Leveraging artificial intelligence for enterprise innovation
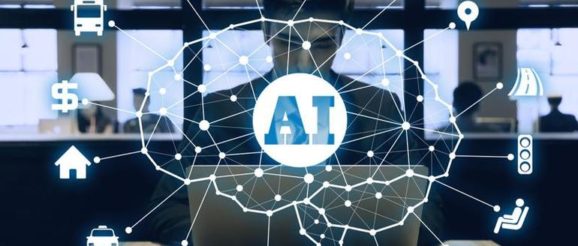
In this age of digital disruption, enterprise innovation is more important than ever before – if not completely necessary for survival.
Artificial intelligence (AI) is one of the most useful new technologies that can be leveraged to help enterprises to generate and build disruptive innovations: new technologies that make more widely accessible valued products and services.
Specifically, new AI technologies could be designed to help enterprise innovators gain new insights into identifying the right kind of problems to be pursued, and in so doing, create new value for each and every individual in an organization, a community, and throughout society.
To this end, I draw on research on data distortion, mental models, and collaboration dynamics to offer three ideas on how AI might be designed to mitigate the inherent biases of human cognition to help innovators and enterprises find and pursue the right problems to solve in search of innovation opportunities.
Problem finding and innovation
In their seminal work on problem finding, Jacob Getzels and Mihaly Csikszentmihalyi [1] posit that creative outcomes originate from those who engage in a continuous process of reformulating their own perspective of the problem at hand.
In other words, creativity is best achieved by continuously reframing a problem to find the right problem to solve. It turns out that finding the right problem to solve is actually quite difficult – in large part because it is not natural. For one, the natural tendency is to jump straight to brainstorming when confronted with a problem.
The natural tendency to brainstorm immediately – rather than engaging in problem finding – reflects a subjective understanding of the problem biased by the limitations of the individual perspective, and therefore inhibits innovation.
To mitigate this inherent bias, enterprises have strategically approached innovation with data, expertise, and collaboration.
Data distortion
First, enterprises collect data to mitigate the bias inherent in problem finding.
Collecting data is based on the premise that the more data that you can collect about your target market or consumer, the deeper your customer insight is likely to be, and there you will be able to find the right [customer] problem to solve.
However, research has shown that we tend to collect information that is consistent with our initial or emerging preferences [2]. That is, our natural tendency is to collect and emphasize data that strengthens or confirms our initial biased understanding of problem, rather than data that provides an alternative understanding of the problem.
To this end, AI can make a significant contribution to innovation: AI can be developed to support non-biased data collection to foster effective problem finding. It might be designed with a Gaussian mixture model algorithm characteristic of unsupervised learning to augment the cognition underpinning data collection for innovation by aiding the innovator to develop deeper (and more accurate) insights about the kind of “jobs” that a customer needs done.
In doing so, the innovator is able to strategically identify and decide which opportunities for innovation to pursue and develop.