When Innovation Becomes Magic | Human-Centered Change and Innovation
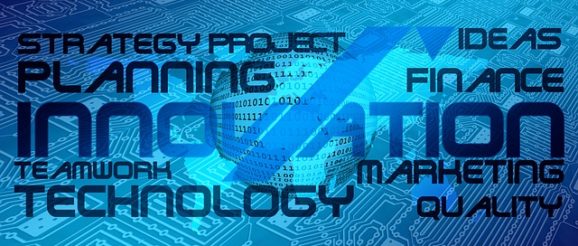
GUEST POST from Pete Foley
Arthur C Clarke’s 3rd Law famously stated:
In other words, if the technology of an advanced civilization is so far beyond comprehension, it appears magical to a less advanced one. This could take the form of a human encounter with a highly advanced extraterrestrial civilization, how current technology might be viewed by historical figures, or encounters between human cultures with different levels of scientific and technological knowledge.
Clarke’s law implicitly assumed that knowledge within a society is sufficiently democratized that we never view technology within a civilization as ‘magic’. But a combination of specialization, rapid advancements in technology, and a highly stratified society means this is changing. Generative AI, Blockchain and various forms of automation are all ‘everyday magic’ that we increasingly use, but mostly with little more than an illusion of understanding around how they work. More technological leaps are on the horizon, and as innovation accelerates exponentially, we are all going to have to navigate a world that looks and feels increasingly magical. Knowing how to do this effectively is going to become an increasingly important skill for us all.
The Magic Behind the Curtain: So what’s the problem? Why do we need to understand the ‘magic’ behind the curtain, as long as we can operate the interface, and reap the benefits? After all, most of us use phones, computers, cars, or take medicines without really understanding how they work. We rely on experts to guide us, and use interfaces that help us navigate complex technology without a need for deep understanding of what goes on behind the curtain.
It’s a nuanced question. Take a car as an analogy. We certainly don’t need to know how to build one in order to use one. But we do need to know how to operate it and understand what it’s performance limitations are. It also helps to have at least some basic knowledge of how it works; enough to change a tire on a remote road, or to have some concept of basic mechanics to minimize the potential of being ripped off by a rogue mechanic. In a nutshell, the more we understand it, the more efficiently, safely and economically we leverage it. It’s a similar situation with medicine. It is certainly possible to defer all of our healthcare decisions to a physician. But people who partner with their doctors, and become advocates for their own health generally have superior outcomes, are less likely to die from unintended contraindications, and typically pay less for healthcare. And this is not trivial. The third leading cause of death in Europe behind cancer and heart disease are issues associated with prescription medications. We don’t need to know everything to use a tool, but in most cases, the more we know the better
The Speed/Knowledge Trade-Off: With new, increasingly complex technologies coming at us in waves, it’s becoming increasing challenging to make sense of what’s ‘behind the curtain’. This has the potential for costly mistakes. But delaying embracing technology until we fully understand it can come with serious opportunity costs. Adopt too early, and we risk getting it wrong, too late and we ‘miss the bus’. How many people who invested in crypto currency or NFT’s really understood what they were doing? And how many of those have lost on those deals, often to the benefit of those with deeper knowledge? That isn’t to in anyway suggest that those who are knowledgeable in those fields deliberately exploit those who aren’t, but markets tend to reward those who know, and punish those who don’t.
The AI Oracle: The recent rise of Generative AI has many people treating it essentially as an oracle. We ask it a question, and it ‘magically’ spits out an answer in a very convincing and sharable format. Few of us understand the basics of how it does this, let alone the details or limitations. We may not call it magic, but we often treat it as such. We really have little choice; as we lack sufficient understanding to apply quality critical thinking to what we are told, so have to take answers on trust. That would be brilliant if AI was foolproof. But while it is certainly right a lot of the time, it does make mistakes, often quite embarrassing ones. . For example, Google’s BARD incorrectly claimed the James Webb Space Telescope had taken the first photo of a planet outside our solar system, which led to panic selling of parent company Alphabet’s stock. Generative AI is a superb innovation, but its current iterations are far from perfect. They are limited by the data bases they are fed on, are extremely poor at spotting their own mistakes, can be manipulated by the choice of data sets they are trained on, and they lack the underlying framework of understanding that is essential for critical thinking or for making analogical connections. I’m sure that we’ll eventually solve these issues, either with iterations of current tech, or via integration of new technology platforms. But until we do, we have a brilliant, but still flawed tool. It’s mostly right, is perfect for quickly answering a lot of questions, but its biggest vulnerability is that most users have pretty limited capability to understand when it’s wrong.
Technology Blind Spots: That of course is the Achilles Heel, or blind spot and a dilemma. If an answer is wrong, and we act on it without realizing, it’s potentially trouble. But if we know the answer, we didn’t really need to ask the AI. Of course, it’s more nuanced than that. Just getting the right answer is not always enough, as the causal understanding that we pick up by solving a problem ourselves can also be important. It helps us to spot obvious errors, but also helps to generate memory, experience, problem solving skills, buy-in, and belief in an idea. Procedural and associative memory is encoded differently to answers, and mechanistic understanding helps us to reapply insights and make analogies.
Need for Causal Understanding. Belief and buy-in can be particularly important. Different people respond to a lack of ‘internal’ understanding in different ways. Some shy away from the unknown and avoid or oppose what they don’t understand. Others embrace it, and trust the experts. There’s really no right or wrong in this. Science is a mixture of both approaches it stands on the shoulders of giants, but advances based on challenging existing theories. Good scientists are both data driven and skeptical. But in some cases skepticism based on lack of causal understanding can be a huge barrier to adoption. It has contributed to many of the debates we see today around technology adoption, including genetically engineered foods, efficacy of certain pharmaceuticals, environmental contaminants, nutrition, vaccinations, and during Covid, RNA vaccines and even masks. Even extremely smart people can make poor decisions because of a lack of causal understanding. In 2003, Steve Jobs was advised by his physicians to undergo immediately surgery for a rare form of pancreatic cancer. Instead he delayed the procedure for nine months and attempted to treat himself with alternative medicine, a decision that very likely cut his life tragically short.
What Should We Do? We need to embrace new tools and opportunities, but we need to do so with our eyes open. Loss aversion, and the fear of losing out is a very powerful motivator of human behavior, and so an important driver in the adoption of new technology. But it can be costly. A lot of people lost out with crypto and NFT’s because they had a fairly concrete idea of what they could miss out on if they didn’t engage, but a much less defined idea of the risk, because they didn’t deeply understand the system. Ironically, in this case, our loss aversion bias caused a significant number of people to lose out!
Similarly with AI, a lot of people are embracing it enthusiastically, in part because they are afraid of being left behind. That is probably right, but it’s important to balance this enthusiasm with an understanding of its potential limitations. We may not need to know how to build a car, but it really helps to know how to steer and when to apply the brakes . Knowing how to ask an AI questions, and when to double check answers are both going to be critical skills. For big decisions, ‘second opinions’ are going to become extremely important. And the human ability to interpret answers through a filter of nuance, critical thinking, different perspectives, analogy and appropriate skepticism is going to be a critical element in fully leveraging AI technology, at least for now.
Today AI is still a tool, not an oracle. It augments our intelligence, but for complex, important or nuanced decisions or information retrieval, I’d be wary of sitting back and letting it replace us. Its ability to process data in quantity is certainly superior to any human, but we still need humans to interpret, challenge and integrate information. The winners of this iteration of AI technology will be those who become highly skilled at walking that line, and who are good at managing the trade off between speed and accuracy using AI as a tool. The good news is that we are naturally good at this, it’s a critical function of the human brain, embodied in the way it balances Kahneman’s System 1 and System 2 thinking. Future iterations may not need us, but for now AI is a powerful partner and tool, but not a replacement
Image credit: Pixabay
Sign up here to join 17,000+ leaders getting Human-Centered Change & Innovation Weekly delivered to their inbox every week.