Will Smart Machines Be Less Biased Than Humans? – Center for Data Innovation
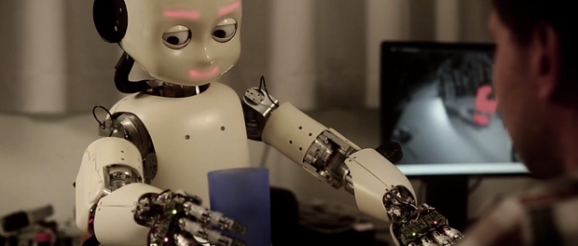
There is no question that, by their very nature, artificial intelligence (AI) systems are more complex than traditional software systems where the parameters were built in and largely understandable. It was relatively clear how the older rules-based expert systems made decisions.
In contrast, machine learning allows AI systems to continually adjust and improve based on experience. Now different outcomes are more likely to originate from obscure changes in how variables are weighted in computer models.
This has led some critics to claim that this complexity will result in systemic “algorithmic bias” that enables government and corporate abuse. These critics fall into two camps. The first camp believes that companies or governments will deliberately “hide behind their algorithm,” using their algorithm’s opaque complexity as a cover to exploit, discriminate or otherwise act unethically. For example, Tim Hwang and Madeleine Clare Elish, of nonprofit organization Data & Society, claim that Uber deliberately uses its surge pricing algorithm to create a “mirage of a marketplace” that does not accurately reflect supply and demand so Uber can charge users more. In other words, they are saying the Uber algorithm is a sham that gives cover to unfair pricing policies.
The second are those, such as Frank Pasquale, author of The Black Box Society: The Secret Algorithms That Control Money and Information, who argue that opaque, complicated systems will allow “runaway data” to produce unintended and damaging results. For example, Cathy O’Neil, author of the forthcoming book with the catchy title Weapons of Math Destruction: How Big Data Increases Inequality and Threatens Democracy, describes how machine-learning algorithms are likely to be racist and sexist because computer scientists typically train these systems using historical data that reflects societies’ existing biases. Likewise, Hannah Devlin asks in the Guardian, “would we be comfortable with a[n AI] diagnostic tool that saved lives overall, say, but discriminated against certain groups of patients?”
To be sure, AI, like any technology, can be used unethically or irresponsibly. But resistance to AI because of this concern fails to recognize a key point: AI systems are not independent from their developers and, more importantly, from the organizations using them. For example, once malicious Internet users taught Microsoft’s experimental AI chatbot to spew hate speech on the Internet, the company pulled the plug on that particular project. If a government wants to systematically discriminate against certain groups of its citizens, it doesn’t need AI to do so.
Furthermore, if an algorithmic system produces unintended and potentially discriminatory outcomes, it’s not because the technology itself is malicious; it’s because it simply follows human instructions, or more often relies on data sets from the world that may reflect bias. Quite simply, these systems will reflect human intention. As famous scientist and author Lawrence Krauss writes:
“…We have not lost control because we create the conditions and initial algorithms that determine the decision-making. I envisage the human-computer interface as like having a helpful partner, and the more intelligent machines become the more helpful they can be partners. Any partnership requires some level of trust and loss of control, but if the benefits often outweigh the losses, we preserve the partnership. If they don’t, we sever it. I see no difference if the partner is a human or a machine.”
David Mindell, professor of the history and engineering of manufacturing at the Massachusetts Institute of Technology, agrees in his book, Our Robots, Ourselves, writing:
“For any apparently autonomous system, we can always find the wrapper of human control that makes it useful and returns meaningful data. To move our notions of robotics and automation, and particularly the newer idea of autonomy, into the twenty-first century, we must deeply grasp how human intentions, plans, and assumptions are always built into machines. Even if software takes actions that could not have been predicted, it acts within frames and constraints imposed upon it by its creators. How a system is designed, by whom, and for what purpose shapes its abilities and its relationships with people who use it.”
Nonetheless, many critics seem convinced that the complexity of these systems is responsible for any problems that emerge, and that pulling back the curtain on this complexity by mandating “algorithmic transparency” is necessary to ensure that the public can police against nefarious corporate or government attempts to use algorithms unethically or irresponsibly. Data and algorithmic transparency, as defined by the Electronic Privacy Information Center (EPIC): “Entities that collect personal information should be transparent about what information they collect, how they collect it, who will have access to it, and how it is intended to be used. Furthermore, the algorithms employed in big data should be made available to the public.”
Combatting bias and protecting against harmful outcomes is of course important, but mandating that companies make their propriety AI software publicly available would not actually solve these problems and would create other problems. Moreover, the economic impact of such a mandate would be significant, as it would prevent companies from capitalizing on their intellectual property and future investment and research into AI would slow. Other companies would simply copy their algorithms.
Calls for algorithmic transparency hinge on a glaring logical inconsistency. Algorithms are simply a recipe for decision-making, so if proponents of algorithmic transparency really worry that these decisions are harmful, then they should also call for all aspects of all decision-making to be public.
Maybe CEOs and other top decision makers should be required to take detailed psychological tests to determine their biases? That advocates do not call for such disclosures indicates that such proponents must think regular, human decisions are already transparent, fair, and free from the subconscious and overt biases we know permeate every aspect of society and the economy. This is of course wrong. And as Daniel Kahneman writes in his book Thinking, Fast and Slow, human irrationality and bias is inherent in being human.
Equally confounding, these critics acknowledge the ability for AI systems to be incredibly complex and effective but fail to think that companies and governments can responsibly embed ethical principles into algorithmic systems. Damned if they do; damned if they don’t.
To embed such principles, author Nicholas Carr laments in his book The Glass Cage: Automation and Us, “We’ll need to become gods.” He asks, “Who gets to program the robot’s conscience? Is it the robot’s manufacturer? The robot’s owner? The software coders? Politicians? Government regulators? Philosophers? An insurance underwriter?” But this ignores the fact that the body politic already “plays god” in most decisions governments make.
We decide as a society that a certain number of road fatalities a year is acceptable, because we choose not to spend more on higher priced, but more safely designed roads.
We say it is okay for people to die from treatable diseases because we choose not to spend even more on health care.
But by definition, societal resources are scarce relative to needs, and decisions must be made on a regular basis about how to allocate these resources among competing uses. And yes, these are “godlike,” in that they will result in some people being harmed. But absent massive increases in productivity, societies will have to do the best they can at managing and minimizing the tradeoffs. Programming smart machines will be no different.
Fortunately, many have recognized that embedding ethical principles into machine-learning systems is both possible and effective for combatting unintended or harmful outcomes. In May 2016, the White House published a report detailing the opportunities and challenges of big data and civil rights, but rather than focus on demonizing the complex and necessarily proprietary nature of algorithmic systems, it presented a framework for “equal opportunity by design”—the principle of ensuring fairness and safeguarding against discrimination throughout a data-driven system’s entire lifespan. This approach, described more generally by Federal Trade Commissioner Terrell McSweeny as “responsibility by design,” rightly recognizes that algorithmic systems can produce unintended outcomes, but doesn’t demand a company waive rights to keep its software proprietary.
Instead, the principle of responsibility by design provides developers with a productive framework for solving the root problems of undesirable results in algorithmic systems: bad data as an input, such as incomplete data and selection bias, and poorly designed algorithms, such as conflating correlation with causation, and failing to account for historical bias.
Moreover, researchers are making progress in enabling algorithmic accountability. For example, Carnegie Mellon researchers have found a way to help determine why a particular machine-learning system is making the decisions it’s making, without having to divulge the underlying workings of the system or code.
It also is important to note that some calls for algorithmic transparency are actually more in line with the principle of responsibility by design than with EPIC’s definition. For example, former chief technologist of the Federal Trade Commission (FTC) Ashkan Soltani said that although pursuing algorithmic transparency was one of the goals of the FTC, “accountability” rather than “transparency” would be a more appropriate way to describe the ideal approach, and that making companies surrender their source code is “not necessarily what we need to do.”
Figuring out just how to define responsibility by design and encourage adherence to it warrants continued research and discussion, but it is crucial that policymakers understand that AI systems are valuable because of their complexity, not in spite of it.
Attempting to pull back the curtain on this complexity to protect against undesirable outcomes is counterproductive and threatens the progress of AI and machine learning as a whole.
This is an excerpt from the Information Technology and Innovation Foundation’s report, “’It’s Going to Kill Us!’ and Other Myths about the Future of Artificial Intelligence.” This excerpt was originally published in Brink.
Image: Dalle Molle Institute for Artificial Intelligence Research.