DARPA is betting $2 billion on your next AI innovation
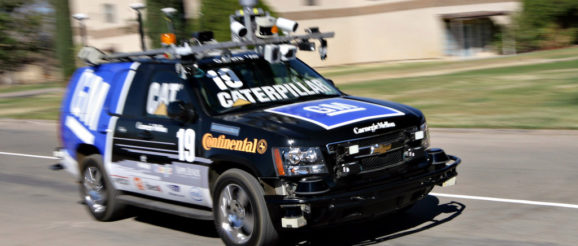
DARPA stands for “Defense Advanced Research Projects Agency,” but while defense is good and all, what DARPA is really into is that P, for projects. The agency is focused on the development of breakthrough technology, and its sights are focused on the enormous potential of artificial intelligence. Its funding for AI projects is huge by any measure, and available to applicants far beyond the traditional defense community. Which could mean you.
As a 60th birthday present for itself, DARPA launched the AI Next campaign this past September, announcing a $2 billion investment applied to AI in a variety areas over a period of five years — or about $400 million a year, says Brian Pierce, Director of the Information Innovation Office at DARPA, and a notable speaker at this year’s VB Summit, October 22-23 in Mill Valley, CA.
Anyone can participate in DARPA-funded programs by responding to an invitation for proposals on fbo.gov. Sometimes the agency is looking for proposals aimed at solving a specific technical goal, and sometimes it simply invites a range of innovative projects, all of which have the potential to be funded out of some very deep pockets.
DARPA-funded initiatives like their previous Grand Challenges and the Urban Challenge, with $2 million dollars in first-place prize money, resulted in leaps in evolution for the autonomous car. Challengers went from limping through an autonomous vehicle race in 2004 — where no one won — to five vehicles finishing the course just a year later. Three years later, those cars were navigating traffic in the Urban Challenge, with a course designed to replicate an urban landscape. Plus, DARPA investment allowed an audio electronics company in the Bay area to develop that low-cost lidar system for sensing in self-driving vehicles.
And DARPA investment also launched what became Siri, developed by SRI in Menlo Park.
The mission of AI Next is to take artificial intelligence into the next wave of its evolution, and trigger a whole new level of innovation, Pierce says.
You might remember the first wave, in which we saw the rise of rule-based systems, the “if this, then do that,” style of AI that’s been applied to things like tax software, shipping logistics, and chess game software. The first wave of AI is essentially pre-programmed by human experts, with no capacity for learning.
Where things really got exciting was the land fall of the second wave, where machine learning, or statistical learning approaches, were born – things like voice recognition technology, from which Siri sprung, and the face recognition we see in cameras today. These second-wave approaches require a huge amount of data to train functions like deep neural networks that, for example, classify images.
However, these functions are still addressing relatively narrow applications. If you go to something outside the training set, confidence or accuracy can drop off significantly. Algorithms that are used today in second-wave AI also tend to be dominated by advances made 20 years ago or so, and second-wave AI applications are prone to being fooled. For instance, the case where Post-Its fooled machine learning algorithms into thinking a stop sign was actually a 45 MPH speed limit sign. AI Next is designed to address these kinds of issues in the second wave, but it’s also looking ahead to the future.
But the emphasis is on the third wave, which is all about broadening the application of AI, or adding what you might call “contextual reasoning,” or even common sense. In situations an AI system has never encountered or been explicitly trained for, can it learn to pick up clues from the environment and come to accurate conclusions? DARPA is betting yes, says Pierce.
With today’s machine learning approaches, an algorithm can identify objects in a scene – a woman on a sofa holding a bowl of popcorn. But where the machine would fail today is in not being able to infer what that person is actually doing in the scene, the way a human might put together smaller, more subtle pieces, connect them to our own experiences, and make an inference about what’s actually happening. You see the blue glow on her face, her rapt attention on something outside of the picture; you figure out, with a high probability of success that she’s probably watching the latest episode of The Good Place on the television and is on Team Elhani.
Or show a computer a picture of a cat on a suitcase. There’s a cat, there’s a suitcase—but the real question is, can the cat fit into the suitcase? That’s beyond the ability of machine learning today, as it lacks the human ability to sense how objects occupy volume in space.
The initiative to address this issue is called Machine Common Sense. But as much as you’re interested in knowing if the suitcase is large enough for the cat, why does it matter? Because if we want machines to work with us, cooperating with us on physical tasks, we’re going to want them to have this common sense reasoning about the physical world, and about people, that we start to acquire from the day we’re born.
Equipping machines with this kind of common sense helps get us closer to making a computer or a machine actually function more as a partner or colleague rather than just as a tool serving the purpose of a human’s tasks or objectives, says Pierce – presumably because making Skynet feel included is the way to keep Skynet from killing us all.
Other programs include Learning with Less Labels, focused on reducing the expense of AI, where billions of data points is cheap, but actually labeling that data is where things gets spendy. Lifelong Learning Machines wants to make machines able to transfer the ability to identify objects in one environment to a whole new environment.
“We feel that if we can make the interactions between humans and machines more symmetric, we can have machines become more effective partners in whatever endeavor we may tackle,” Pierce explains. “It’s the foundation that starts to enable other types of applications.”