World History – Work – Lives/Contributions – Life – Technologies – Human Longevity – Science, Technology and Innovation – Time – Data – World @ Rodrigo Nunes Cal
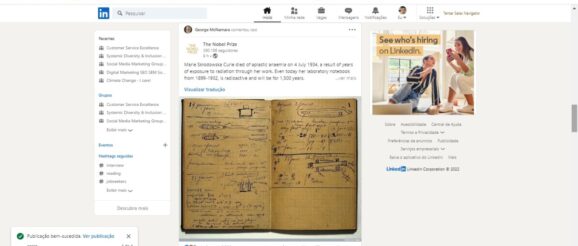
1. Cancer is very related to the weight loss of the patient.
2. Age, weight and genetics of the person are very important factors that influence cancer in a determined ways.
3. The genetics of the mouse is very similar to that of the human.
4. Maintaining proper body weight is one of the main ways to prevent cancer of a person.
5. Animal testing has a very high importance to world society.
6. The mouse is the main animal model used as the basis for research on diseases that affect humans.
– Informacoes-relevantes-relacionadas-a-leitura-de-livros-e-seus-aspectos-interligados-no-ambiente-escolar-rodrigo-nunes-cal-parte-1 @ Relevant-information-related-book-reading-and-its-interconnected-aspects-in-the-school-environment – Very important links to download eBooks for free – Links muito importantes para baixar e-Books gratuitamente
– Informacoes-relevantes-relacionadas-a-leitura-de-livros-e-seus-aspectos-interligados-no-ambiente-escolar-parte-2 @ Relevant-information-related-book-reading-and-its-interconnected-aspects-in-the-school-environment – Very important links to download eBooks for free – Links muito importantes para baixar e-Books gratuitamente
GRUPO_AF2 – ´´My´´ Dissertation – Study Group 1 – Aerobic Physical
GRUPO_AF1 – ´´My´´ Dissertation – Study Group 1 – Aerobic Physical Activity
GRUPO AFAN 2 – ´´My´´ Dissertation – Study Group 2 – Anaerobic Physical Activity
GRUPO AFAN 1 – ´´My´´ Dissertation – Study Group 2 – Anaerobic Physical Activity – National Human Genome Research Institute –> https://www.genome.gov/
´´At NHGRI, we are focused on advances in genomics research. Building on our leadership role in the initial sequencing of the human genome, we collaborate with the world’s scientific and medical communities to enhance genomic technologies that accelerate breakthroughs and improve lives. By empowering and expanding the field of genomics, we can benefit all of humankind.´´
Informacoes-relevantes-relacionadas-a-leitura-de-livros-e-seus-aspectos-interligados-no-ambiente-escolar-rodrigo-nunes-cal-parte-1 @ Relevant-information-related-book-reading-and-its-interconnected-aspects-in-the-school-environment – Very important links to download eBooks for free – Links muito importantes para baixar e-Books gratuitamente
Informacoes-relevantes-relacionadas-a-leitura-de-livros-e-seus-aspectos-interligados-no-ambiente-escolar-parte-2 @ Relevant-information-related-book-reading-and-its-interconnected-aspects-in-the-school-environment – Very important links to download eBooks for free – Links muito importantes para baixar e-Books gratuitamente
rodrigonunescal_dissert – ´´my´´ dissertation – Lung Cancer Research in Mice
CARCINÓGENO DMBA EM MODELOS EXPERIMENTAIS – DMBA CARCINOGEN IN EXPERIMENTAL MODELS
GRUPO_AF2 – ´´my´´ dissertation
Informacoes-relevantes-relacionadas-a-leitura-de-livros-e-seus-aspectos-interligados-no-ambiente-escolar-rodrigo-nunes-cal-parte-1 @ Relevant-information-related-book-reading-and-its-interconnected-aspects-in-the-school-environment – Very important links to download eBooks for free – Links muito importantes para baixar e-Books gratuitamente
Informacoes-relevantes-relacionadas-a-leitura-de-livros-e-seus-aspectos-interligados-no-ambiente-escolar-parte-2 @ Relevant-information-related-book-reading-and-its-interconnected-aspects-in-the-school-environment – Very important links to download eBooks for free – Links muito importantes para baixar e-Books gratuitamente
I n t e r n e t – Memory – Time – History – Levels of Influences – Human Mind – Human Life – Life – Vision – Reading – Reading Mind – Probabilities – Different Technologies – Different Influences – Different Imaginations – Images – information – photos – knowledge – Videos – Thoughts – Thinking – Impossible – Possible – Reality – Virtuality – Times – Health – DNA – Human DNA – Cell – Human – Human Cell – Cell Phones – Virtual Life – Real Life – No Internet – 100 – Número(s) – Internet – Listening – Different Interpretations – Speaking – 100 Internet – Sem Internet – 100 – gene – gene – Sem gene(s) – Sem DNA – 0 – 01 – 1 – I – V -X – Computer – Computer History – History of the World – Numbers – Data – Graphics – World Innovation – Future References – Future Citations – Impact Factors – Journals – Scientific Community – Discussion – Researches – Articles – DataBase – 100 – DNA – Animal Cell – Human Cell – Mysteries – No Gene – Person – Other Person – Genetics – Science – People – Types of Communication – Human Longevity #Time #Tempo(s)
– Visit and share !! Thanks in advance!! #probability #reader #time #people #countries #country #world #economy #longevity #click #clicks #cities
EDITORIAL: The editorial was written by the three editors acting as individuals and reflects their scientific views not an endorsed position of the American Statistical Association.
Some of you exploring this special issue of The American Statistician might be wondering if it’s a scolding from pedantic statisticians lecturing you about what not to do with p-values, without offering any real ideas of what to do about the very hard problem of separating signal from noise in data and making decisions under uncertainty. Fear not. In this issue, thanks to 43 innovative and thought-provoking papers from forward-looking statisticians, help is on the way.
1 “Don’t” Is Not Enough
There’s not much we can say here about the perils of p-values and significance testing that hasn’t been said already for decades (Ziliak and McCloskey 2008; Hubbard 2016). If you’re just arriving to the debate, here’s a sampling of what not to do:
Don’t. Don’t. Just…don’t. Yes, we talk a lot about don’ts. The ASA Statement on p-Values and Statistical Significance (Wasserstein and Lazar 2016) was developed primarily because after decades, warnings about the don’ts had gone mostly unheeded. The statement was about what not to do, because there is widespread agreement about the don’ts.
Knowing what not to do with p-values is indeed necessary, but it does not suffice. It is as though statisticians were asking users of statistics to tear out the beams and struts holding up the edifice of modern scientific research without offering solid construction materials to replace them. Pointing out old, rotting timbers was a good start, but now we need more.
Recognizing this, in October 2017, the American Statistical Association (ASA) held the Symposium on Statistical Inference, a two-day gathering that laid the foundations for this special issue of The American Statistician. Authors were explicitly instructed to develop papers for the variety of audiences interested in these topics. If you use statistics in research, business, or policymaking but are not a statistician, these articles were indeed written with YOU in mind. And if you are a statistician, there is still much here for you as well.
The papers in this issue propose many new ideas, ideas that in our determination as editors merited publication to enable broader consideration and debate. The ideas in this editorial are likewise open to debate. They are our own attempt to distill the wisdom of the many voices in this issue into an essence of good statistical practice as we currently see it: some do’s for teaching, doing research, and informing decisions.
Yet the voices in the 43 papers in this issue do not sing as one. At times in this editorial and the papers you’ll hear deep dissonance, the echoes of “statistics wars” still simmering today (Mayo 2018). At other times you’ll hear melodies wrapping in a rich counterpoint that may herald an increasingly harmonious new era of statistics. To us, these are all the sounds of statistical inference in the 21st century, the sounds of a world learning to venture beyond “p < 0.05.”
This is a world where researchers are free to treat “p = 0.051” and “p = 0.049” as not being categorically different, where authors no longer find themselves constrained to selectively publish their results based on a single magic number. In this world, where studies with “p < 0.05” and studies with “p > 0.05” are not automatically in conflict, researchers will see their results more easily replicated—and, even when not, they will better understand why. As we venture down this path, we will begin to see fewer false alarms, fewer overlooked discoveries, and the development of more customized statistical strategies. Researchers will be free to communicate all their findings in all their glorious uncertainty, knowing their work is to be judged by the quality and effective communication of their science, and not by their p-values. As “statistical significance” is used less, statistical thinking will be used more.
The ASA Statement on P-Values and Statistical Significance started moving us toward this world. As of the date of publication of this special issue, the statement has been viewed over 294,000 times and cited over 1700 times—an average of about 11 citations per week since its release. Now we must go further. That’s what this special issue of The American Statistician sets out to do.
To get to the do’s, though, we must begin with one more don’t.
2 Don’t Say “Statistically Significant”
The ASA Statement on P-Values and Statistical Significance stopped just short of recommending that declarations of “statistical significance” be abandoned. We take that step here. We conclude, based on our review of the articles in this special issue and the broader literature, that it is time to stop using the term “statistically significant” entirely. Nor should variants such as “significantly different,” “p < 0.05,” and “nonsignificant” survive, whether expressed in words, by asterisks in a table, or in some other way.
Regardless of whether it was ever useful, a declaration of “statistical significance” has today become meaningless. Made broadly known by Fisher’s use of the phrase (1925), Edgeworth’s (1885) original intention for statistical significance was simply as a tool to indicate when a result warrants further scrutiny. But that idea has been irretrievably lost. Statistical significance was never meant to imply scientific importance, and the confusion of the two was decried soon after its widespread use (Boring 1919). Yet a full century later the confusion persists.
And so the tool has become the tyrant. The problem is not simply use of the word “significant,” although the statistical and ordinary language meanings of the word are indeed now hopelessly confused (Ghose 2013); the term should be avoided for that reason alone. The problem is a larger one, however: using bright-line rules for justifying scientific claims or conclusions can lead to erroneous beliefs and poor decision making (ASA statement, Principle 3). A label of statistical significance adds nothing to what is already conveyed by the value of p; in fact, this dichotomization of p-values makes matters worse.
For example, no p-value can reveal the plausibility, presence, truth, or importance of an association or effect. Therefore, a label of statistical significance does not mean or imply that an association or effect is highly probable, real, true, or important. Nor does a label of statistical nonsignificance lead to the association or effect being improbable, absent, false, or unimportant. Yet the dichotomization into “significant” and “not significant” is taken as an imprimatur of authority on these characteristics. In a world without bright lines, on the other hand, it becomes untenable to assert dramatic differences in interpretation from inconsequential differences in estimates. As Gelman and Stern (2006) famously observed, the difference between “significant” and “not significant” is not itself statistically significant.
Furthermore, this false split into “worthy” and “unworthy” results leads to the selective reporting and publishing of results based on their statistical significance—the so-called “file drawer problem” (Rosenthal 1979). And the dichotomized reporting problem extends beyond just publication, notes Amrhein, Trafimow, and Greenland (2019): when authors use p-value thresholds to select which findings to discuss in their papers, “their conclusions and what is reported in subsequent news and reviews will be biased…Such selective attention based on study outcomes will therefore not only distort the literature but will slant published descriptions of study results—biasing the summary descriptions reported to practicing professionals and the general public.” For the integrity of scientific publishing and research dissemination, therefore, whether a p-value passes any arbitrary threshold should not be considered at all when deciding which results to present or highlight.
To be clear, the problem is not that of having only two labels. Results should not be trichotomized, or indeed categorized into any number of groups, based on arbitrary p-value thresholds. Similarly, we need to stop using confidence intervals as another means of dichotomizing (based, on whether a null value falls within the interval). And, to preclude a reappearance of this problem elsewhere, we must not begin arbitrarily categorizing other statistical measures (such as Bayes factors).
Despite the limitations of p-values (as noted in Principles 5 and 6 of the ASA statement), however, we are not recommending that the calculation and use of continuous p-values be discontinued. Where p-values are used, they should be reported as continuous quantities (e.g., p = 0.08). They should also be described in language stating what the value means in the scientific context. We believe that a reasonable prerequisite for reporting any p-value is the ability to interpret it appropriately. We say more about this in Section 3.3.
To move forward to a world beyond “p < 0.05,” we must recognize afresh that statistical inference is not—and never has been—equivalent to scientific inference (Hubbard, Haig, and Parsa 2019; Ziliak 2019). However, looking to statistical significance for a marker of scientific observations’ credibility has created a guise of equivalency. Moving beyond “statistical significance” opens researchers to the real significance of statistics, which is “the science of learning from data, and of measuring, controlling, and communicating uncertainty” (Davidian and Louis 2012).
In sum, “statistically significant”—don’t say it and don’t use it.
3 There Are Many Do’s
With the don’ts out of the way, we can finally discuss ideas for specific, positive, constructive actions. We have a massive list of them in the seventh section of this editorial! In that section, the authors of all the articles in this special issue each provide their own short set of do’s. Those lists, and the rest of this editorial, will help you navigate the substantial collection of articles that follows.
Because of the size of this collection, we take the liberty here of distilling our readings of the articles into a summary of what can be done to move beyond “p < 0.05.” You will find the rich details in the articles themselves.
What you will NOT find in this issue is one solution that majestically replaces the outsized role that statistical significance has come to play. The statistical community has not yet converged on a simple paradigm for the use of statistical inference in scientific research—and in fact it may never do so. A one-size-fits-all approach to statistical inference is an inappropriate expectation, even after the dust settles from our current remodeling of statistical practice (Tong 2019). Yet solid principles for the use of statistics do exist, and they are well explained in this special issue.
We summarize our recommendations in two sentences totaling seven words: “Accept uncertainty. Be thoughtful, open, and modest.” Remember “ATOM.”
3.1 Accept Uncertainty
Uncertainty exists everywhere in research. And, just like with the frigid weather in a Wisconsin winter, there are those who will flee from it, trying to hide in warmer havens elsewhere. Others, however, accept and even delight in the omnipresent cold; these are the ones who buy the right gear and bravely take full advantage of all the wonders of a challenging climate. Significance tests and dichotomized p-values have turned many researchers into scientific snowbirds, trying to avoid dealing with uncertainty by escaping to a “happy place” where results are either statistically significant or not. In the real world, data provide a noisy signal. Variation, one of the causes of uncertainty, is everywhere. Exact replication is difficult to achieve. So it is time to get the right (statistical) gear and “move toward a greater acceptance of uncertainty and embracing of variation” (Gelman 2016).
Statistical methods do not rid data of their uncertainty. “Statistics,” Gelman (2016) says, “is often sold as a sort of alchemy that transmutes randomness into certainty, an ‘uncertainty laundering’ that begins with data and concludes with success as measured by statistical significance.” To accept uncertainty requires that we “treat statistical results as being much more incomplete and uncertain than is currently the norm” (Amrhein, Trafimow, and Greenland 2019). We must “countenance uncertainty in all statistical conclusions, seeking ways to quantify, visualize, and interpret the potential for error” (Calin-Jageman and Cumming 2019).
“Accept uncertainty and embrace variation in effects,” advise McShane et al. in Section 7 of this editorial. “[W]e can learn much (indeed, more) about the world by forsaking the false promise of certainty offered by dichotomous declarations of truth or falsity—binary statements about there being ‘an effect’ or ‘no effect’—based on some p-value or other statistical threshold being attained.”
We can make acceptance of uncertainty more natural to our thinking by accompanying every point estimate in our research with a measure of its uncertainty such as a standard error or interval estimate. Reporting and interpreting point and interval estimates should be routine. However, simplistic use of confidence intervals as a measurement of uncertainty leads to the same bad outcomes as use of statistical significance (especially, a focus on whether such intervals include or exclude the “null hypothesis value”). Instead, Greenland (2019) and Amrhein, Trafimow, and Greenland (2019) encourage thinking of confidence intervals as “compatibility intervals,” which use p-values to show the effect sizes that are most compatible with the data under the given model.
How will accepting uncertainty change anything? To begin, it will prompt us to seek better measures, more sensitive designs, and larger samples, all of which increase the rigor of research. It also helps us be modest (the fourth of our four principles, on which we will expand in Section 3.4) and encourages “meta-analytic thinking” (Cumming 2014). Accepting uncertainty as inevitable is a natural antidote to the seductive certainty falsely promised by statistical significance. With this new outlook, we will naturally seek out replications and the integration of evidence through meta-analyses, which usually requires point and interval estimates from contributing studies. This will in turn give us more precise overall estimates for our effects and associations. And this is what will lead to the best research-based guidance for practical decisions.
Accepting uncertainty leads us to be thoughtful, the second of our four principles.
3.2 Be Thoughtful
What do we mean by this exhortation to “be thoughtful”? Researchers already clearly put much thought into their work. We are not accusing anyone of laziness. Rather, we are envisioning a sort of “statistical thoughtfulness.” In this perspective, statistically thoughtful researchers begin above all else with clearly expressed objectives. They recognize when they are doing exploratory studies and when they are doing more rigidly pre-planned studies. They invest in producing solid data. They consider not one but a multitude of data analysis techniques. And they think about so much more.
3.2.1 Thoughtfulness in the Big Picture
“[M]ost scientific research is exploratory in nature,” Tong (2019) contends. “[T]he design, conduct, and analysis of a study are necessarily flexible, and must be open to the discovery of unexpected patterns that prompt new questions and hypotheses. In this context, statistical modeling can be exceedingly useful for elucidating patterns in the data, and researcher degrees of freedom can be helpful and even essential, though they still carry the risk of overfitting. The price of allowing this flexibility is that the validity of any resulting statistical inferences is undermined.”
Calin-Jageman and Cumming (2019) caution that “in practice the dividing line between planned and exploratory research can be difficult to maintain. Indeed, exploratory findings have a slippery way of ‘transforming’ into planned findings as the research process progresses.” At the bottom of that slippery slope one often finds results that don’t reproduce.
Anderson (2019) proposes three questions thoughtful researchers asked thoughtful researchers evaluating research results: What are the practical implications of the estimate? How precise is the estimate? And is the model correctly specified? The latter question leads naturally to three more: Are the modeling assumptions understood? Are these assumptions valid? And do the key results hold up when other modeling choices are made? Anderson further notes, “Modeling assumptions (including all the choices from model specification to sample selection and the handling of data issues) should be sufficiently documented so independent parties can critique, and replicate, the work.”
Drawing on archival research done at the Guinness Archives in Dublin, Ziliak (2019) emerges with ten “G-values” he believes we all wish to maximize in research. That is, we want large G-values, not small p-values. The ten principles of Ziliak’s “Guinnessometrics” are derived primarily from his examination of experiments conducted by statistician William Sealy Gosset while working as Head Brewer for Guinness. Gosset took an economic approach to the logic of uncertainty, preferring balanced designs over random ones and estimation of gambles over bright-line “testing.” Take, for example, Ziliak’s G-value 10: “Consider purpose of the inquiry, and compare with best practice,” in the spirit of what farmers and brewers must do. The purpose is generally NOT to falsify a null hypothesis, says Ziliak. Ask what is at stake, he advises, and determine what magnitudes of change are humanly or scientifically meaningful in context.
Pogrow (2019) offers an approach based on practical benefit rather than statistical or practical significance. This approach is especially useful, he says, for assessing whether interventions in complex organizations (such as hospitals and schools) are effective, and also for increasing the likelihood that the observed benefits will replicate in subsequent research and in clinical practice. In this approach, “practical benefit” recognizes that reliance on small effect sizes can be as problematic as relying on p-values.
Thoughtful research prioritizes sound data production by putting energy into the careful planning, design, and execution of the study (Tong 2019).
Locascio (2019) urges researchers to be prepared for a new publishing model that evaluates their research based on the importance of the questions being asked and the methods used to answer them, rather than the outcomes obtained.
3.2.2 Thoughtfulness Through Context and Prior Knowledge
Thoughtful research considers the scientific context and prior evidence. In this regard, a declaration of statistical significance is the antithesis of thoughtfulness: it says nothing about practical importance, and it ignores what previous studies have contributed to our knowledge.
Thoughtful research looks ahead to prospective outcomes in the context of theory and previous research. Researchers would do well to ask, What do we already know, and how certain are we in what we know? And building on that and on the field’s theory, what magnitudes of differences, odds ratios, or other effect sizes are practically important? These questions would naturally lead a researcher, for example, to use existing evidence from a literature review to identify specifically the findings that would be practically important for the key outcomes under study.
Thoughtful research includes careful consideration of the definition of a meaningful effect size. As a researcher you should communicate this up front, before data are collected and analyzed. Afterwards is just too late; it is dangerously easy to justify observed results after the fact and to overinterpret trivial effect sizes as being meaningful. Many authors in this special issue argue that consideration of the effect size and its “scientific meaningfulness” is essential for reliable inference (e.g., Blume et al. 2019; Betensky 2019). This concern is also addressed in the literature on equivalence testing (Wellek 2017).
Thoughtful research considers “related prior evidence, plausibility of mechanism, study design and data quality, real world costs and benefits, novelty of finding, and other factors that vary by research domain…without giving priority to p-values or other purely statistical measures” (McShane et al. 2019).
Thoughtful researchers “use a toolbox of statistical techniques, employ good judgment, and keep an eye on developments in statistical and data science,” conclude Heck and Krueger ((2019)), who demonstrate how the p-value can be useful to researchers as a heuristic.
3.2.3 Thoughtful Alternatives and Complements to P-Values
Thoughtful research considers multiple approaches for solving problems. This special issue includes some ideas for supplementing or replacing p-values. Here is a short summary of some of them, with a few technical details:
Amrhein, Trafimow, and Greenland (2019) and Greenland (2019) advise that null p-values should be supplemented with a p-value from a test of a pre-specified alternative (such as a minimal important effect size). To reduce confusion with posterior probabilities and better portray evidential value, they further advise that p-values be transformed into s-values (Shannon information, surprisal, or binary logworth) s = – log2(p). This measure of evidence affirms other arguments that the evidence against a hypothesis contained in the p-value is not nearly as strong as is believed by many researchers. The change of scale also moves users away from probability misinterpretations of the p-value.
Blume et al. (2019) offer a “second generation p-value (SGPV),” the characteristics of which mimic or improve upon those of p-values but take practical significance into account. The null hypothesis from which an SGPV is computed is a composite hypothesis representing a range of differences that would be practically or scientifically inconsequential, as in equivalence testing (Wellek 2017). This range is determined in advance by the experimenters. When the SGPV is 1, the data only support null hypotheses; when the SGPV is 0, the data are incompatible with any of the null hypotheses. SGPVs between 0 and 1 are inconclusive at varying levels (maximally inconclusive at or near SGPV = 0.5.) Blume et al. illustrate how the SGPV provides a straightforward and useful descriptive summary of the data. They argue that it eliminates the problem of how classical statistical significance does not imply scientific relevance, it lowers false discovery rates, and its conclusions are more likely to reproduce in subsequent studies.
The “analysis of credibility”(AnCred) is promoted by Matthews (2019). This approach takes account of both the width of the confidence interval and the location of its bounds when assessing weight of evidence. AnCred assesses the credibility of inferences based on the confidence interval by determining the level of prior evidence needed for a new finding to provide credible evidence for a nonzero effect. If this required level of prior evidence is supported by current knowledge and insight, Matthews calls the new result “credible evidence for a non-zero effect,” irrespective of its statistical significance/nonsignificance.
Colquhoun (2019) proposes continuing the use of continuous p-values, but only in conjunction with the “false positive risk (FPR).” The FPR answers the question, “If you observe a ‘significant’ p-value after doing a single unbiased experiment, what is the probability that your result is a false positive?” It tells you what most people mistakenly still think the p-value does, Colquhoun says. The problem, however, is that to calculate the FPR you need to specify the prior probability that an effect is real, and it’s rare to know this. Colquhoun suggests that the FPR could be calculated with a prior probability of 0.5, the largest value reasonable to assume in the absence of hard prior data. The FPR found this way is in a sense the minimum false positive risk (mFPR); less plausible hypotheses (prior probabilities below 0.5) would give even bigger FPRs, Colquhoun says, but the mFPR would be a big improvement on reporting a p-value alone. He points out that p-values near 0.05 are, under a variety of assumptions, associated with minimum false positive risks of 20–30%, which should stop a researcher from making too big a claim about the “statistical significance” of such a result.
Benjamin and Berger (2019) propose a different supplement to the null p-value. The Bayes factor bound (BFB)—which under typically plausible assumptions is the value 1/(-ep ln p)—represents the upper bound of the ratio of data-based odds of the alternative hypothesis to the null hypothesis. Benjamin and Berger advise that the BFB should be reported along with the continuous p-value. This is an incomplete step toward revising practice, they argue, but one that at least confronts the researcher with the maximum possible odds that the alternative hypothesis is true—which is what researchers often think they are getting with a p-value. The BFB, like the FPR, often clarifies that the evidence against the null hypothesis contained in the p-value is not nearly as strong as is believed by many researchers.
Goodman, Spruill, and Komaroff (2019) propose a two-stage approach to inference, requiring both a small p-value below a pre-specified level and a pre-specified sufficiently large effect size before declaring a result “significant.” They argue that this method has improved performance relative to use of dichotomized p-values alone.
Gannon, Pereira, and Polpo (2019) have developed a testing procedure combining frequentist and Bayesian tools to provide a significance level that is a function of sample size.
Manski (2019) and Manski and Tetenov (2019) urge a return to the use of statistical decision theory, which they say has largely been forgotten. Statistical decision theory is not based on p-value thresholds and readily distinguishes between statistical and clinical significance.
Billheimer (2019) suggests abandoning inference about parameters, which are frequently hypothetical quantities used to idealize a problem. Instead, he proposes focusing on the prediction of future observables, and their associated uncertainty, as a means to improving science and decision-making.
3.2.4 Thoughtful Communication of Confidence
Be thoughtful and clear about the level of confidence or credibility that is present in statistical results.
Amrhein, Trafimow, and Greenland (2019) and Greenland (2019) argue that the use of words like “significance” in conjunction with p-values and “confidence” with interval estimates misleads users into overconfident claims. They propose that researchers think of p-values as measuring the compatibility between hypotheses and data, and interpret interval estimates as “compatibility intervals.”
In what may be a controversial proposal, Goodman (2018) suggests requiring “that any researcher making a claim in a study accompany it with their estimate of the chance that the claim is true.” Goodman calls this the confidence index. For example, along with stating “This drug is associated with elevated risk of a heart attack, relative risk (RR) = 2.4, p = 0.03,” Goodman says investigators might add a statement such as “There is an 80% chance that this drug raises the risk, and a 60% chance that the risk is at least doubled.” Goodman acknowledges, “Although simple on paper, requiring a confidence index would entail a profound overhaul of scientific and statistical practice.”
In a similar vein, Hubbard and Carriquiry (2019) urge that researchers prominently display the probability the hypothesis is true or a probability distribution of an effect size, or provide sufficient information for future researchers and policy makers to compute it. The authors further describe why such a probability is necessary for decision making, how it could be estimated by using historical rates of reproduction of findings, and how this same process can be part of continuous “quality control” for science.
Being thoughtful in our approach to research will lead us to be open in our design, conduct, and presentation of it as well.
3.3 Be Open
We envision openness as embracing certain positive practices in the development and presentation of research work.
3.3.1 Openness to Transparency and to the Role of Expert Judgment
First, we repeat oft-repeated advice: Be open to “open science” practices. Calin-Jageman and Cumming (2019), Locascio (2019), and others in this special issue urge adherence to practices such as public pre-registration of methods, transparency and completeness in reporting, shared data and code, and even pre-registered (“results-blind”) review. Completeness in reporting, for example, requires not only describing all analyses performed but also presenting all findings obtained, without regard to statistical significance or any such criterion.
Openness also includes understanding and accepting the role of expert judgment, which enters the practice of statistical inference and decision-making in numerous ways (O’Hagan 2019). “Indeed, there is essentially no aspect of scientific investigation in which judgment is not required,” O’Hagan observes. “Judgment is necessarily subjective, but should be made as carefully, as objectively, and as scientifically as possible.”
Subjectivity is involved in any statistical analysis, Bayesian or frequentist. Gelman and Hennig (2017) observe, “Personal decision making cannot be avoided in statistical data analysis and, for want of approaches to justify such decisions, the pursuit of objectivity degenerates easily to a pursuit to merely appear objective.” One might say that subjectivity is not a problem; it is part of the solution.
Acknowledging this, Brownstein et al. (2019) point out that expert judgment and knowledge are required in all stages of the scientific method. They examine the roles of expert judgment throughout the scientific process, especially regarding the integration of statistical and content expertise. “All researchers, irrespective of their philosophy or practice, use expert judgment in developing models and interpreting results,” say Brownstein et al. “We must accept that there is subjectivity in every stage of scientific inquiry, but objectivity is nevertheless the fundamental goal. Therefore, we should base judgments on evidence and careful reasoning, and seek wherever possible to eliminate potential sources of bias.”
How does one rigorously elicit expert knowledge and judgment in an effective, unbiased, and transparent way? O’Hagan (2019) addresses this, discussing protocols to elicit expert knowledge in an unbiased and as scientifically sound was as possible. It is also important for such elicited knowledge to be examined critically, comparing it to actual study results being an important diagnostic step.
3.3.2 Openness in Communication
Be open in your reporting. Report p-values as continuous, descriptive statistics, as we explain in Section 2. We realize that this leaves researchers without their familiar bright line anchors. Yet if we were to propose a universal template for presenting and interpreting continuous p-values we would violate our own principles! Rather, we believe that the thoughtful use and interpretation of p-values will never adhere to a rigid rulebook, and will instead inevitably vary from study to study. Despite these caveats, we can offer recommendations for sound practices, as described below.
In all instances, regardless of the value taken by p or any other statistic, consider what McShane et al. (2019) call the “currently subordinate factors”—the factors that should no longer be subordinate to “p < 0.05.” These include relevant prior evidence, plausibility of mechanism, study design and data quality, and the real-world costs and benefits that determine what effects are scientifically important. The scientific context of your study matters, they say, and this should guide your interpretation.
When using p-values, remember not only Principle 5 of the ASA statement: “A p-value…does not measure the size of an effect or the importance of a result” but also Principle 6: “By itself, a p-value does not provide a good measure of evidence regarding a model or hypothesis.” Despite these limitations, if you present p-values, do so for more than one hypothesized value of your variable of interest (Fraser 2019; Greenland 2019), such as 0 and at least one plausible, relevant alternative, such as the minimum practically important effect size (which should be determined before analyzing the data).
Betensky (2019) also reminds us to interpret the p-value in the context of sample size and meaningful effect size.
Instead of p, you might consider presenting the s-value (Greenland 2019), which is described in Section 3.2. As noted in Section 3.1, you might present a confidence interval. Sound practices in the interpretation of confidence intervals include (1) discussing both the upper and lower limits and whether they have different practical implications, (2) paying no particular attention to whether the interval includes the null value, and (3) remembering that an interval is itself an estimate subject to error and generally provides only a rough indication of uncertainty given that all of the assumptions used to create it are correct and, thus, for example, does not “rule out” values outside the interval. Amrhein, Trafimow, and Greenland (2019) suggest that interval estimates be interpreted as “compatibility” intervals rather than as “confidence” intervals, showing the values that are most compatible with the data, under the model used to compute the interval. They argue that such an interpretation and the practices outlined here can help guard against overconfidence.
It is worth noting that Tong (2019) disagrees with using p-values as descriptive statistics. “Divorced from the probability claims attached to such quantities (confidence levels, nominal Type I errors, and so on), there is no longer any reason to privilege such quantities over descriptive statistics that more directly characterize the data at hand.” He further states, “Methods with alleged generality, such as the p-value or Bayes factor, should be avoided in favor of discipline- and problem-specific solutions that can be designed to be fit for purpose.”
Failing to be open in reporting leads to publication bias. Ioannidis (2019) notes the high level of selection bias prevalent in biomedical journals. He defines “selection” as “the collection of choices that lead from the planning of a study to the reporting of p-values.” As an illustration of one form of selection bias, Ioannidis compared “the set of p-values reported in the full text of an article with the set of p-values reported in the abstract.” The main finding, he says, “was that p-values chosen for the abstract tended to show greater significance than those reported in the text, and that the gradient was more pronounced in some types of journals and types of designs.” Ioannidis notes, however, that selection bias “can be present regardless of the approach to inference used.” He argues that in the long run, “the only direct protection must come from standards for reproducible research.”
To be open, remember that one study is rarely enough. The words “a groundbreaking new study” might be loved by news writers but must be resisted by researchers. Breaking ground is only the first step in building a house. It will be suitable for habitation only after much more hard work.
Be open by providing sufficient information so that other researchers can execute meaningful alternative analyses. van Dongen et al. (2019) provide an illustrative example of such alternative analyses by different groups attacking the same problem.
Being open goes hand in hand with being modest.
3.4 Be Modest
Researchers of any ilk may rarely advertise their personal modesty. Yet the most successful ones cultivate a practice of being modest throughout their research, by understanding and clearly expressing the limitations of their work.
Being modest requires a reality check (Amrhein, Trafimow, and Greenland 2019). “A core problem,” they observe, “is that both scientists and the public confound statistics with reality. But statistical inference is a thought experiment, describing the predictive performance of models about reality. Of necessity, these models are extremely simplified relative to the complexities of actual study conduct and of the reality being studied. Statistical results must eventually mislead us when they are used and communicated as if they present this complex reality, rather than a model for it. This is not a problem of our statistical methods. It is a problem of interpretation and communication of results.”
Be modest in recognizing there is not a “true statistical model” underlying every problem, which is why it is wise to thoughtfully consider many possible models (Lavine 2019). Rougier (2019) calls on researchers to “recognize that behind every choice of null distribution and test statistic, there lurks a plausible family of alternative hypotheses, which can provide more insight into the null distribution.”p-values, confidence intervals, and other statistical measures are all uncertain. Treating them otherwise is immodest overconfidence.
Remember that statistical tools have their limitations. Rose and McGuire (2019) show how use of stepwise regression in health care settings can lead to policies that are unfair.
Remember also that the amount of evidence for or against a hypothesis provided by p-values near the ubiquitous p < 0.05 threshold (Johnson 2019) is usually much less than you think (Benjamin and Berger 2019; Colquhoun 2019; Greenland 2019).
Be modest about the role of statistical inference in scientific inference. “Scientific inference is a far broader concept than statistical inference,” says Hubbard, Haig, and Parsa (2019). “A major focus of scientific inference can be viewed as the pursuit of significant sameness, meaning replicable and empirically generalizable results among phenomena. Regrettably, the obsession with users of statistical inference to report significant differences in data sets actively thwarts cumulative knowledge development.”
The nexus of openness and modesty is to report everything while at the same time not concluding anything from a single study with unwarranted certainty. Because of the strong desire to inform and be informed, there is a relentless demand to state results with certainty. Again, accept uncertainty and embrace variation in associations and effects, because they are always there, like it or not. Understand that expressions of uncertainty are themselves uncertain. Accept that one study is rarely definitive, so encourage, sponsor, conduct, and publish replication studies. Then, use meta-analysis, evidence reviews, and Bayesian methods to synthesize evidence across studies.
Resist the urge to overreach in the generalizability of claims, Watch out for pressure to embellish the abstract or the press release. If the study’s limitations are expressed in the paper but not in the abstract, they may never be read.
Be modest by encouraging others to reproduce your work. Of course, for it to be reproduced readily, you will necessarily have been thoughtful in conducting the research and open in presenting it.
Hubbard and Carriquiry (see their “do list” in Section 7) suggest encouraging reproduction of research by giving “a byline status for researchers who reproduce studies.” They would like to see digital versions of papers dynamically updated to display “Reproduced by….” below original research authors’ names or “not yet reproduced” until it is reproduced.
Indeed, when it comes to reproducibility, Amrhein, Trafimow, and Greenland (2019) demand that we be modest in our expectations. “An important role for statistics in research is the summary and accumulation of information,” they say. “If replications do not find the same results, this is not necessarily a crisis, but is part of a natural process by which science evolves. The goal of scientific methodology should be to direct this evolution toward ever more accurate descriptions of the world and how it works, not toward ever more publication of inferences, conclusions, or decisions.”
Referring to replication studies in psychology, McShane et al. (2019) recommend that future large-scale replication projects “should follow the ‘one phenomenon, many studies’ approach of the Many Labs project and Registered Replication Reports rather than the ‘many phenomena, one study’ approach of the Open Science Collaboration project. In doing so, they should systematically vary method factors across the laboratories involved in the project.” This approach helps achieve the goals of Amrhein, Trafimow, and Greenland (2019) by increasing understanding of why and when results replicate or fail to do so, yielding more accurate descriptions of the world and how it works. It also speaks to significant sameness versus significant difference a la Hubbard, Haig, and Parsa (2019).
Kennedy-Shaffer’s (2019) historical perspective on statistical significance reminds us to be modest, by prompting us to recall how the current state of affairs in p-values has come to be.
Finally, be modest by recognizing that different readers may have very different stakes on the results of your analysis, which means you should try to take the role of a neutral judge rather than an advocate for any hypothesis. This can be done, for example, by pairing every null p-value with a p-value testing an equally reasonable alternative, and by discussing the endpoints of every interval estimate (not only whether it contains the null).
Accept that both scientific inference and statistical inference are hard, and understand that no knowledge will be efficiently advanced using simplistic, mechanical rules and procedures. Accept also that pure objectivity is an unattainable goal—no matter how laudable—and that both subjectivity and expert judgment are intrinsic to the conduct of science and statistics. Accept that there will always be uncertainty, and be thoughtful, open, and modest. ATOM.
And to push this acronym further, we argue in the next section that institutional change is needed, so we put forward that change is needed at the ATOMIC level. Let’s go.
4 Editorial, Educational and Other Institutional Practices Will Have to Change
Institutional reform is necessary for moving beyond statistical significance in any context—whether journals, education, academic incentive systems, or others. Several papers in this special issue focus on reform.
Goodman (2019) notes considerable social change is needed in academic institutions, in journals, and among funding and regulatory agencies. He suggests (see Section 7) partnering “with science reform movements and reformers within disciplines, journals, funding agencies and regulators to promote and reward ‘reproducible’ science and diminish the impact of statistical significance on publication, funding and promotion.” Similarly, Colquhoun (2019) says, “In the end, the only way to solve the problem of reproducibility is to do more replication and to reduce the incentives that are imposed on scientists to produce unreliable work. The publish-or-perish culture has damaged science, as has the judgment of their work by silly metrics.”
Trafimow (2019), who added energy to the discussion of p-values a few years ago by banning them from the journal he edits (Fricker et al. 2019), suggests five “nonobvious changes” to editorial practice. These suggestions, which demand reevaluating traditional practices in editorial policy, will not be trivial to implement but would result in massive change in some journals.
Locascio (2017, 2019) suggests that evaluation of manu-scripts for publication should be “results-blind.” That is, manuscripts should be assessed for suitability for publication based on the substantive importance of the research without regard to their reported results. Kmetz (2019) supports this approach as well and says that it would be a huge benefit for reviewers, “freeing [them] from their often thankless present jobs and instead allowing them to review research designs for their potential to provide useful knowledge.” (See also “registered reports” from the Center for Open Science (https://cos.io/rr/?_ga=2.184185454.979594832.1547755516-1193527346.1457026171) and “registered replication reports” from the Association for Psychological Science (https://www.psychologicalscience.org/publications/replication) in relation to this concept.)
Amrhein, Trafimow, and Greenland (2019) ask if results-blind publishing means that anything goes, and then answer affirmatively: “Everything should be published in some form if whatever we measured made sense before we obtained the data because it was connected in a potentially useful way to some research question.” Journal editors, they say, “should be proud about [their] exhaustive methods sections” and base their decisions about the suitability of a study for publication “on the quality of its materials and methods rather than on results and conclusions; the quality of the presentation of the latter is only judged after it is determined that the study is valuable based on its materials and methods.”
A “variation on this theme is pre-registered replication, where a replication study, rather than the original study, is subject to strict pre-registration (e.g., Gelman 2015),” says Tong (2019). “A broader vision of this idea (Mogil and Macleod 2017) is to carry out a whole series of exploratory experiments without any formal statistical inference, and summarize the results by descriptive statistics (including graphics) or even just disclosure of the raw data. When results from this series of experiments converge to a single working hypothesis, it can then be subjected to a pre-registered, randomized and blinded, appropriately powered confirmatory experiment, carried out by another laboratory, in which valid statistical inference may be made.”
Hurlbert, Levine, and Utts (2019) urge abandoning the use of “statistically significant” in all its forms and encourage journals to provide instructions to authors along these lines: “There is now wide agreement among many statisticians who have studied the issue that for reporting of statistical tests yielding p-values it is illogical and inappropriate to dichotomize the p-scale and describe results as ‘significant’ and ‘nonsignificant.’ Authors are strongly discouraged from continuing this never justified practice that originated from confusions in the early history of modern statistics.”
Hurlbert, Levine, and Utts (2019) also urge that the ASA Statement on P–Values and Statistical Significance “be sent to the editor-in-chief of every journal in the natural, behavioral and social sciences for forwarding to their respective editorial boards and stables of manuscript reviewers. That would be a good way to quickly improve statistical understanding and practice.” Kmetz (2019) suggests referring to the ASA statement whenever submitting a paper or revision to any editor, peer reviewer, or prospective reader. Hurlbert et al. encourage a “community grassroots effort” to encourage change in journal procedures.
Campbell and Gustafson (2019) propose a statistical model for evaluating publication policies in terms of weighing novelty of studies (and the likelihood of those studies subsequently being found false) against pre-specified study power. They observe that “no publication policy will be perfect. Science is inherently challenging and we must always be willing to accept that a certain proportion of research is potentially false.”
Statistics education will require major changes at all levels to move to a post “p < 0.05” world. Two papers in this special issue make a specific start in that direction (Maurer et al. 2019; Steel, Liermann, and Guttorp 2019), but we hope that volumes will be written on this topic in other venues. We are excited that, with support from the ASA, the US Conference on Teaching Statistics (USCOTS) will focus its 2019 meeting on teaching inference.
The change that needs to happen demands change to editorial practice, to the teaching of statistics at every level where inference is taught, and to much more. However…
5 It Is Going to Take Work, and It Is Going to Take Time
If it were easy, it would have already been done, because as we have noted, this is nowhere near the first time the alarm has been sounded.
Why is eliminating the use of p-values as a truth arbiter so hard? “The basic explanation is neither philosophical nor scientific, but sociologic; everyone uses them,” says Goodman (2019). “It’s the same reason we can use money. When everyone believes in something’s value, we can use it for real things; money for food, and p-values for knowledge claims, publication, funding, and promotion. It doesn’t matter if the p-value doesn’t mean what people think it means; it becomes valuable because of what it buys.”
Goodman observes that statisticians alone cannot address the problem, and that “any approach involving only statisticians will not succeed.” He calls on statisticians to ally themselves “both with scientists in other fields and with broader based, multidisciplinary scientific reform movements. What statisticians can do within our own discipline is important, but to effectively disseminate or implement virtually any method or policy, we need partners.”
“The loci of influence,” Goodman says, “include journals, scientific lay and professional media (including social media), research funders, healthcare payors, technology assessors, regulators, academic institutions, the private sector, and professional societies. They also can include policy or informational entities like the National Academies…as well as various other science advisory bodies across the government. Increasingly, they are also including non-traditional science reform organizations comprised both of scientists and of the science literate lay public…and a broad base of health or science advocacy groups…”
It is no wonder, then, that the problem has persisted for so long. And persist it has! Hubbard (2019) looked at citation-count data on twenty-five articles and books severely critical of the effect of null hypothesis significance testing (NHST) on good science. Though issues were well known, Hubbard says, this did nothing to stem NHST usage over time.
Greenland (personal communication, January 25, 2019) notes that cognitive biases and perverse incentives to offer firm conclusions where none are warranted can warp the use of any method. “The core human and systemic problems are not addressed by shifting blame to p-values and pushing alternatives as magic cures—especially alternatives that have been subject to little or no comparative evaluation in either classrooms or practice,” Greenland said. “What we need now is to move beyond debating only our methods and their interpretations, to concrete proposals for elimination of systemic problems such as pressure to produce noteworthy findings rather than to produce reliable studies and analyses. Review and provisional acceptance of reports before their results are given to the journal (Locascio 2019) is one way to address that pressure, but more ideas are needed since review of promotions and funding applications cannot be so blinded. The challenges of how to deal with human biases and incentives may be the most difficult we must face.” Supporting this view is McShane and Gal’s (2016, 2017) empirical demonstration of cognitive dichotomization errors among biomedical and social science researchers—and even among statisticians.
Challenges for editors and reviewers are many. Here’s an example: Fricker et al. (2019) observed that when p-values were suspended from the journal Basic and Applied Social Psychology authors tended to overstate conclusions.
With all the challenges, how do we get from here to there, from a “p < 0.05” world to a post “p < 0.05” world?
Matthews (2019) notes that “Any proposal encouraging changes in inferential practice must accept the ubiquity of NHST.…Pragmatism suggests, therefore, that the best hope of achieving a change in practice lies in offering inferential tools that can be used alongside the concepts of NHST, adding value to them while mitigating their most egregious features.”
Benjamin and Berger (2019) propose three practices to help researchers during the transition away from use of statistical significance. “…[O]ur goal is to suggest minimal changes that would require little effort for the scientific community to implement,” they say. “Motivating this goal are our hope that easy (but impactful) changes might be adopted and our worry that more complicated changes could be resisted simply because they are perceived to be too difficult for routine implementation.”
Yet there is also concern that progress will stop after a small step or two. Even some proponents of small steps are clear that those small steps still carry us far short of the destination.
For example, Matthews (2019) says that his proposed methodology “is not a panacea for the inferential ills of the research community.” But that doesn’t make it useless. It may “encourage researchers to move beyond NHST and explore the statistical armamentarium now available to answer the central question of research: what does our study tell us?” he says. It “provides a bridge between the dominant but flawed NHST paradigm and the less familiar but more informative methods of Bayesian estimation.”
Likewise, Benjamin and Berger (2019) observe, “In research communities that are deeply attached to reliance on ‘p < 0.05,’ our recommendations will serve as initial steps away from this attachment. We emphasize that our recommendations are intended merely as initial, temporary steps and that many further steps will need to be taken to reach the ultimate destination: a holistic interpretation of statistical evidence that fully conforms to the principles laid out in the ASA Statement…”
Yet, like the authors of this editorial, not all authors in this special issue support gradual approaches with transitional methods.
Some (e.g., Amrhein, Trafimow, and Greenland 2019; Hurlbert, Levine, and Utts 2019; McShane et al. 2019) prefer to rip off the bandage and abandon use of statistical significance altogether. In short, no more dichotomizing p-values into categories of “significance.” Notably, these authors do not suggest banning the use of p-values, but rather suggest using them descriptively, treating them as continuous, and assessing their weight or import with nuanced thinking, clear language, and full understanding of their properties.
So even when there is agreement on the destination, there is disagreement about what road to take. The questions around reform need consideration and debate. It might turn out that different fields take different roads.
The catalyst for change may well come from those people who fund, use, or depend on scientific research, say Calin-Jageman and Cumming (2019). They believe this change has not yet happened to the desired level because of “the cognitive opacity of the NHST approach: the counter-intuitive p-value (it’s good when it is small), the mysterious null hypothesis (you want it to be false), and the eminently confusable Type I and Type II errors.”
Reviewers of this editorial asked, as some readers of it will, is a p-value threshold ever okay to use? We asked some of the authors of articles in the special issue that question as well. Authors identified four general instances. Some allowed that, while p-value thresholds should not be used for inference, they might still be useful for applications such as industrial quality control, in which a highly automated decision rule is needed and the costs of erroneous decisions can be carefully weighed when specifying the threshold. Other authors suggested that such dichotomized use of p-values was acceptable in model-fitting and variable selection strategies, again as automated tools, this time for sorting through large numbers of potential models or variables. Still others pointed out that p-values with very low thresholds are used in fields such as physics, genomics, and imaging as a filter for massive numbers of tests. The fourth instance can be described as “confirmatory setting[s] where the study design and statistical analysis plan are specified prior to data collection, and then adhered to during and after it” (Tong 2019). Tong argues these are the only proper settings for formal statistical inference. And Wellek (2017) says at present it is essential in these settings. “[B]inary decision making is indispensable in medicine and related fields,” he says. “[A] radical rejection of the classical principles of statistical inference…is of virtually no help as long as no conclusively substantiated alternative can be offered.”
Eliminating the declaration of “statistical significance” based on p < 0.05 or other arbitrary thresholds will be easier in some venues than others. Most journals, if they are willing, could fairly rapidly implement editorial policies to effect these changes. Suggestions for how to do that are in this special issue of The American Statistician. However, regulatory agencies might require longer timelines for making changes. The U.S. Food and Drug Administration (FDA), for example, has long established drug review procedures that involve comparing p-values to significance thresholds for Phase III drug trials. Many factors demand consideration, not the least of which is how to avoid turning every drug decision into a court battle. Goodman (2019) cautions that, even as we seek change, “we must respect the reason why the statistical procedures are there in the first place.” Perhaps the ASA could convene a panel of experts, internal and external to FDA, to provide a workable new paradigm. (See Ruberg et al. 2019, who argue for a Bayesian approach that employs data from other trials as a “prior” for Phase 3 trials.)
Change is needed. Change has been needed for decades. Change has been called for by others for quite a while. So…
6 Why Will Change Finally Happen Now?
In 1991, a confluence of weather events created a monster storm that came to be known as “the perfect storm,” entering popular culture through a book (Junger 1997) and a 2000 movie starring George Clooney. Concerns about reproducible science, falling public confidence in science, and the initial impact of the ASA statement in heightening awareness of long-known problems created a perfect storm, in this case, a good storm of motivation to make lasting change. Indeed, such change was the intent of the ASA statement, and we expect this special issue of TAS will inject enough additional energy to the storm to make its impact widely felt.
We are not alone in this view. “60+ years of incisive criticism has not yet dethroned NHST as the dominant approach to inference in many fields of science,” note Calin-Jageman and Cumming (2019). “Momentum, though, seems to finally be on the side of reform.”
Goodman (2019) agrees: “The initial slow speed of progress should not be discouraging; that is how all broad-based social movements move forward and we should be playing the long game. But the ball is rolling downhill, the current generation is inspired and impatient to carry this forward.”
So, let’s do it. Let’s move beyond “statistically significant,” even if upheaval and disruption are inevitable for the time being. It’s worth it. In a world beyond “p < 0.05,” by breaking free from the bonds of statistical significance, statistics in science and policy will become more significant than ever.
7 Authors’ Suggestions
The editors of this special TAS issue on statistical inference asked all the contact authors to help us summarize the guidance they provided in their papers by providing us a short list of do’s. We asked them to be specific but concise and to be active—start each with a verb. Here is the complete list of the authors’ responses, ordered as the papers appear in this special issue.
7.1 Getting to a Post “p < 0.05” Era
Ioannidis, J., What Have We (Not) Learnt From Millions of Scientific Papers With p-Values?
Goodman, S., Why Is Getting Rid of p–Values So Hard? Musings on Science and Statistics
Hubbard, R., Will the ASA’s Efforts to Improve Statistical Practice be Successful? Some Evidence to the Contrary
This list applies to the ASA and to the professional statistics community more generally.
Kmetz, J., Correcting Corrupt Research: Recommendations for the Profession to Stop Misuse of p–Values
Hubbard, D., and Carriquiry, A., Quality Control for Scientific Research: Addressing Reproducibility, Responsiveness and Relevance
Brownstein, N., Louis, T., O’Hagan, A., and Pendergast, J., The Role of Expert Judgment in Statistical Inference and Evidence-Based Decision-Making
O’Hagan, A., Expert Knowledge Elicitation: Subjective but Scientific
Kennedy-Shaffer, L., Before p < 0.05 to Beyond p < 0.05: Using History to Contextualize p-Values and Significance Testing
Hubbard, R., Haig, B. D., and Parsa, R. A., The Limited Role of Formal Statistical Inference in Scientific Inference
McShane, B., Tackett, J., Böckenholt, U., and Gelman, A., Large Scale Replication Projects in Contemporary Psychological Research
7.2 Interpreting and Using p
Greenland, S., Valid p-Values Behave Exactly as They Should: Some Misleading Criticisms of p-Values and Their Resolution With s-Values
Betensky, R., The p–Value Requires Context, Not a Threshold
Anderson, A., Assessing Statistical Results: Magnitude, Precision and Model Uncertainty
Heck, P., and Krueger, J., Putting the p-Value in Its Place
Johnson, V., Evidence From Marginally Significant t-Statistics
Fraser, D., The p–Value Function and Statistical Inference
Rougier, J., p–Values, Bayes Factors, and Sufficiency
Rose, S., and McGuire, T., Limitations of p–Values and R-Squared for Stepwise Regression Building: A Fairness Demonstration in Health Policy Risk Adjustment
7.3 Supplementing or Replacing p
Blume, J., Greevy, R., Welty, V., Smith, J., and DuPont, W., An Introduction to Second Generation p-Values
Goodman, W., Spruill, S., and Komaroff, E., A Proposed Hybrid Effect Size Plus p-Value Criterion: Empirical Evidence Supporting Its Use
Benjamin, D., and Berger, J., Three Recommendations for Improving the Use of p-Values
Colquhoun, D., The False Positive Risk: A Proposal Concerning What to Do About p–Values
Notes:
There are many ways to calculate the FPR. One, based on a point null and simple alternative can be calculated with the web calculator at http://fpr-calc.ucl.ac.uk/. However other approaches to the calculation of FPR, based on different assumptions, give results that are similar (Table 1 in Colquhoun 2019).
To calculate FPR it is necessary to specify a prior probability and this is rarely known. My recommendation 2 is based on giving the FPR for a prior probability of 0.5. Any higher prior probability of there being a real effect is not justifiable in the absence of hard data. In this sense, the calculated FPR is the minimum that can be expected. More implausible hypotheses would make the problem worse. For example, if the prior probability of there being a real effect were only 0.1, then observation of p = 0.05 would imply a disastrously high FPR = 0.76, and in order to achieve an FPR of 0.05, you’d need to observe p = 0.00045. Others (especially Goodman) have advocated giving likelihood ratios (LRs) in place of p-values. The FPR for a prior of 0.5 is simply 1/(1 + LR), so to give the FPR for a prior of 0.5 is simply a more-easily-comprehensible way of specifying the LR, and so should be acceptable to frequentists and Bayesians.
Matthews, R., Moving Toward the Post p < 0.05 Era via the Analysis of Credibility
Gannon, M., Pereira, C., and Polpo, A., Blending Bayesian and Classical Tools to Define Optimal Sample-Size-Dependent Significance Levels
Pogrow, S., How Effect Size (Practical Significance) Misleads Clinical Practice: The Case for Switching to Practical Benefit to Assess Applied Research Findings
7.4 Adopting More Holistic Approaches
McShane, B., Gal, D., Gelman, A., Robert, C., and Tackett, J., Abandon Statistical Significance
Tong, C., Statistical Inference Enables Bad Science; Statistical Thinking Enables Good Science
Amrhein, V., Trafimow, D., and Greenland, S., Inferential Statistics as Descriptive Statistics: There Is No Replication Crisis If We Don’t Expect Replication
1. Do not dichotomize, but embrace variation.
(a)Report and interpret inferential statistics like the p-value in a continuous fashion; do not use the word “significant.”
(b)Interpret interval estimates as “compatibility intervals,” showing effect sizes most compatible with the data, under the model used to compute the interval; do not focus on whether such intervals include or exclude zero.
(c)Treat inferential statistics as highly unstable local descriptions of relations between models and the obtained data.
(i)Free your “negative results” by allowing them to be potentially positive. Most studies with large p-values or interval estimates that include the null should be considered “positive,” in the sense that they usually leave open the possibility of important effects (e.g., the effect sizes within the interval estimates).
(ii)Free your “positive results” by allowing them to be different. Most studies with small p-values or interval estimates that are not near the null should be considered provisional, because in replication studies the p-values could be large and the interval estimates could show very different effect sizes.
(iii)There is no replication crisis if we don’t expect replication. Honestly reported results must vary from replication to replication because of varying assumption violations and random variation; excessive agreement itself would suggest deeper problems such as failure to publish results in conflict with group expectations.
Calin-Jageman, R., and Cumming, G., The New Statistics for Better Science: Ask How Much, How Uncertain, and What Else Is Known
Ziliak, S., How Large Are Your G-Values? Try Gosset’s Guinnessometrics When a Little “p” Is Not Enough
Billheimer, D., Predictive Inference and Scientific Reproducibility
Manski, C., Treatment Choice With Trial Data: Statistical Decision Theory Should Supplant Hypothesis Testing
Manski, C., and Tetenov, A., Trial Size for Near Optimal Choice between Surveillance and Aggressive Treatment: Reconsidering MSLT-II
Lavine, M., Frequentist, Bayes, or Other?
Ruberg, S., Harrell, F., Gamalo-Siebers, M., LaVange, L., Lee J., Price K., and Peck C., Inference and Decision-Making for 21st Century Drug Development and Approval
van Dongen, N., Wagenmakers, E.J., van Doorn, J., Gronau, Q., van Ravenzwaaij, D., Hoekstra, R., Haucke, M., Lakens, D., Hennig, C., Morey, R., Homer, S., Gelman, A., and Sprenger, J., Multiple Perspectives on Inference for Two Simple Statistical Scenarios
7.5 Reforming Institutions: Changing Publication Policies and Statistical Education
Trafimow, D., Five Nonobvious Changes in Editorial Practice for Editors and Reviewers to Consider When Evaluating Submissions in a Post P < 0.05 Universe
Locascio, J., The Impact of Results Blind Science Publishing on Statistical Consultation and Collaboration
For journal reviewers
For investigators/manuscript authors
Hurlbert, S., Levine, R., and Utts, J., Coup de Grâce for a Tough Old Bull: “Statistically Significant” Expires
Campbell, H., and Gustafson, P., The World of Research Has Gone Berserk: Modeling the Consequences of Requiring “Greater Statistical Stringency” for Scientific Publication
Fricker, R., Burke, K., Han, X., and Woodall, W., Assessing the Statistical Analyses Used in Basic and Applied Social Psychology After Their p-Value Ban
Maurer, K., Hudiburgh, L., Werwinski, L., and Bailer J., Content Audit for p-Value Principles in Introductory Statistics
Steel, A., Liermann, M., and Guttorp, P., Beyond Calculations: A Course in Statistical Thinking
Gratefully,
Ronald L. Wasserstein
American Statistical Association, Alexandria, VA
[email protected]
Allen L. Schirm
Mathematica Policy Research (retired), Washington, DC
[email protected]
Nicole A. Lazar
Department of Statistics, University of Georgia, Athens, GA
[email protected]
Source: Institute for Operations Research and the Management Sciences (INFORMS)
Source: Society for Industrial and Organisational Psychology of South Africa (SIOPSA)
Without the help of a huge team, this special issue would never have happened. The articles herein are about the equivalent of three regular issues of The American Statistician. Thank you to all the authors who submitted papers for this issue. Thank you, authors whose papers were accepted, for enduring our critiques. We hope they made you happier with your finished product. Thank you to a talented, hard-working group of associate editors for handling many papers: Frank Bretz, George Cobb, Doug Hubbard, Ray Hubbard, Michael Lavine, Fan Li, Xihong Lin, Tom Louis, Regina Nuzzo, Jane Pendergast, Annie Qu, Sherri Rose, and Steve Ziliak. Thank you to all who served as reviewers. We definitely couldn’t have done this without you. Thank you, TAS Editor Dan Jeske, for your vision and your willingness to let us create this special issue. Special thanks to Janet Wallace, TAS editorial coordinator, for spectacular work and tons of patience. We also are grateful to ASA Journals Manager Eric Sampson for his leadership, and to our partners, the team at Taylor and Francis, for their commitment to ASA’s publishing efforts. Thank you to all who read and commented on the draft of this editorial. You made it so much better! Regina Nuzzo provided extraordinarily helpful substantive and editorial comments. And thanks most especially to the ASA Board of Directors, for generously and enthusiastically supporting the “p-values project” since its inception in 2014. Thank you for your leadership of our profession and our association.
References to articles in this special issue
Other articles or books referenced
Keep up to date
Register to receive personalised research and resources by email
Registered in England & Wales No. 3099067
5 Howick Place | London | SW1P 1WG
AcceptCookie Policy
It’s time to talk about ditching statistical significance
Looking beyond a much used and abused measure would make science harder, but better.
Fans of The Hitchhiker’s Guide to the Galaxy know that the answer to life, the Universe and everything is 42. The joke, of course, is that truth cannot be revealed by a single number.
And yet this is the job often assigned to P values: a measure of how surprising a result is, given assumptions about an experiment, including that no effect exists. Whether a P value falls above or below an arbitrary threshold demarcating ‘statistical significance’ (such as 0.05) decides whether hypotheses are accepted, papers are published and products are brought to market. But using P values as the sole arbiter of what to accept as truth can also mean that some analyses are biased, some false positives are overhyped and some genuine effects are overlooked.Scientists rise up against statistical significance
Change is in the air. In a Comment in this week’s issue, three statisticians call for scientists to abandon statistical significance. The authors do not call for P values themselves to be ditched as a statistical tool — rather, they want an end to their use as an arbitrary threshold of significance. More than 800 researchers have added their names as signatories. A series of related articles is being published by the American Statistical Association this week (R. L. Wasserstein et al. Am. Stat. https://doi.org/10.1080/00031305.2019.1583913; 2019). “The tool has become the tyrant,” laments one article.
Statistical significance is so deeply integrated into scientific practice and evaluation that extricating it would be painful. Critics will counter that arbitrary gatekeepers are better than unclear ones, and that the more useful argument is over which results should count for (or against) evidence of effect. There are reasonable viewpoints on all sides; Nature is not seeking to change how it considers statistical analysis in evaluation of papers at this time, but we encourage readers to share their views (see go.nature.com/correspondence).
If researchers do discard statistical significance, what should they do instead? They can start by educating themselves about statistical misconceptions. Most important will be the courage to consider uncertainty from multiple angles in every study. Logic, background knowledge and experimental design should be considered alongside P values and similar metrics to reach a conclusion and decide on its certainty.
When working out which methods to use, researchers should also focus as much as possible on actual problems. People who will duel to the death over abstract theories on the best way to use statistics often agree on results when they are presented with concrete scenarios.
Researchers should seek to analyse data in multiple ways to see whether different analyses converge on the same answer. Projects that have crowdsourced analyses of a data set to diverse teams suggest that this approach can work to validate findings and offer new insights.
In short, be sceptical, pick a good question, and try to answer it in many ways. It takes many numbers to get close to the truth.
Nature 567, 283 (2019)
Sign up to Nature Briefing
An essential round-up of science news, opinion and analysis, delivered to your inbox every weekday.Email addressYes! Sign me up to receive the daily Nature Briefing email. I agree my information will be processed in accordance with the Nature and Springer Nature Limited Privacy Policy.Sign up
Nature (Nature) ISSN 1476-4687 (online) ISSN 0028-0836 (print)
Scientists rise up against statistical significance
Valentin Amrhein, Sander Greenland, Blake McShane and more than 800 signatories call for an end to hyped claims and the dismissal of possibly crucial effects.
When was the last time you heard a seminar speaker claim there was ‘no difference’ between two groups because the difference was ‘statistically non-significant’?
If your experience matches ours, there’s a good chance that this happened at the last talk you attended. We hope that at least someone in the audience was perplexed if, as frequently happens, a plot or table showed that there actually was a difference.
How do statistics so often lead scientists to deny differences that those not educated in statistics can plainly see? For several generations, researchers have been warned that a statistically non-significant result does not ‘prove’ the null hypothesis (the hypothesis that there is no difference between groups or no effect of a treatment on some measured outcome)1. Nor do statistically significant results ‘prove’ some other hypothesis. Such misconceptions have famously warped the literature with overstated claims and, less famously, led to claims of conflicts between studies where none exists.
We have some proposals to keep scientists from falling prey to these misconceptions.
Pervasive problem
Let’s be clear about what must stop: we should never conclude there is ‘no difference’ or ‘no association’ just because a P value is larger than a threshold such as 0.05 or, equivalently, because a confidence interval includes zero. Neither should we conclude that two studies conflict because one had a statistically significant result and the other did not. These errors waste research efforts and misinform policy decisions.
For example, consider a series of analyses of unintended effects of anti-inflammatory drugs2. Because their results were statistically non-significant, one set of researchers concluded that exposure to the drugs was “not associated” with new-onset atrial fibrillation (the most common disturbance to heart rhythm) and that the results stood in contrast to those from an earlier study with a statistically significant outcome.
Now, let’s look at the actual data. The researchers describing their statistically non-significant results found a risk ratio of 1.2 (that is, a 20% greater risk in exposed patients relative to unexposed ones). They also found a 95% confidence interval that spanned everything from a trifling risk decrease of 3% to a considerable risk increase of 48% (P = 0.091; our calculation). The researchers from the earlier, statistically significant, study found the exact same risk ratio of 1.2. That study was simply more precise, with an interval spanning from 9% to 33% greater risk (P = 0.0003; our calculation).
It is ludicrous to conclude that the statistically non-significant results showed “no association”, when the interval estimate included serious risk increases; it is equally absurd to claim these results were in contrast with the earlier results showing an identical observed effect. Yet these common practices show how reliance on thresholds of statistical significance can mislead us (see ‘Beware false conclusions’).
These and similar errors are widespread. Surveys of hundreds of articles have found that statistically non-significant results are interpreted as indicating ‘no difference’ or ‘no effect’ in around half (see ‘Wrong interpretations’ and Supplementary Information).
In 2016, the American Statistical Association released a statement in The American Statistician warning against the misuse of statistical significance and P values. The issue also included many commentaries on the subject. This month, a special issue in the same journal attempts to push these reforms further. It presents more than 40 papers on ‘Statistical inference in the 21st century: a world beyond P < 0.05’. The editors introduce the collection with the caution “don’t say ‘statistically significant’”3. Another article4 with dozens of signatories also calls on authors and journal editors to disavow those terms.
We agree, and call for the entire concept of statistical significance to be abandoned.
We are far from alone. When we invited others to read a draft of this comment and sign their names if they concurred with our message, 250 did so within the first 24 hours. A week later, we had more than 800 signatories — all checked for an academic affiliation or other indication of present or past work in a field that depends on statistical modelling (see the list and final count of signatories in the Supplementary Information). These include statisticians, clinical and medical researchers, biologists and psychologists from more than 50 countries and across all continents except Antarctica. One advocate called it a “surgical strike against thoughtless testing of statistical significance” and “an opportunity to register your voice in favour of better scientific practices”.
We are not calling for a ban on P values. Nor are we saying they cannot be used as a decision criterion in certain specialized applications (such as determining whether a manufacturing process meets some quality-control standard). And we are also not advocating for an anything-goes situation, in which weak evidence suddenly becomes credible. Rather, and in line with many others over the decades, we are calling for a stop to the use of P values in the conventional, dichotomous way — to decide whether a result refutes or supports a scientific hypothesis5.
Quit categorizing
The trouble is human and cognitive more than it is statistical: bucketing results into ‘statistically significant’ and ‘statistically non-significant’ makes people think that the items assigned in that way are categorically different6–8. The same problems are likely to arise under any proposed statistical alternative that involves dichotomization, whether frequentist, Bayesian or otherwise.
Unfortunately, the false belief that crossing the threshold of statistical significance is enough to show that a result is ‘real’ has led scientists and journal editors to privilege such results, thereby distorting the literature. Statistically significant estimates are biased upwards in magnitude and potentially to a large degree, whereas statistically non-significant estimates are biased downwards in magnitude. Consequently, any discussion that focuses on estimates chosen for their significance will be biased. On top of this, the rigid focus on statistical significance encourages researchers to choose data and methods that yield statistical significance for some desired (or simply publishable) result, or that yield statistical non-significance for an undesired result, such as potential side effects of drugs — thereby invalidating conclusions.
The pre-registration of studies and a commitment to publish all results of all analyses can do much to mitigate these issues. However, even results from pre-registered studies can be biased by decisions invariably left open in the analysis plan9. This occurs even with the best of intentions.
Again, we are not advocating a ban on P values, confidence intervals or other statistical measures — only that we should not treat them categorically. This includes dichotomization as statistically significant or not, as well as categorization based on other statistical measures such as Bayes factors.
One reason to avoid such ‘dichotomania’ is that all statistics, including P values and confidence intervals, naturally vary from study to study, and often do so to a surprising degree. In fact, random variation alone can easily lead to large disparities in P values, far beyond falling just to either side of the 0.05 threshold. For example, even if researchers could conduct two perfect replication studies of some genuine effect, each with 80% power (chance) of achieving P < 0.05, it would not be very surprising for one to obtain P < 0.01 and the other P > 0.30. Whether a P value is small or large, caution is warranted.
We must learn to embrace uncertainty. One practical way to do so is to rename confidence intervals as ‘compatibility intervals’ and interpret them in a way that avoids overconfidence. Specifically, we recommend that authors describe the practical implications of all values inside the interval, especially the observed effect (or point estimate) and the limits. In doing so, they should remember that all the values between the interval’s limits are reasonably compatible with the data, given the statistical assumptions used to compute the interval7,10. Therefore, singling out one particular value (such as the null value) in the interval as ‘shown’ makes no sense.
We’re frankly sick of seeing such nonsensical ‘proofs of the null’ and claims of non-association in presentations, research articles, reviews and instructional materials. An interval that contains the null value will often also contain non-null values of high practical importance. That said, if you deem all of the values inside the interval to be practically unimportant, you might then be able to say something like ‘our results are most compatible with no important effect’.
When talking about compatibility intervals, bear in mind four things. First, just because the interval gives the values most compatible with the data, given the assumptions, it doesn’t mean values outside it are incompatible; they are just less compatible. In fact, values just outside the interval do not differ substantively from those just inside the interval. It is thus wrong to claim that an interval shows all possible values.
Second, not all values inside are equally compatible with the data, given the assumptions. The point estimate is the most compatible, and values near it are more compatible than those near the limits. This is why we urge authors to discuss the point estimate, even when they have a large P value or a wide interval, as well as discussing the limits of that interval. For example, the authors above could have written: ‘Like a previous study, our results suggest a 20% increase in risk of new-onset atrial fibrillation in patients given the anti-inflammatory drugs. Nonetheless, a risk difference ranging from a 3% decrease, a small negative association, to a 48% increase, a substantial positive association, is also reasonably compatible with our data, given our assumptions.’ Interpreting the point estimate, while acknowledging its uncertainty, will keep you from making false declarations of ‘no difference’, and from making overconfident claims.
Third, like the 0.05 threshold from which it came, the default 95% used to compute intervals is itself an arbitrary convention. It is based on the false idea that there is a 95% chance that the computed interval itself contains the true value, coupled with the vague feeling that this is a basis for a confident decision. A different level can be justified, depending on the application. And, as in the anti-inflammatory-drugs example, interval estimates can perpetuate the problems of statistical significance when the dichotomization they impose is treated as a scientific standard.
Last, and most important of all, be humble: compatibility assessments hinge on the correctness of the statistical assumptions used to compute the interval. In practice, these assumptions are at best subject to considerable uncertainty7,8,10. Make these assumptions as clear as possible and test the ones you can, for example by plotting your data and by fitting alternative models, and then reporting all results.
Whatever the statistics show, it is fine to suggest reasons for your results, but discuss a range of potential explanations, not just favoured ones. Inferences should be scientific, and that goes far beyond the merely statistical. Factors such as background evidence, study design, data quality and understanding of underlying mechanisms are often more important than statistical measures such as P values or intervals.
The objection we hear most against retiring statistical significance is that it is needed to make yes-or-no decisions. But for the choices often required in regulatory, policy and business environments, decisions based on the costs, benefits and likelihoods of all potential consequences always beat those made based solely on statistical significance. Moreover, for decisions about whether to pursue a research idea further, there is no simple connection between a P value and the probable results of subsequent studies.
What will retiring statistical significance look like? We hope that methods sections and data tabulation will be more detailed and nuanced. Authors will emphasize their estimates and the uncertainty in them — for example, by explicitly discussing the lower and upper limits of their intervals. They will not rely on significance tests. When P values are reported, they will be given with sensible precision (for example, P = 0.021 or P = 0.13) — without adornments such as stars or letters to denote statistical significance and not as binary inequalities (P < 0.05 or P > 0.05). Decisions to interpret or to publish results will not be based on statistical thresholds. People will spend less time with statistical software, and more time thinking.
Our call to retire statistical significance and to use confidence intervals as compatibility intervals is not a panacea. Although it will eliminate many bad practices, it could well introduce new ones. Thus, monitoring the literature for statistical abuses should be an ongoing priority for the scientific community. But eradicating categorization will help to halt overconfident claims, unwarranted declarations of ‘no difference’ and absurd statements about ‘replication failure’ when the results from the original and replication studies are highly compatible. The misuse of statistical significance has done much harm to the scientific community and those who rely on scientific advice. P values, intervals and other statistical measures all have their place, but it’s time for statistical significance to go.
Nature 567, 305-307 (2019)
Sign up to Nature Briefing
An essential round-up of science news, opinion and analysis, delivered to your inbox every weekday.Email addressYes! Sign me up to receive the daily Nature Briefing email. I agree my information will be processed in accordance with the Nature and Springer Nature Limited Privacy Policy.Sign up
Nature (Nature) ISSN 1476-4687 (online) ISSN 0028-0836 (print)