Where Innovation Is Happening in Geolocation. Part 1: Signal Processing
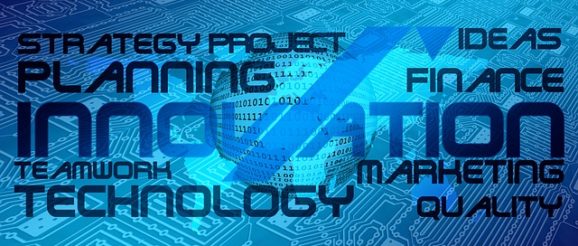
By Rabih Chrabieh, CTO, Nestwave
At first glance, geolocation technology seems to work well. Our phones and cars get us to where we need to be with little effort and rather reliably. For consumers, it’s a free service, thanks to service providers like Google and Apple.
Peel back a layer and things are more complex. For one, even smartphone and in-vehicle navigation systems are not perfect. Ever try to start a route from inside a parking garage? While walking on a city sidewalk, has your map app located you on the wrong block, or perhaps inside a building? How’s your battery life when you’re running navigation?
These challenges multiple when faced with the demands of IoT applications. IoT use cases in smart city and smart factory deployments strain the current class of consumer solutions in areas including ubiquitous accuracy [1], power consumption and cost. Despite advances in satellite (GNSS) and terrestrial (4G, BLE and WiFi) geolocation technology, existing technology and solutions are often not up to the task.
At Nestwave, we’ve been innovating in numerous technical areas in an effort to move the needle. In Part 1 of this blog, we’ll be covering advances in a process that occurs deep inside a geolocation chipset: the signal processing used to determine the time of arrival of a wireless positioning signal.
The operation of satellite and many terrestrial geolocation systems begins with the transmission of wireless positioning signals between nodes of known location and an object of unknown location. A time of arrival (TOA) is determined and then converted into a distance from the transmitter, via velocity of the wave and clock synchronization. Multilateration is then used to calculate the position of an object based on the distance to the nodes of known location.
In a perfect world, a positioning signal would be transmitted at time t0 as a pure impulse or having a front rising edge of infinite vertical slope, propagate through a lossless, impairment-free medium and be received with no distortion. Establishing an accurate TOA would be rather straightforward.
In reality, signals are transmitted with finite slopes and are further bandwidth limited upon reception. They attenuate over distance, and critically, are distorted by atmospheric impairment, signal blockage, non-line-of-sight propagation and superimposed signal reflections due to multipath.
Take a quick glance at Figure 1. The red and blue traces show the received positioning signal transmitted from two antennas at a single location. When did each arrive? Maybe at the signal peak? Which peak? You’d be wrong. The green reference signal establishes “zero” on this Figure, showing the actual TOA (or in this case, the equivalent distance). It’s quite easy to see that ranging errors on the order of dozens of meters is possible in the presence of impairments such as multipath.
Figure 1: Real-world reception of positioning signals
Nestwave has developed a new approach to determining TOA using unique methods of signal filtering and estimation to improve accuracy in the presence of signal impairments and multipath.
Let’s start by looking at signal filtering. In conventional systems, received signals are often filtered using a symmetrical matched filter (MF). The impulse response of such filters is typically a sinc function. MF solutions can optimize data symbol detection in noisy/multipath environments where information is contained (and useful) in both direct and multipath signal components. However, for TOA estimation, we want to eliminate the energy contained in multipath components – not use it.
Nestwave has developed a near-causal filtering approach which enables better identification of the direct positioning signal path. In Figure 2 below, the Nestwave filter (blue) exhibits a taller, steeper rising filter edge as compared to a conventional sinc (yellow). When presented a signal with large multipath energy, the Nestwave filter results in a “cleaner” and more distinct signal rising edge.
Figure 2: Nestwave near-causal filter vs typical matched filter
Once we have a filtered signal, we need to estimate the TOA. Conventional techniques are either inadequate in dense multipath and NLOS environments, or for the cases of maximum-likelihood (ML) and MUSIC algorithms, unreliable and impractical due to the intense processing requirements. Nestwave has developed several advanced, robust estimation solutions which result in near-ML optimality but with reduced complexity in dense multipath.
A key to our approach is to simplify how we treat the multipath components as determined by actual (or expected) channel conditions, e.g. a power-delay profile. We sort multipath components into two classes: (1) nuisance multipaths that require joint estimation and (2) multipaths that can be treated as colored noise. By reducing the number of paths (= direct path + nuisance paths) for estimation, this approach exponentially reduces the complexity of ML computation with little loss of accuracy.
Let’s see how this all comes together in practice. Nestwave ran field testing in downtown San Francisco, an environment well known for substantial multipath. Indoor and outdoor locations were sampled according to the paths shown in Figure 3 below. In total, 7,000 sample points were collected across (300) 4G cells.
Figure 3: Indoor and outdoor test locations in San Francisco
We applied three different filtering methods to the received signals: (1) a conventional sinc-filter design (2) a Dolph-Chebyshev filter, known for high performance in the presence of multipath and (3) Nestwave’s near-causal filter design.
As can be seen in the cumulative distribution function (CDF) shown in Figure 4, the Nestwave solution outperformed the other two solutions, often substantially. At the 80% mark, the Nestwave ranging error is one-half of Dolph-Chebyshev and less than one-seventh the error of a conventional sinc filter solution.
Figure 4: Cumulative distribution function of ranging error comparing Nestwave with conventional solutions
Putting it all together, better filtering and estimation techniques can be used to improve geolocation accuracy and reduce power consumption even when faced with real-world impairments such as dense multipath and NLOS conditions.
As IoT deployments grow and applications expand, these benefits will be critical for chipset and product manufacturers looking to provide the best performance and value to their customers.
Rabih is the head of technology and system architecture, designing and developing Nestwave’s ultra-low-power indoor/outdoor geolocation solution for the Internet of Things (IoT). He has more than 25 years of experience in the wireless industry at companies such as Sequans, Qualcomm and ArrayComm, designing and architecting solutions for 3G, 4G and UWB systems. He also co-founded Softwave Wireless which developed a wireless network planning tool and was recently acquired by a major player in the Silicon Valley. Rabih is an inventor on more than 30 patents issued and pending.
[1]anywhere and everywhere including urban canyon, underground and in-building.
If you wish to download a copy of this white paper, click here
Contact Nestwave
Your Name: | |
Your E-mail address: |
|
Your Company address: |
|
Your Phone Number: | |
Write your message: | |