Visual Transformers are key to next level of innovation in image related AI applications
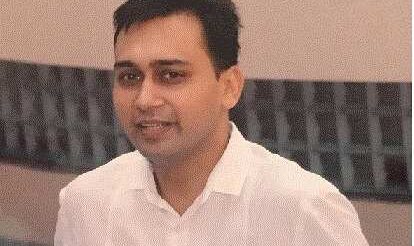
In the field of computer vision, convolutional neural networks (CNNs) have long been the dominant approach to image recognition. However, in recent years, a new type of model has emerged that is challenging CNNs for dominance: visual transformers.
Visual transformers are a type of neural network that is inspired by the transformer architecture, which was originally developed for natural language processing (NLP). Transformers are able to learn long-range dependencies in their input data, which makes them well-suited for tasks such as machine translation and text summarization.
Visual transformers have been shown to be effective for a variety of image recognition tasks, including object detection, image classification, and image segmentation. In some cases, visual transformers have even outperformed CNNs on these tasks.
One of the main advantages of visual transformers is that they are able to learn global features from images. CNNs, on the other hand, are only able to learn local features. This is because CNNs operate on a sliding window over the input image, and they only learn features that are present within the window.
Visual transformers, on the other hand, are able to attend to any part of the input image. This allows them to learn global features that are not limited to a specific region of the image.
Another advantage of visual transformers is that they are more efficient than CNNs. This is because visual transformers do not require any convolution operations. Convolution operations are computationally expensive, and they can be a bottleneck for training deep neural networks.
As a result of these advantages, visual transformers are becoming increasingly popular for image recognition tasks. They are already being used in a variety of commercial applications, and they are likely to become even more widespread in the future.
A Closer Look at Visual Transformers
Visual transformers are a relatively new type of model, so there is still a lot that we do not know about them. However, we do know that they work by learning global features from images. This is done through a process called self-attention.
Self-attention is a mechanism that allows a neural network to attend to any part of its input data. In the case of visual transformers, self-attention is used to attend to any part of the input image. This allows the network to learn global features that are not limited to a specific region of the image.
Self-attention is a powerful mechanism, and it has been shown to be effective for a variety of tasks. In addition to image recognition, self-attention has also been used for tasks such as machine translation, text summarization, and natural language generation.
The Future of Visual Transformers
Visual transformers are a promising new approach to image recognition. They have already shown promising results on a variety of tasks, and they are likely to become even more widespread in the future.
One of the main challenges facing visual transformers is that they are still relatively new. This means that there is a lot of room for improvement. However, the research community is actively working on improving visual transformers, and it is likely that they will continue to improve in the future.
Another challenge facing visual transformers is that they are not as widely adopted as CNNs. This is because CNNs are more established, and they have a larger community of developers and researchers. However, as visual transformers continue to improve, it is likely that they will become more widely adopted.
Overall, visual transformers are a promising new approach to image recognition.
{{{short}}}
{{#more}}
{{{long}}}… Read More
{{/more}}
{{/totalcount}}
{{^totalcount}}