also looking at our patient segmentation, so, understanding patient payment patterns and behaviors. Who pays and how, for example, on the first bill, second bill, etc. We want to create a better patient experience around this. We’re trying to understand how we can build a model that studies that. There are technologies out there that build, refine, test, and further refine those models. Modeling patient payment behavior.
So predictive denials management comes first, and then the science of understanding patient payment behavior?
Yes, that’s correct; this could be expanded over time to numerous areas. First, there’s the set of processes around understanding the likelihood of denial versus payment per individual payer. We’re beginning to model that out, and to test that modeling out.
So you’re laying the foundations now?
Yes, with the technology and the people. And the people are hard to find and are expensive. Revenue cycle historically involved large numbers of people, but who were usually closer to entry-level people. In fact, we’ve spent more than a decade using BPA/RPA technologies to automate what had largely been manual work, since payers pushed all the administrative activity onto portals. So we’ll be moving into AI-assisted revenue cycle management within a couple of years, but in an evolutionary way. We started BPA a decade ago in one avenue, claims statusing. We knew we had staff members going onto those portals all day long. So we automated those processes.
The challenge we have is that the payers are looking to reduce unnecessary utilization, and they’re putting in more requirements around documentation and authorization, and are intensifying their utilization management. To get to the point where we’re using it in a practical sense—well, I think that within a year, we’ll have this helping our organization, where it’s functional and scalable and showing an impact, and repurposing staff to do other kinds of work that provide a higher yield for us. And that’s true, too, for patients, as we learn their past history and preferences, to meet their needs and our needs. There are a lot of different benefits to this, including increased revenue, as well as accommodating all the new payer policies coming out, and improving our interactions with patients, that will be within a two-to-three-year time period.
What advice might you be able to offer your peers in RCM departments in other patient care organizations?
We’ve got two experts leading this work; they’re data scientists with advanced degrees from Carnegie-Mellon. We call them business optimization experts. One came to us from outside healthcare, and one had been working in our organization. And the thing is that you’ve got to marry the knowledge of these data scientists with the expertise of your current revenue cycle team. The data scientists can really understand the modeling, but need to understand how things impact the operations. Sometimes, that marriage is hard, it’s a work in progress; it involves very different backgrounds. But invest in the people who can manage the technology. That’s the piece we’ve done, and we’re marrying it with the operational expertise, to derive benefits.
We’ve started on a small scale; it’s not yet AI. We manage patient access for 30-plus hospital emergency departments, and it’s 24/7 work with three shifts a day. And the ED is in itself unpredictable, but when you run it through predictive analytics tools, you can predict how many people will come into the ED. We had been over-staffing for every shift, but now we predictive-model it. We call people mobile access staff. People dedicated to working with patients coming into the ED. And they’ll work at Presbyterian. And then you have COVID quarantines. And you have people getting ill. And snow days. And unexpected high volumes. So we created an overflow pool of people who could be deployed based on need, and we used predictive modeling to create that staff. They’re staff members who can travel from one facility to another. And by doing that, we were able to avoid over-hearing in every hospital, yet manage all the needed staff.
And you’ll apply that predictive modeling to RCM?
Yes; we want to take that further with denial predictions and processing.
What advice might you share with colleagues from less-well-resourced hospitals?
I think the BPA technology is out there, and there are vendors who will bring real value propositions to them. It’s probably going to be a challenge to develop the next frontier in machine learning and AI. We have a small team of people doing this; it’s not an army of people, but it will pay off for us in the long run. Those same organizations may not even have BPA in yet. Almost all of our BPA is done internally in-house.